Deep Learning-based Emulator for 6S Atmospheric Correction Model
2021 IEEE International India Geoscience and Remote Sensing Symposium (InGARSS)(2021)
摘要
The quality of high-resolution satellite images is affected by contamination due to atmospheric elements including ozone, CO
2
, and haze, making atmospheric correction a necessary and crucial preprocessing step for many remote sensing applications. Existing models are based on physics-based radiative transfer codes that involve substantial computational costs. They rely on pre-computed lookup tables parameterized by factors that are hard to obtain like air pressure, digital elevation model, aerosol optical depth, and water vapor concentration. Deep Neural networks (DNN) can emulate physics-based models where they learn nonlinear mapping between top-of-the-atmosphere (TOA) reflectance and surface reflectance (SR) from satellite images. DNN need only TOA and SR values to build an effective atmospheric correction model and they depend on combinatorial search instead of complex atmospheric parameters. The paper proposes the first DNN emulator for the 6S physics-based atmospheric correction model. The coefficient of correlation between the model predicted surface reflectance and the 6S physics-based model is 0.9777, 0.9770, 0.9781, and 0.9788 for the Blue, Green, Red, and NIR bands, respectively.
更多查看译文
关键词
emulator,learning-based
AI 理解论文
溯源树
样例
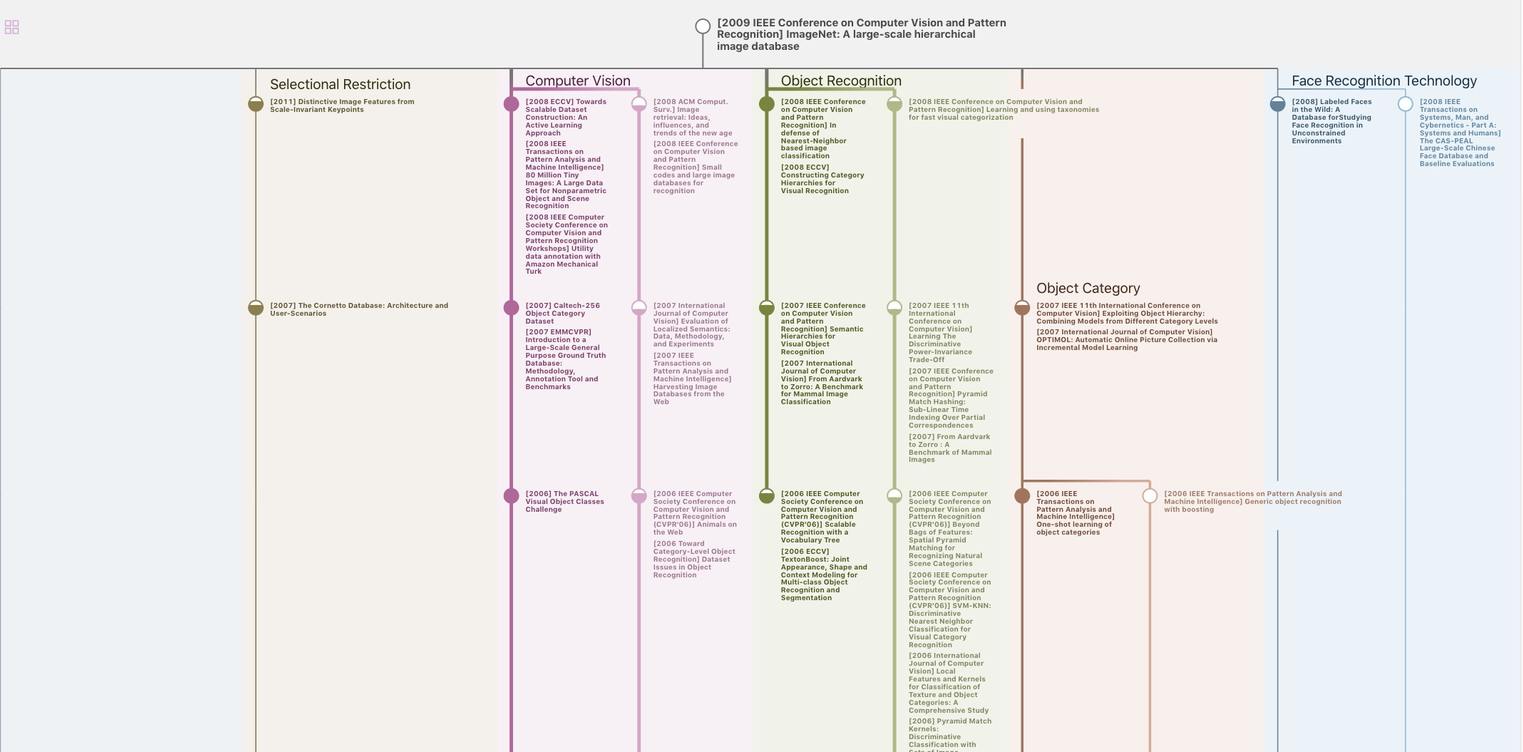
生成溯源树,研究论文发展脉络
Chat Paper
正在生成论文摘要