Evolving Cauchy Possibilistic Clustering for Data Stream Modeling and Classification
2023 IEEE AFRICON(2023)
摘要
This article proposes a novel approach to clustering called eCauchy, which is based on the Cauchy density function. This method is similar to the well-known possibilistic c-means clustering (PCM), which was developed to address the limitations of fuzzy c-means algorithms (FCM). Density-based clustering is particularly useful when dealing with noisy data and frequent outliers. The recursive measure of density can be adapted for various data mining tasks, including regression and classification. The eCauchy algorithm is simple to use and can handle big-data problems. It evolves dynamically by adding and removing clusters based on a few initial parameters, such as maximum and minimum density. This allows for the identification of clusters with different shapes and sizes and is robust to outliers and high levels of noise. The paper demonstrates the universality of this algorithm and its modifications through several simple examples that can also be found in more complex forms in real-world applications.
更多查看译文
关键词
Big-data,Data Stream,Evolving Clustering,Cauchy Density
AI 理解论文
溯源树
样例
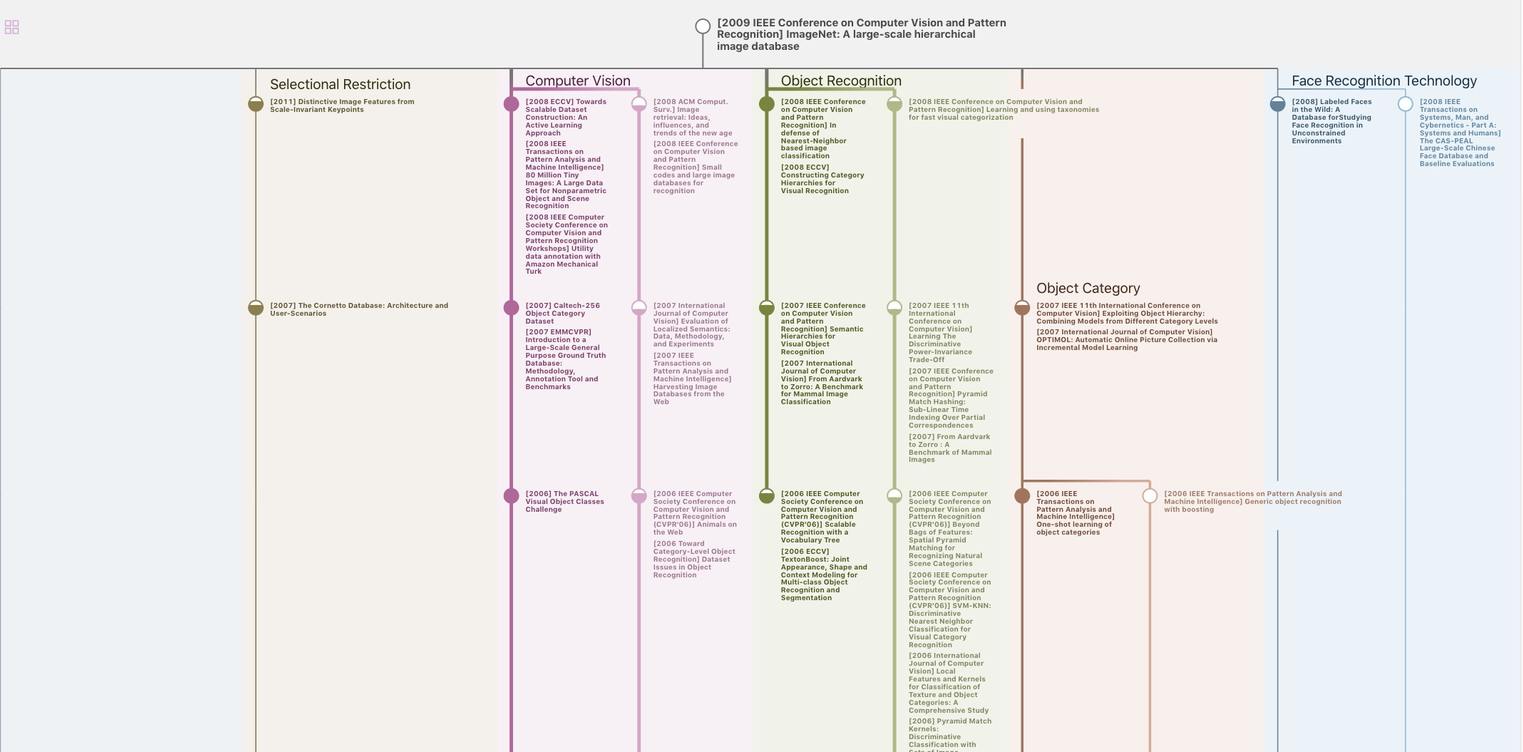
生成溯源树,研究论文发展脉络
Chat Paper
正在生成论文摘要