Quantum Negative Sampling Strategy for Knowledge Graph Embedding with Variational Circuit
2023 IEEE International Conference on Quantum Computing and Engineering (QCE)(2023)
摘要
Knowledge graph is a collection of facts, known as triples(head, relation, tail), which are represented in form of a network, where nodes are entities and edges are relations among the respective head and tail entities. Embedding of knowledge graph for facilitating downstream tasks such as knowledge graph completion, link prediction, recommendation, has been a major area of research recently in classical machine learning. Because the size of knowledge graphs are becoming larger, one of the natural choices is to exploit quantum computing for knowledge graph embedding. Recently, a hybrid quantum classical model for knowledge graph embedding has been studied in which a variational quantum circuit is trained. One of the important aspects in knowledge graph embedding is the sampling of negative triples, which plays a crucial role in efficient training of the model. In classical machine learning various negative sampling strategies have been studied. In quantum knowledge graph embedding model, although we can use these strategies in principle, it is natural to ask if we can exploit quantum advantage in negative sampling. In this article we study such a negative sampling strategy, which exploits quantum superposition, and evaluate the model's performance with a knowledge graph database.
更多查看译文
关键词
Knowledge graph embedding,Link predictions,Hybrid quantum classical model
AI 理解论文
溯源树
样例
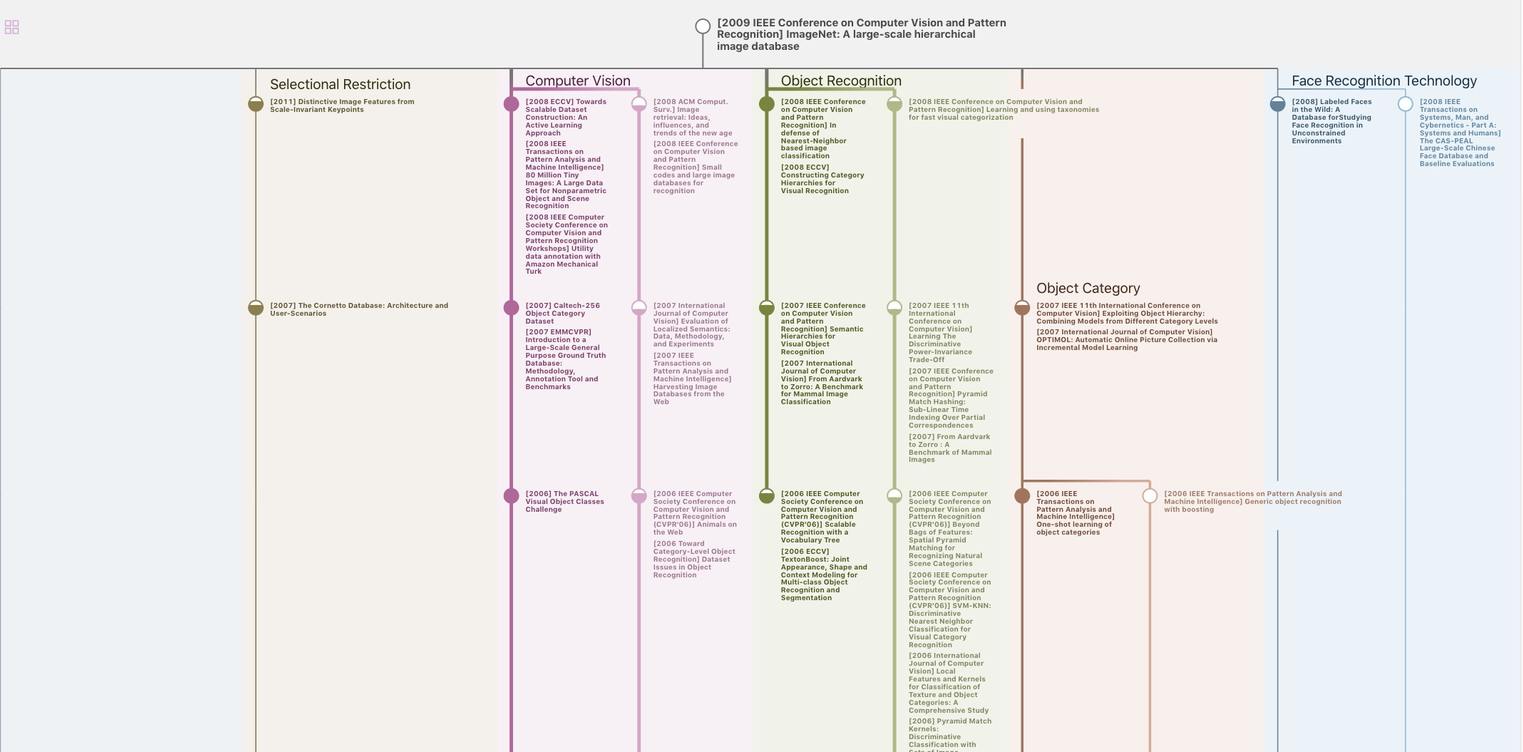
生成溯源树,研究论文发展脉络
Chat Paper
正在生成论文摘要