Tomato Crop Diseases Detection and Classification using ResNet50, DenseNet and Bins Approach
2023 4th International Conference for Emerging Technology (INCET)(2023)
摘要
Detection and Classification of various crop diseases can help to prevent a disastrous situation. If left undetected, many diseases damage the plant, thus reducing the crop yield produced by such plants. Therefore, in this paper, two diseases have been worked on. The two tomato plant diseases that have been focused on are Tomato leaf mold and Tomato target spot. Past practices to detect these diseases have proven to be time-consuming and inefficient due to poor accuracy. The primary focus of this work is to mainly detect these diseases accurately and quickly, because such diseases need to be detected in the premature stage to avoid any major harm to the plant's yield. Proposed system is aimed at addressing the existing challenges. It accepts the image as an input that will be preprocessed and will undergo the detection and classification stages. Feature extraction plays a significant role in such systems. This research contribution mainly deals with training the system using deep learning (DL) models and spatial domain image processing technique namely Convolution Neural Networks (CNN) with ResNet50, DenseNet and histogram partitioning based Bins approach. The novelty added by applying Bins approach for feature extraction clearly defines the image contents being focused on as features which aids in choosing the significant features and ignoring the unwanted features that helps in reducing the feature dimensionality. Further it is followed by application of various classifiers such as Logistic Regression, Linear SVM and Decision Trees over bins feature space. All the proposed algorithms are applied on a common dataset called PlantVillage and their performances are evaluated, analyzed and compared using the same set of parameters like precision, recall, accuracy and F1-score. Results obtained reflect that all the approaches achieved accuracy greater than 90%. Proposed novelty by Bins approach proved equally better with Logistic Regression for all the parameters.
更多查看译文
关键词
Tomato leaf mold,Tomato target spot,preprocessing,image processing,CNN,feature extraction,texture features,Bins Approach,detection,classification,Logistic Regression,ResNet50,DenseNet,SVM,Decision Trees
AI 理解论文
溯源树
样例
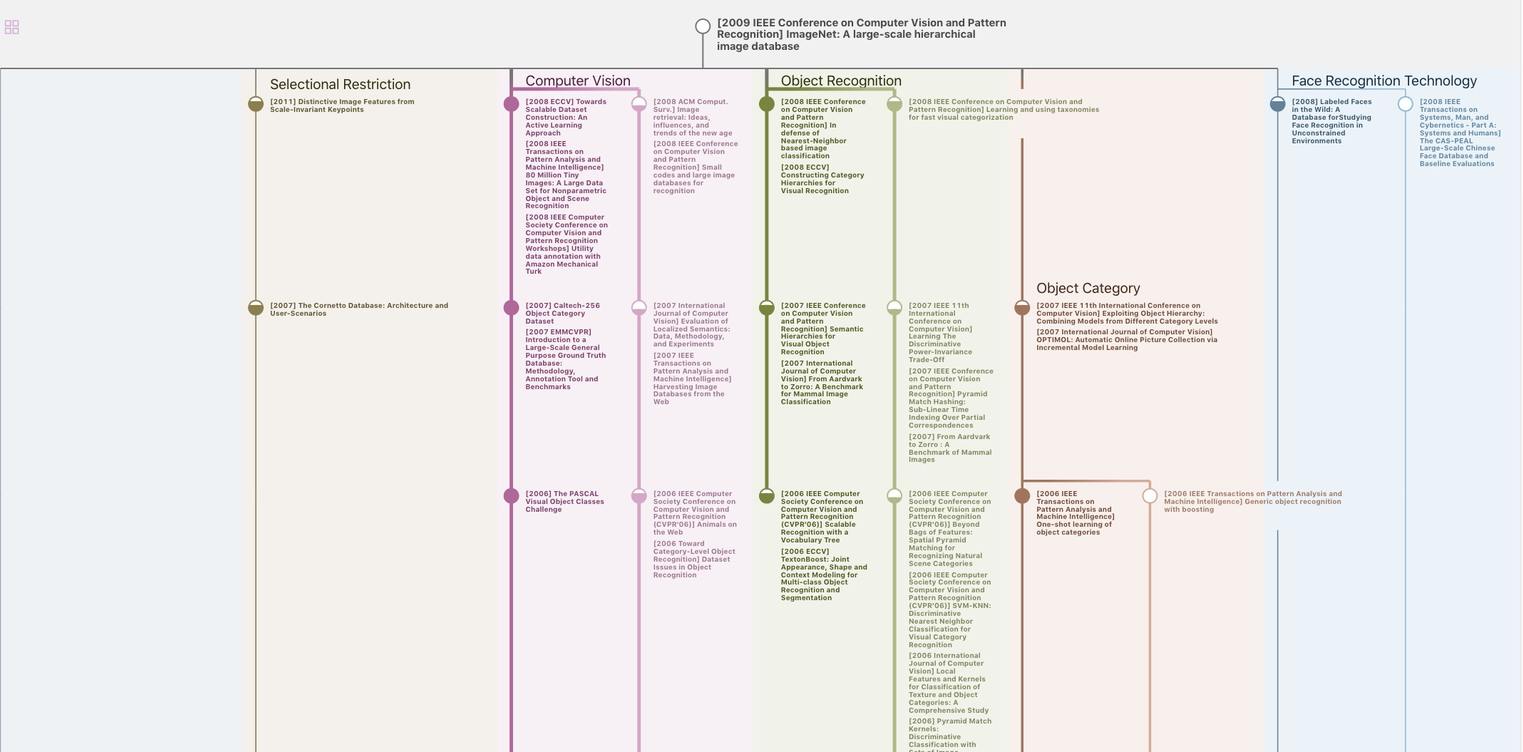
生成溯源树,研究论文发展脉络
Chat Paper
正在生成论文摘要