Graph-based Multivariate Multiscale Permutation Entropy: Study of Robustness to Noise and Application to Two-Phase Flow Data
2023 31st European Signal Processing Conference (EUSIPCO)(2023)
摘要
We propose a novel technique for exploring the complexity of multivariate time series (possibly with different lengths) across multiple time scales using a new graph-based approach. Our method, called multivariate multiscale permutation entropy,
$\text{MMPE}_{\mathrm{G}}$
, incorporates the interactions between channels by constructing an underlying graph for each coarsegrained time series and then applying the recent permutation entropy for graph signals. This approach enables the analysis of multivariate time series with varying lengths, providing insights into the dynamics and relationships between different channels. To address the challenge posed by noise in real-world data analysis, we evaluate the robustness of
$\text{MMPE}_{\mathrm{G}}$
to noise using synthetic time series with varying levels of noise. Our results show that
$\text{MMPE}_{\mathrm{G}}$
exhibits better performance than similar multivariate entropy metrics. We also apply
$\text{MMPE}_{\mathrm{G}}$
to study two-phase flow data, an important industrial process characterised by complex and dynamic behaviour. Specifically, we process multivariate Electrical Resistance Tomography (ERT) data and extract multivariate multiscale permutation entropy values. The results indicate that
$\text{MMPE}_{\mathrm{G}}$
characterises the flow behaviour transition of two-phase flow by incorporating information from different scales and is sensitive to the dynamics of different flow patterns. The noise-robustness of
$\text{MMPE}_{\mathrm{G}}$
makes it a suitable approach for analysing the complexity of multivariate time series and characterising two-phase flow recordings.
更多查看译文
关键词
dispersion entropy,complexity,graph signals,entropy metrics,two-phase flow,multivariate time series
AI 理解论文
溯源树
样例
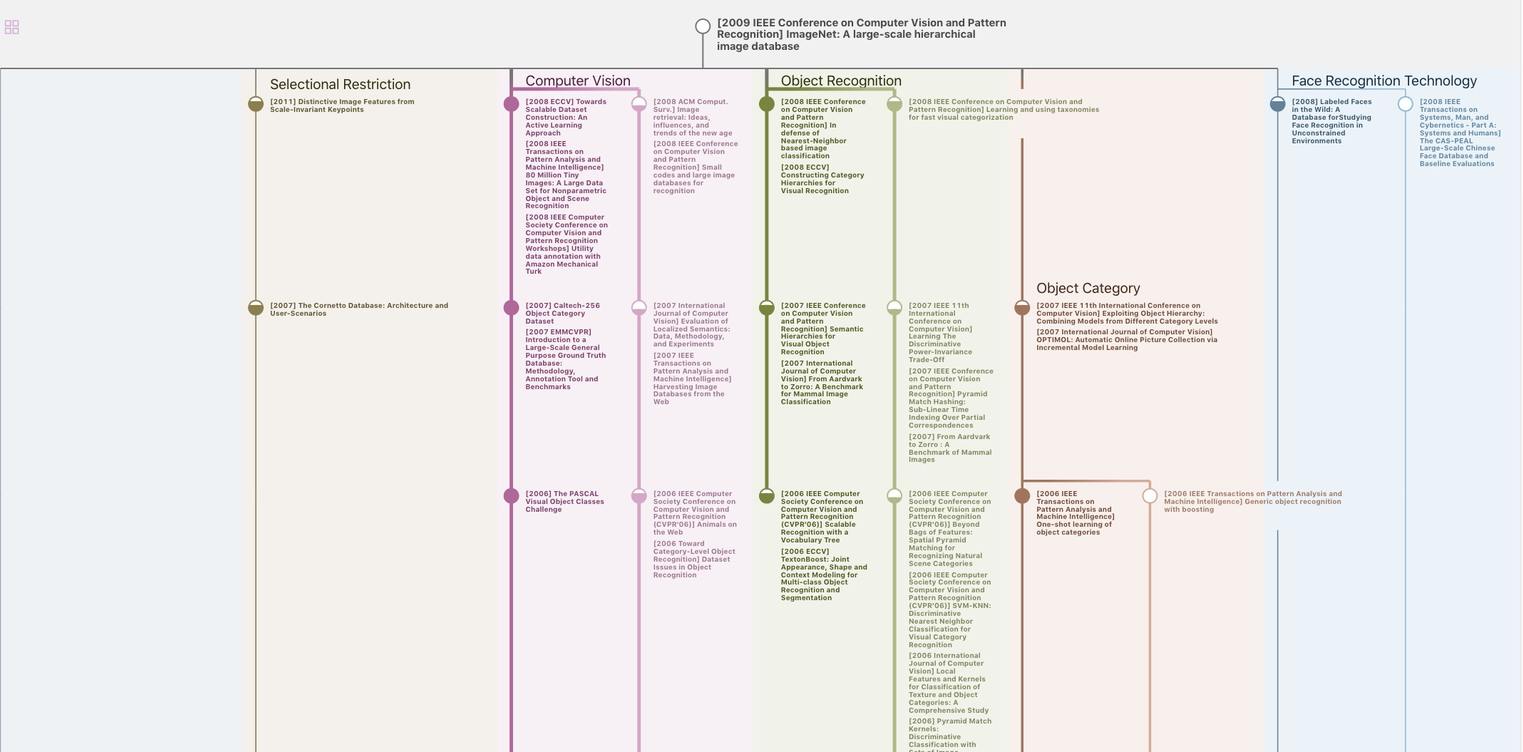
生成溯源树,研究论文发展脉络
Chat Paper
正在生成论文摘要