Examining Generative Adversarial Network for Smart Home DDoS Traffic Generation
2023 International Symposium on Networks, Computers and Communications (ISNCC)(2023)
摘要
Adversarial attacks have become a common place in network security. Neural network-based traffic classifiers have been regarded as effective tools against malicious attacks. However, their performance highly depends on the quality of the training dataset that is often hard to obtain. IoT-centric smart home network is vulnerable to adversarial attacks with a high cost to the individual. In this research, we perform a thorough study on the performance of the original GAN model towards generating flow-based IoT traffic in smart home DDoS attacks. Based on a unique IoT traffic dataset of smart home, we implemented four versions of the original GAN model by using four batch sizes per epoch during training. We captured synthetic IoT traffic at different epochs of the models, which results in a total of 200 IoT traffic datasets. Then, we evaluate the quality of the 200 synthetic datasets using an approach called train-on-synthetic, test-on-real (TSTR). Our study suggests that the original GAN can produce a quality IoT traffic of smart home DDoS attacks at most of the epochs but lacks in providing consistent performance across all the epochs of the GAN model. However, by using TSTR metrics, it is possible to identify the datasets of good quality to be used for real applications.
更多查看译文
关键词
Classifiers,DDoS Attacks,Generative Adversarial Network (GAN),Intrusion Detection,Internet of Things (IoT),Neural Networks,Smart Home,Traffic Generation
AI 理解论文
溯源树
样例
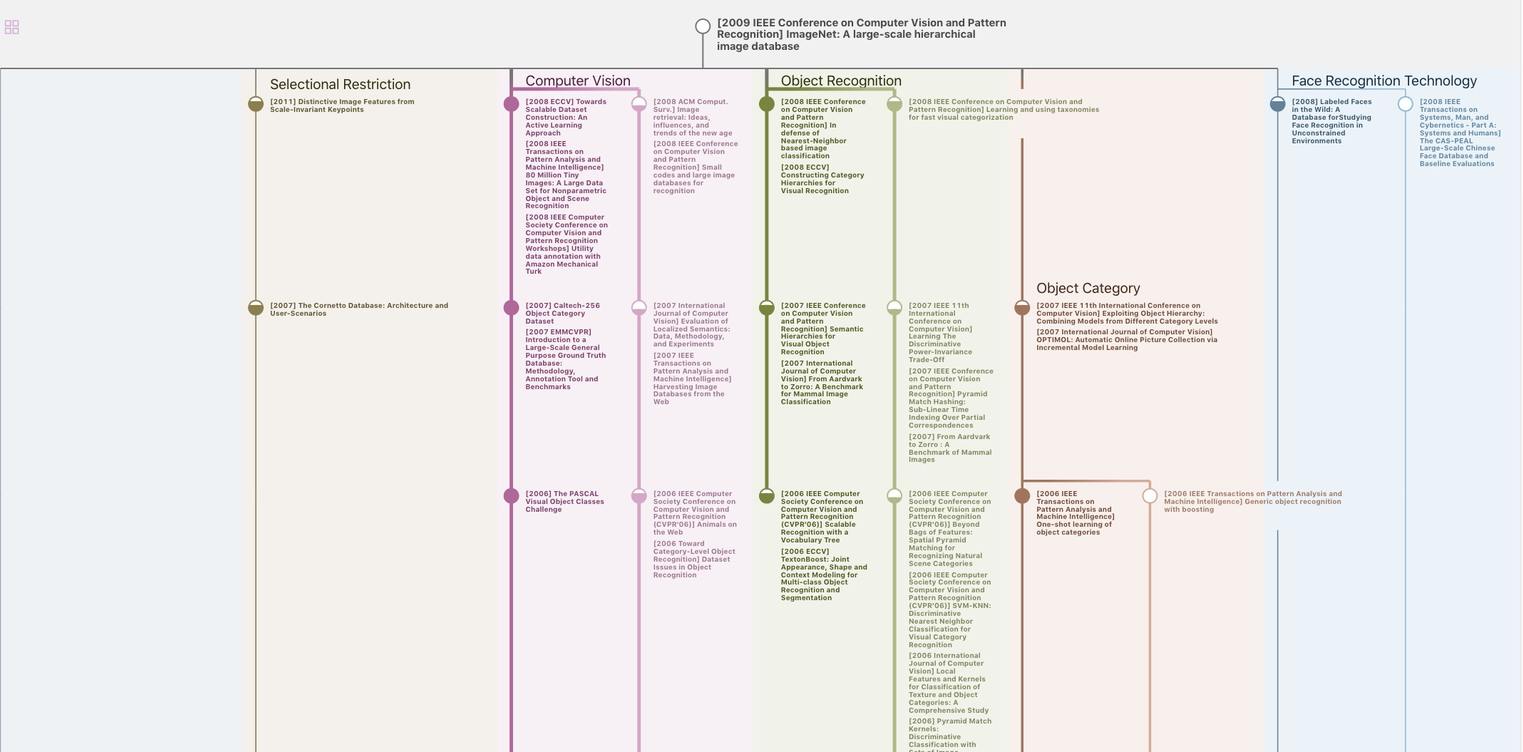
生成溯源树,研究论文发展脉络
Chat Paper
正在生成论文摘要