A Stacking Ensemble Learning Model for Rainfall Prediction based on Indian Climate
2023 6th International Conference on Information Systems and Computer Networks (ISCON)(2023)
摘要
Machine learning (ML) techniques, which are becoming more popular, have been used in different climates to show which ones are better. On top of that, the ensemble learning model, which takes the best parts of different ML models and puts them together, deserves more attention. In this study, a stacking-based ensemble learning model is used to predict rain based on Indian climate parameters. Rainfall is vital for agriculture. Rainfall has been hard to predict in recent years because the weather is always changing. Predicting rain makes people more aware and helps them plan ahead so they can protect their crops from rain. There are now a lot of ways to predict rain. The goal of this paper is to give clients from different fields information about climate so they can understand how important changes in climate and the environment are. Precipitation, temperature, and other aspects of the atmosphere, such as humidity, etc. Estimating how much rain will fall is a very important question in the science of meteorology. Linear Regression, Random Forest, Logistic Regression, Extreme gradient boosting (XGB), and Support vector regression (SVR), are used as base models. As a second-layer learner, the weighting algorithm combines the results from the base models to make predictions. For testing, daily observations were thought about. We used India’s rainfall data to test the accuracy of regressors such as SVM, Linear Regression, Logistic Regression, Random Forest, Gradient Boosting, and XGBoost. Our goal is to get the best possible result and a more accurate prediction of rainfall. To do this, we stacked and ensembled the above-mentioned regressors, and the accuracy of these hybrid models increased exponentially, with a maximum accuracy of 81.2%. This means that using hybrid models helps predict rainfall more accurately. Overall, performance evaluation measures show that the proposed stacking ensemble multi-ML model can provide a flexible and reasonable framework for making predictions that can be used in other regions.
更多查看译文
关键词
Rainfall Prediction,Machine Learning,Regression,Ensemble Stacking
AI 理解论文
溯源树
样例
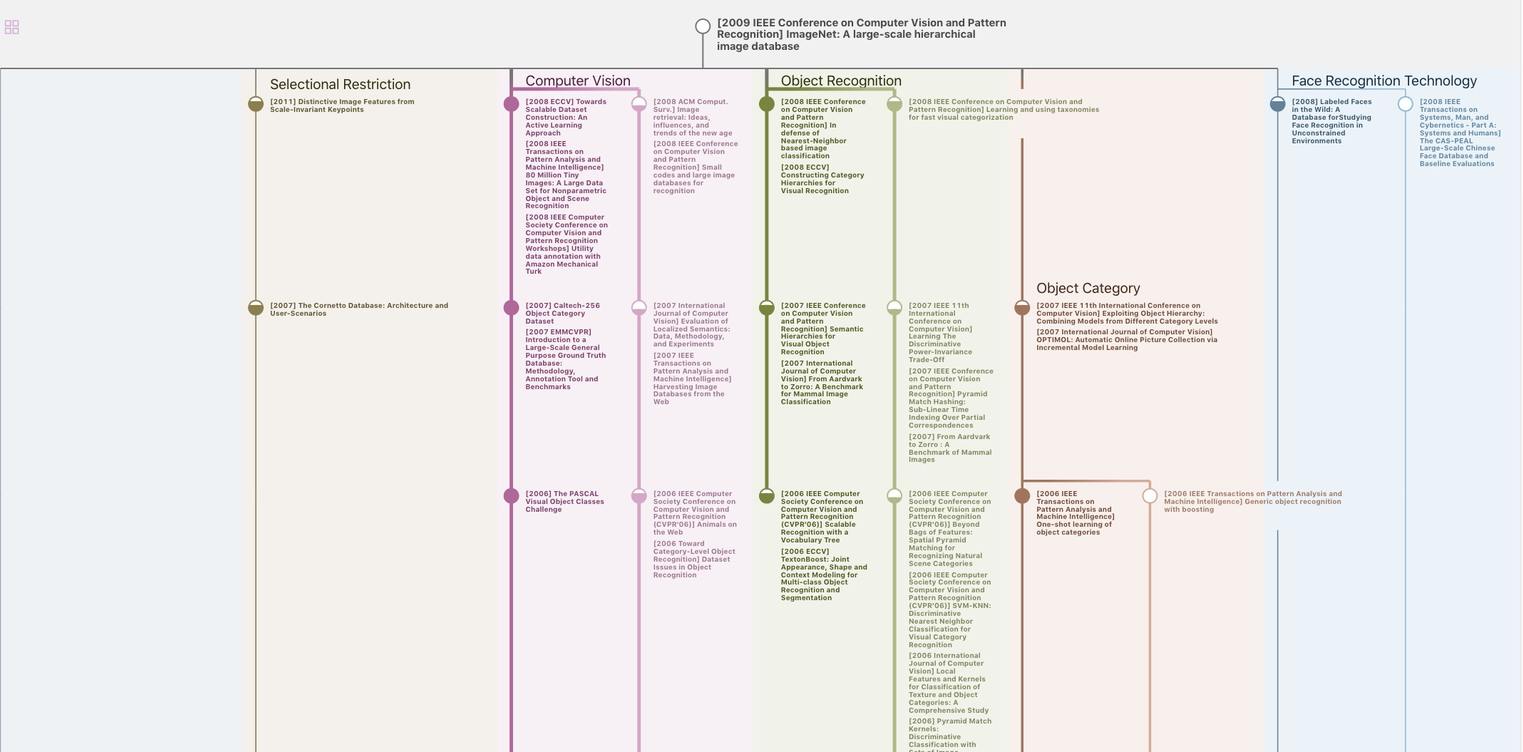
生成溯源树,研究论文发展脉络
Chat Paper
正在生成论文摘要