Machine learning methods for particle stress development in suspension Poiseuille flows
RHEOLOGICA ACTA(2023)
摘要
Numerical simulations are used to study the dynamics of a developing suspension Poiseuille flow with monodispersed and bidispersed neutrally buoyant particles in a planar channel, and machine learning is applied to learn the evolving stresses of the developing suspension. The particle stresses and pressure develop on a slower time scale than the volume fraction, indicating that once the particles reach a steady volume fraction profile, they rearrange to minimize the contact pressure on each particle. We consider the timescale for stress development and how the stress development connects to particle migration. For developing monodisperse suspensions, we present a new physics-informed Galerkin neural network that allows for learning the particle stresses when direct measurements are not possible. We show that when a training set of stress measurements is available, the MOR-physics operator learning method can also capture the particle stresses accurately.
更多查看译文
关键词
Stokes flow,Viscous suspensions,Particle dispersion,Machine learning
AI 理解论文
溯源树
样例
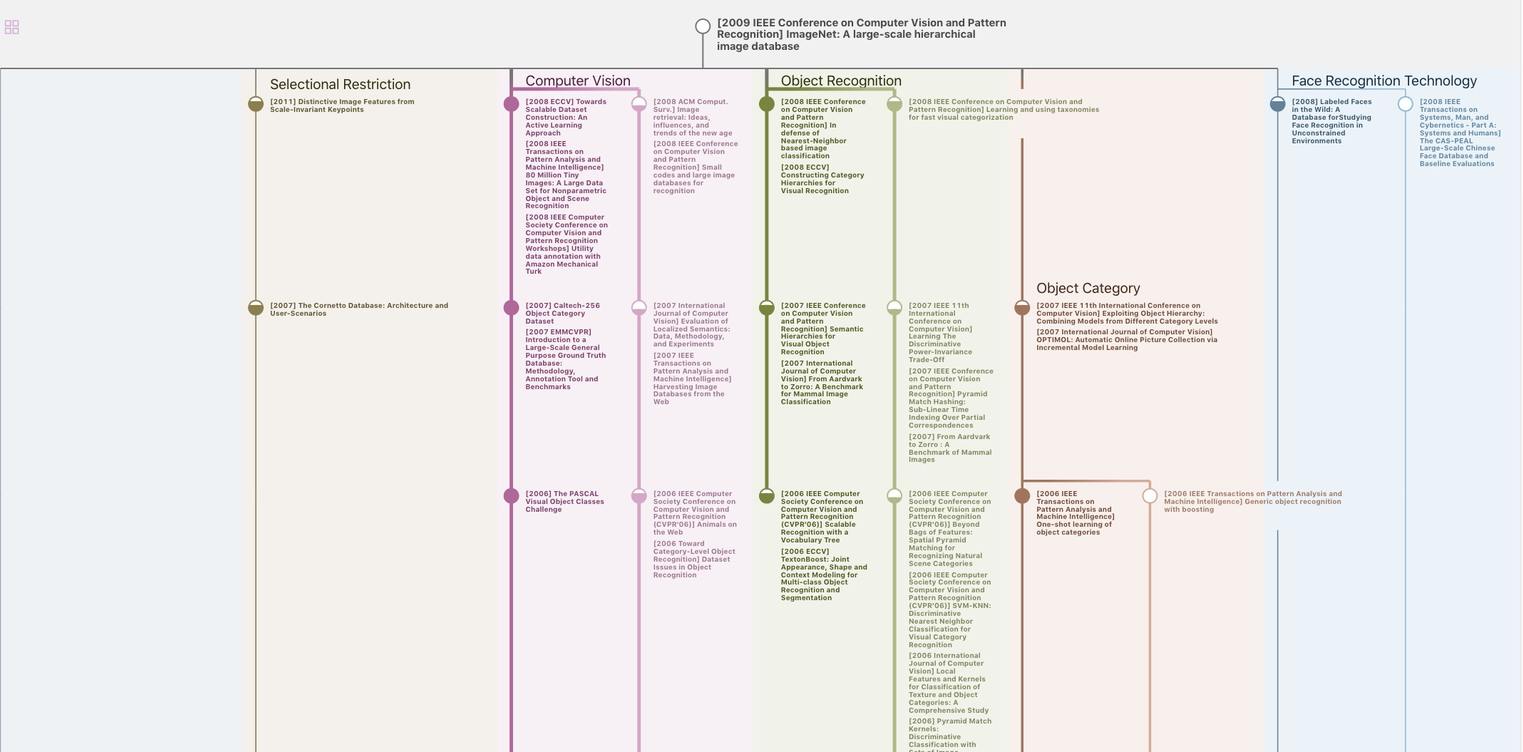
生成溯源树,研究论文发展脉络
Chat Paper
正在生成论文摘要