End-to-End Trainable Gaussian Filtering for Electrocardiogram Signal Classification Using Deep Learning
2023 31st European Signal Processing Conference (EUSIPCO)(2023)
摘要
Deep Learning (DL) is increasingly used in electro-cardiograms (ECGs)-based signal analysis allowing for diagnosing a variety of cardiac disorders and enabling the development of automated diagnosis and prognosis systems. However, ECG signals are typically noisy requiring a series of prepossessing steps before feeding them into DL models for analysis. The main contribution of this paper is a simple, yet very effective end-to-end hybrid trainable filtering and feature extraction pipeline, that is formulated as a set of differentiable layers that can be incorporated into any DL model and employ Gaussian filters as a prior model. Indeed, it is experimentally demonstrated that the proposed method can increase the performance of ECG-based classification both for baseline architectures (e.g., MLPs, CNNs, LSTMs) as well as for state-of-the-art architectures proposed in the literature for ECG signal analysis. Given the simplicity and effectiveness of the proposed method, we believe that it constitutes a practical tool that can readily be incorporated into any DL pipeline that is used for time-series analysis, potentially further increasing its performance with minimal computational overhead.
更多查看译文
关键词
electrocardiogram signal classification,data preprocessing,deep learning,trainable signal denoising,end-to-end trainable filtering
AI 理解论文
溯源树
样例
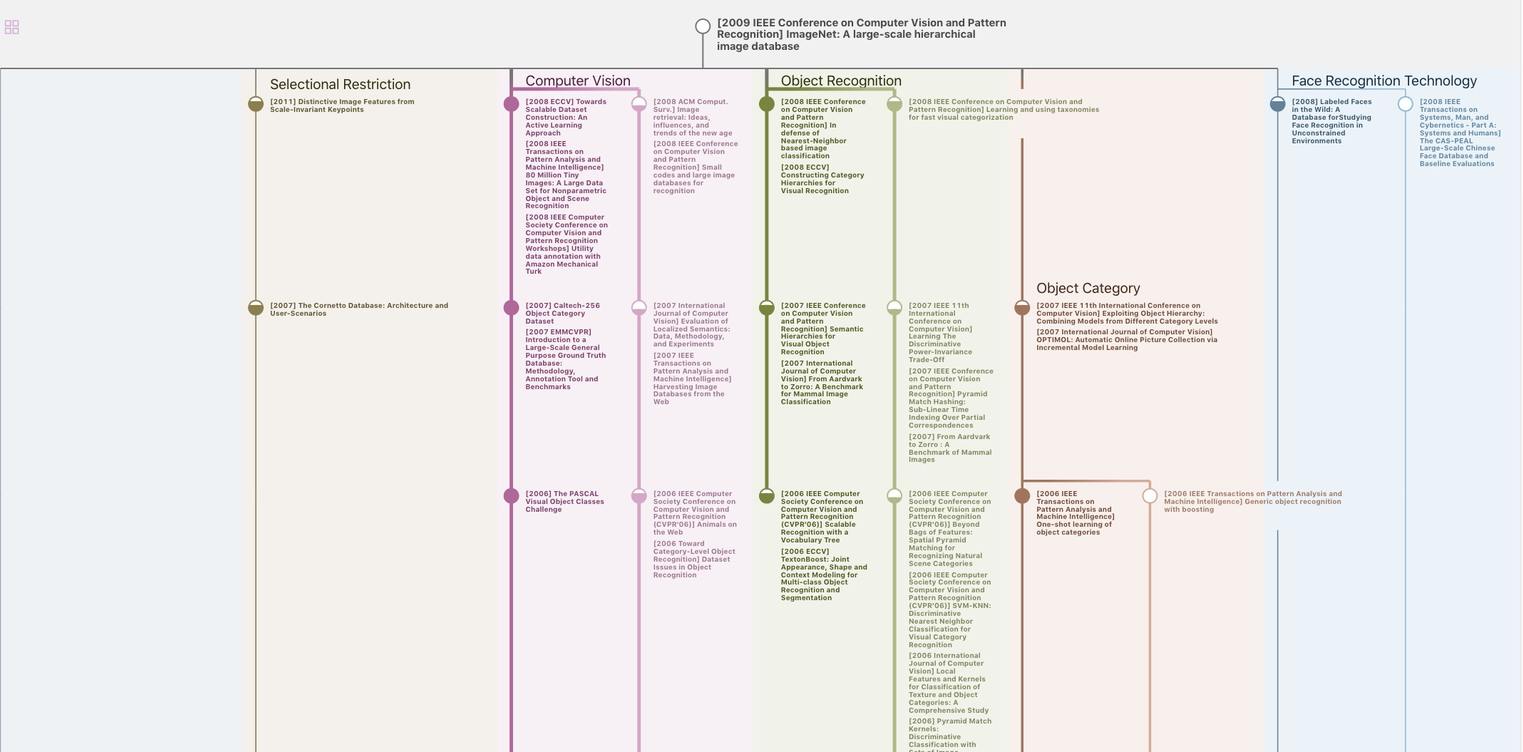
生成溯源树,研究论文发展脉络
Chat Paper
正在生成论文摘要