Predicting Real-time, Recurrent Adverse Invasive Ventilation from Clinical Data Streams
2023 IEEE 19TH INTERNATIONAL CONFERENCE ON BODY SENSOR NETWORKS, BSN(2023)
摘要
Electronic Health Record (EHR) data provide a tremendous opportunity for enhancing the quality of care by delivering personalized treatments [1]. EHR data are rich and tracked at high frequency over time, and can be integrated into a real-time, continuous warning system. One problem that can benefit from real-time monitoring of EHR is the occurrence of invasive-ventilation (iV) for Intensive Care Unit (ICU) patients. The prognostication of a future event falls in the domain of survival analysis. However, iV can occur multiple times during an ICU stay, while most survival analysis tools work only with non-recurring events. Moreover, most survival analysis models are restricted to time-static data, while EHR data changes over time, e.g. heart rate. Therefore, to model the iV problem we turn to BoXHED2.0 [2], a fully nonparametric survival machine learning method that is grounded in theory [3]. Importantly, BoXHED2.0 can handle recurring events data with time-dependent covariates, and we apply it to the MIMIC IV data set [4] to develop a real-time ICU iV warning indicator. Our ICU iV model achieves an AUCPR of 0.34 (versus 0.13 for the benchmarks) and an AUROC of 0.85 (versus 0.73 for the benchmarks) out of sample, speaking to its effectiveness in real-time risk monitoring. Clinical relevance- Creating a real-time risk monitoring system for invasive-ventilation helps reduce its occurrence and/or duration. This would cut down on the complications that the prolonged use of invasive ventilation causes.
更多查看译文
AI 理解论文
溯源树
样例
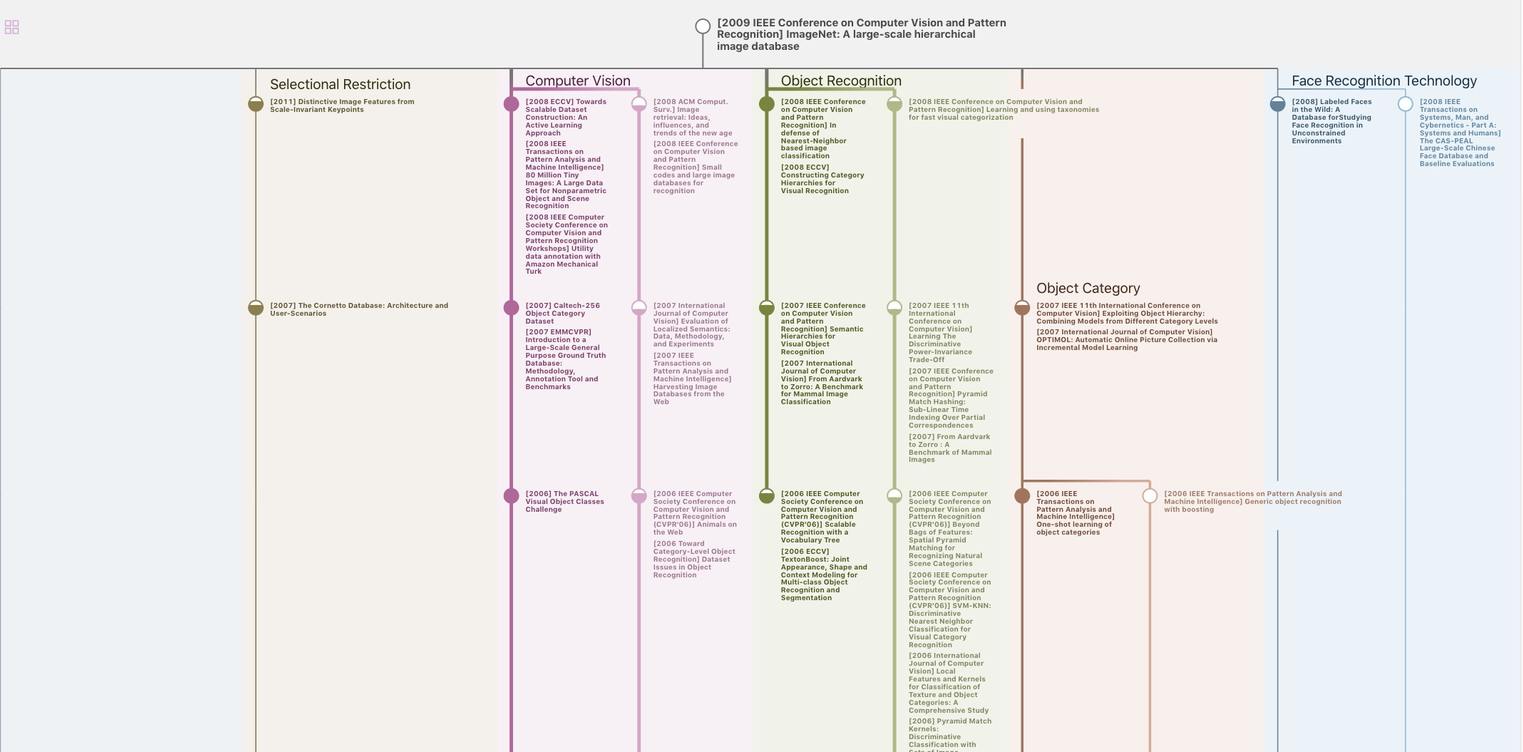
生成溯源树,研究论文发展脉络
Chat Paper
正在生成论文摘要