Marginal MAP Estimation of a Bernoulli-Gaussian Signal: Continuous Relaxation Approach
2023 31st European Signal Processing Conference (EUSIPCO)(2023)
摘要
We focus on recovering the support of sparse signals for sparse inverse problems. Using a Bernoulli-Gaussian prior to model sparsity, we propose to estimate the support of the sparse signal using the so-called Marginal Maximum a Posteriori estimate after marginalizing out the values of the nonzero coefficients. To this end, we propose an Expectation-Maximization procedure in which the discrete optimization problem in the M- step is relaxed into a continuous problem. Empirical assessment with simulated Bernoulli-Gaussian data using magnetoencephalo- graphic lead field matrix shows that this approach outperforms the usual
$\ell_{0}$
Joint Maximum a Posteriori estimation in Type-I and Type-II error for support recovery, as well as in SNR for signal estimation
更多查看译文
关键词
Sparse coding,inverse problem,Bernoulli- Gaussian model,Marginal-MAP,Joint-MAP
AI 理解论文
溯源树
样例
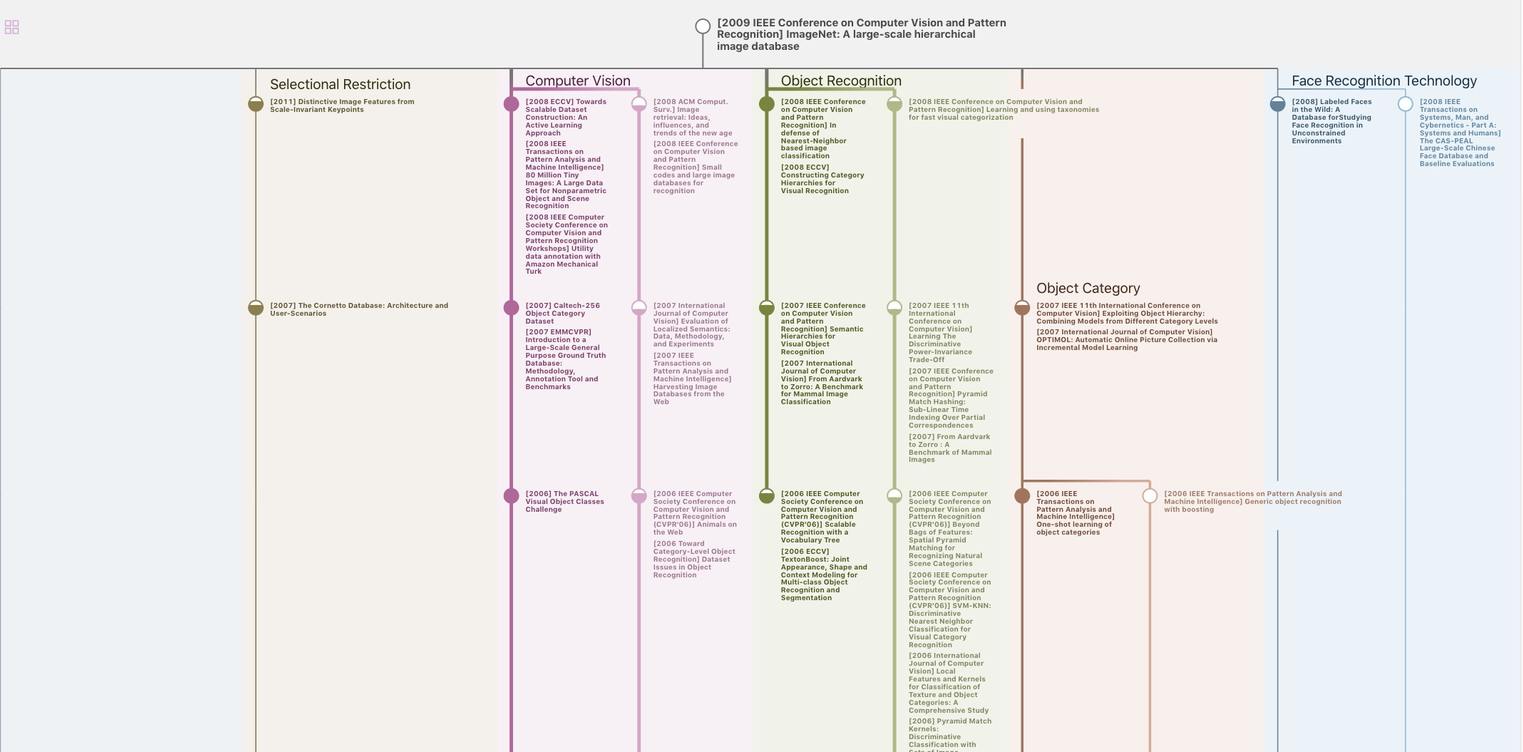
生成溯源树,研究论文发展脉络
Chat Paper
正在生成论文摘要