Adaptive Domain-Invariant Feature Extraction for Cross-Domain Linguistic Steganalysis
IEEE TRANSACTIONS ON INFORMATION FORENSICS AND SECURITY(2024)
摘要
Existing linguistic steganalysis methods require the training and testing datasets to be independent and identically distributed (i.i.d). However, in real-world scenarios, various types of text and steganographic algorithms are employed to generate steganographic text, making it challenging to fulfill the requirement of independent and identical distribution between training and test datasets. This issue, known as the domain mismatch problem, significantly diminishes the detection performance. Thus, it is reasonable to consider domain adaptation by reducing the distribution discrepancy of different domains. However, how to measure and minimize the discrepancy for linguistic steganalysis remains a big challenge. In this paper, we put forward a cross-domain linguistic steganalysis architecture based on a new domain distance metric and adaptive weight selection network. Concretely, a novel steganographic domain distance metric (SDDM) is first proposed, which can effectively characterize the overall distribution discrepancy and capture the weak noise introduced by the information embedding process. Additionally, an adaptive weight selection network with a switching-path structure is designed to calculate domain-specific attention weights, facilitating the model to adapt to various discrepancies scenarios and enhancing its domain-invariant feature representation capability. Extensive experiments show that the proposed method achieves state-of-the-art performance for cross-domain linguistic steganalysis.
更多查看译文
关键词
Feature extraction,Linguistics,Measurement,Testing,Training,Training data,Task analysis,Steganalysis,domain mismatch,domain distance metric
AI 理解论文
溯源树
样例
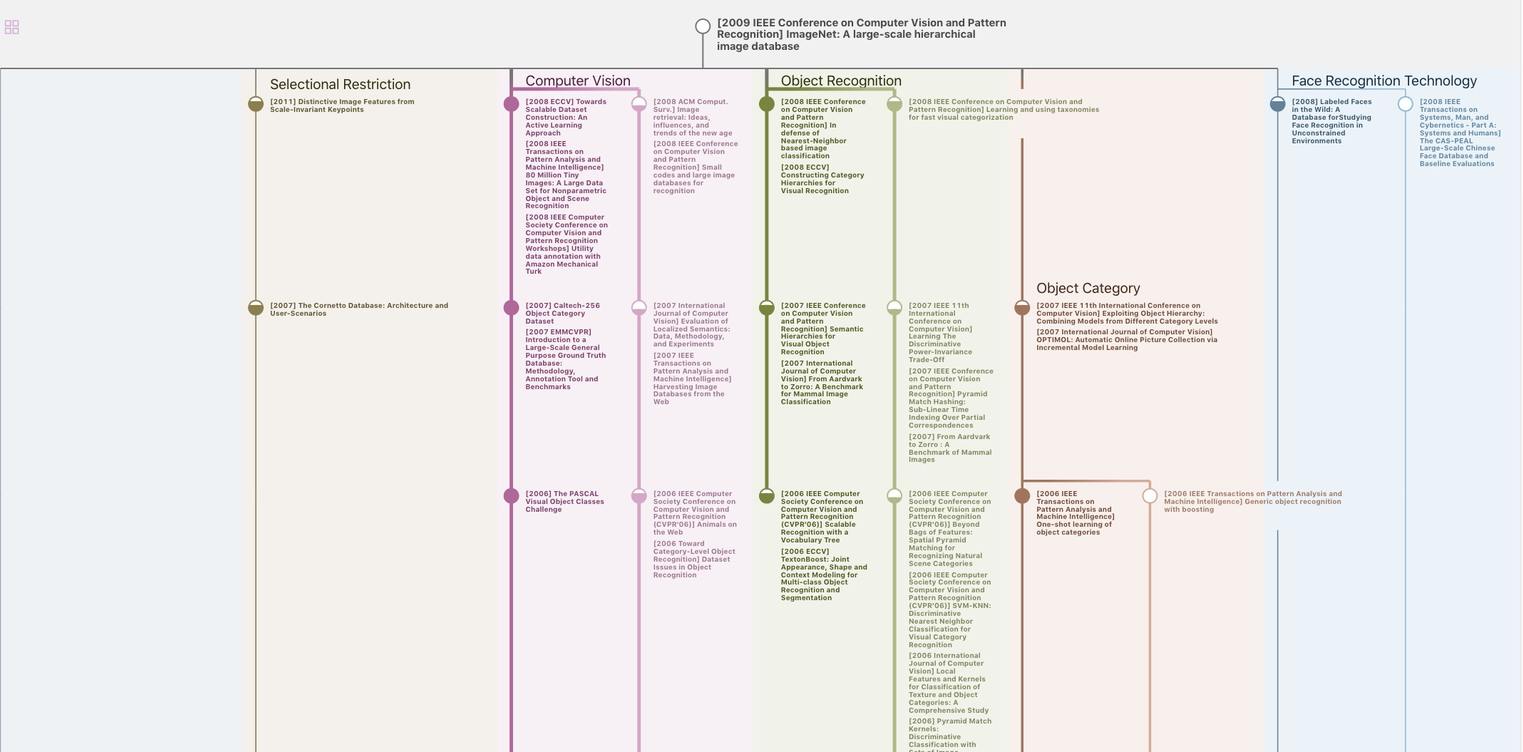
生成溯源树,研究论文发展脉络
Chat Paper
正在生成论文摘要