CPLT: Curriculum Pseudo Label Transformer for Domain Adaptive Object Detection in Foggy Weather
IEEE sensors journal(2023)
摘要
To address the challenge of label-scarce images captured by sensor in foggy weather condition with limited labeled data for autonomous driving, domain adaptation can be applied to transfer information from a label-rich clear-weather dataset to a label-scarce adverse weather dataset. This article proposes a novel approach named curriculum pseudo label transformer (CPLT) for domain adaptive object detection (DAOD). CPLT incorporates two effective techniques to enhance the transformer’s ability to detect objects across domain. First, distribution alignment curriculum (DAC) pseudo label is proposed. The key idea of DAC is to flexibly adjust class-specific thresholds by aligning the class distribution over the course of training. The second technique employed in CPLT is called instance-level feature optimal transport (IFOT), which applies sliced Wasserstein distance to minimize domain discrepancy in instance-level features while preserving the location information. Importantly, neither of these modules introduce additional trainable parameters in the network. Experimental results on five challenging benchmarks demonstrate that CPLT significantly improves domain adaptation object detection performance.
更多查看译文
关键词
Detection transformer (DETR),domain adaptation,flexible threshold,object detection,sliced Wasserstein distance
AI 理解论文
溯源树
样例
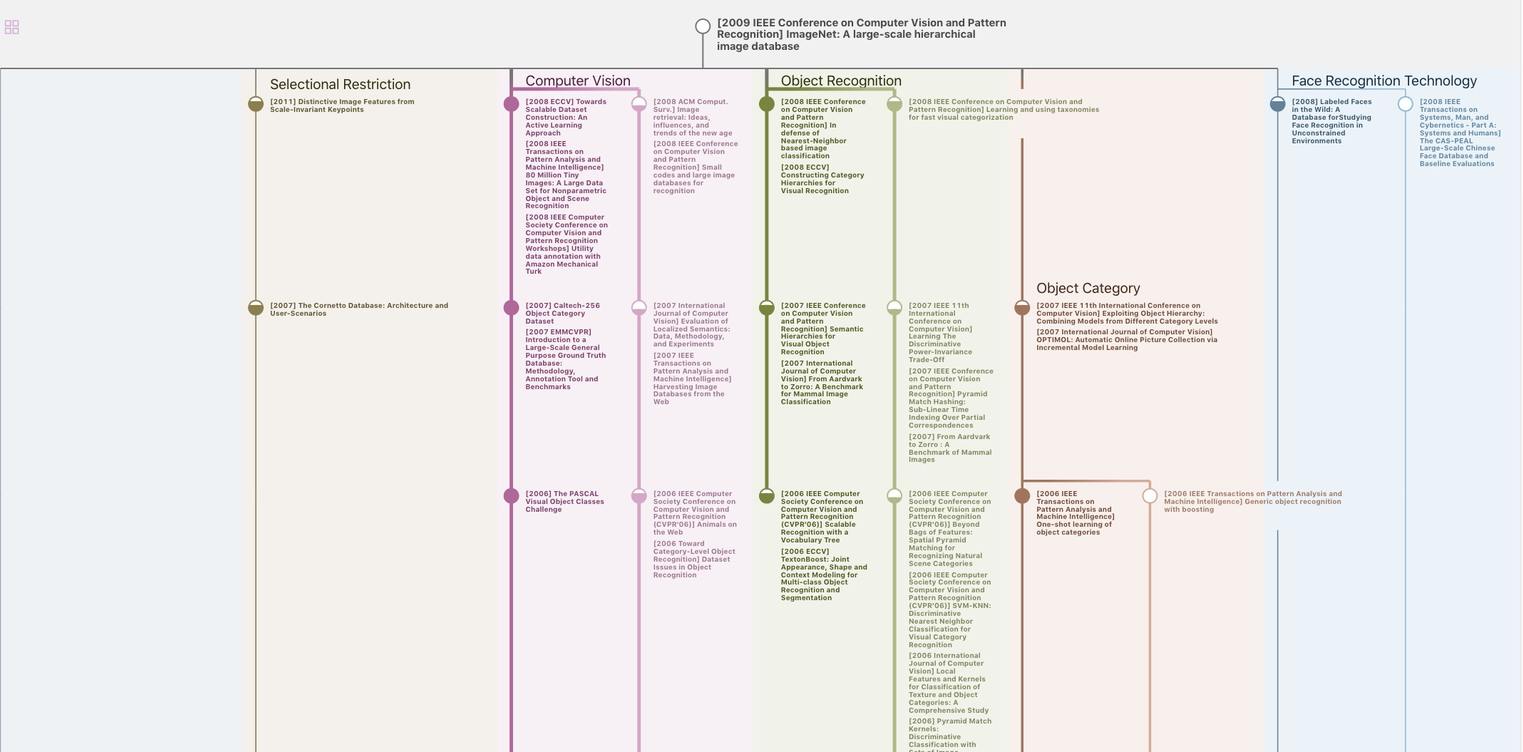
生成溯源树,研究论文发展脉络
Chat Paper
正在生成论文摘要