Error Detection on Knowledge Graphs with Triple Embedding
2023 31st European Signal Processing Conference (EUSIPCO)(2023)
摘要
Knowledge graphs (KGs), as essential ingredients in many real-world applications, always contain a considerable number of errors. KG error detection aims to find the triples whose head entity, tail entity, and corresponding relation are mismatched. Despite being urgently needed, existing KG error detection methods lack generalizability. They mainly utilize supervised information such as entity type or erroneous labels, but such information is not often available in the real world. The challenges in detecting errors in KGs are twofold. Firstly, KGs exhibit unique data characteristics that distinguish them from general graphs. Secondly, real-world KGs tend to be large, and labels are often scarce. To bridge the gap, we propose a novel KG error detection framework based on triple embedding, termed TripleNet. TripleNet constructs a triple network by treating each triple as a node and connecting them via shared entities. It then employs a Bi-LSTM module to capture intra-triple translational information at the local level and uses a graph attention network to gather inter-triple contextual information at the global level. Finally, it computes the suspicious score of each triple by integrating its local and global-level information. Experimental results on two real-world KGs demonstrated that TripleNet outperforms state-of-the-art error detection algorithms with comparable or even better efficiency.
更多查看译文
关键词
corresponding relation,entity type,erroneous labels,essential ingredients,general graphs,global-level information,graph attention network,inter-triple contextual information,intra-triple translational information,KG error detection methods,knowledge graphs,local level information,novel KG error detection framework,real-world applications,real-world KGs,shared entities,state-of-the-art error detection algorithms,supervised information,tail entity,termed TripleNet,triple embedding,triple network,TripleNet constructs,triples whose head entity,unique data characteristics
AI 理解论文
溯源树
样例
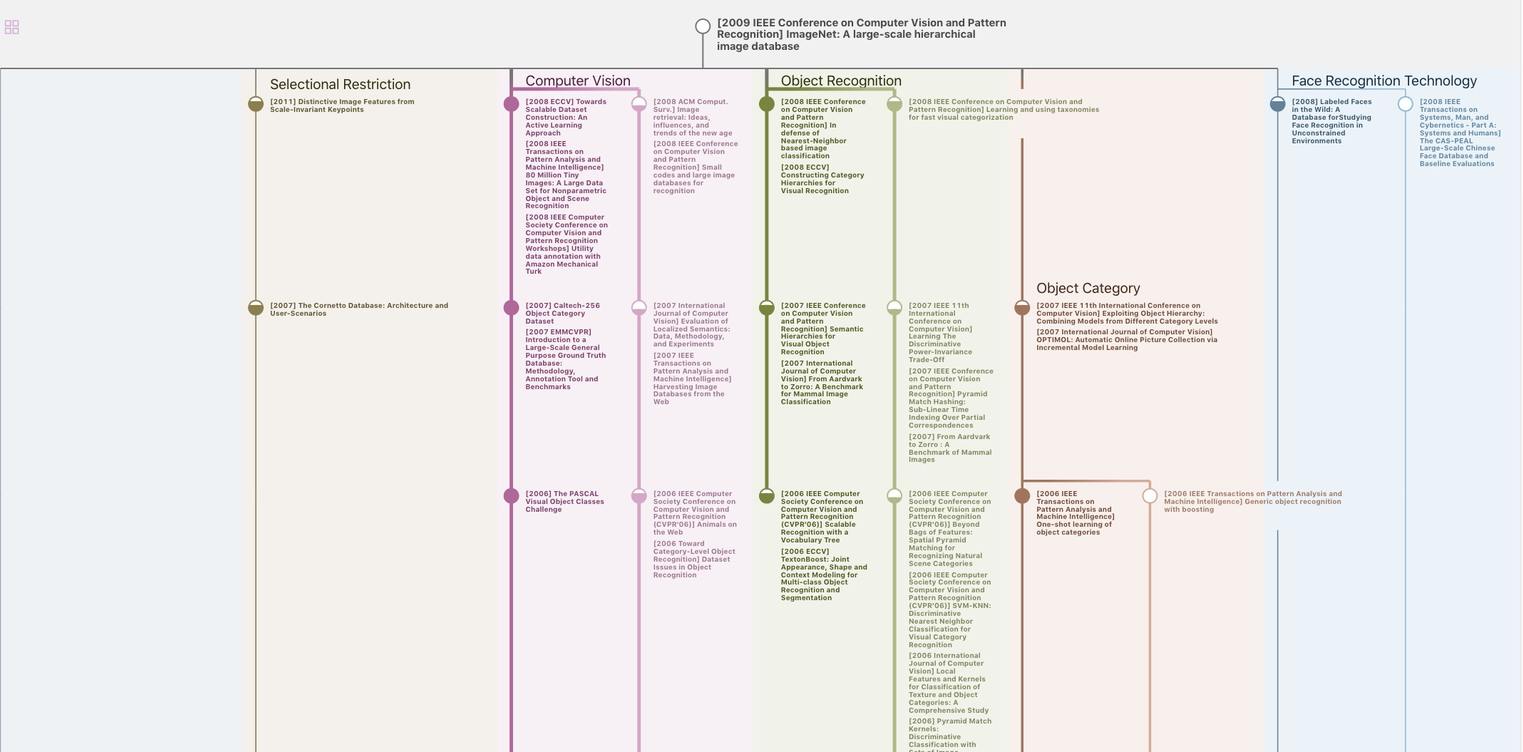
生成溯源树,研究论文发展脉络
Chat Paper
正在生成论文摘要