A Novel Approach for Predicting Remaining Useful Life and Capacity Fade in Lithium-Ion Batteries Using Hybrid Machine Learning
IEEE Access(2023)
摘要
Since lithium-ion batteries (LIBs) are essential to many different sectors, accurate estimates of their Remaining Useful Life (RUL) are necessary to maximize Battery Management Systems (BMS). In this study, we introduce an innovative approach that combines machine learning techniques to create a hybrid model, enhancing the precision and reliability of battery analysis. Our proposed model leverages the power of k-Nearest Neighbors (kNN), Random Forest (RF), and Extreme Gradient Boosting (XGBoost) algorithms to capture complex relationships and patterns in battery data effectively. Our major objective is to precisely estimate the residual energy and RUL of LIBs, allowing for the efficient evaluation of battery health and deterioration over time. We meticulously curate a comprehensive dataset comprising essential battery parameters, including capacity, voltage, cycle, and temperature. The proposed hybrid model achieves impressive results with an R2 value of 0.996457, a minimal RMSE of 0.016861, and a low MAE of 0.008956. Our analysis provides valuable insights for optimizing battery performance, informed maintenance planning, and enhancing energy storage system efficiency.
更多查看译文
关键词
Charge cycles,lithium-ion batteries,RUL,capacity fade,battery performance,feature selection
AI 理解论文
溯源树
样例
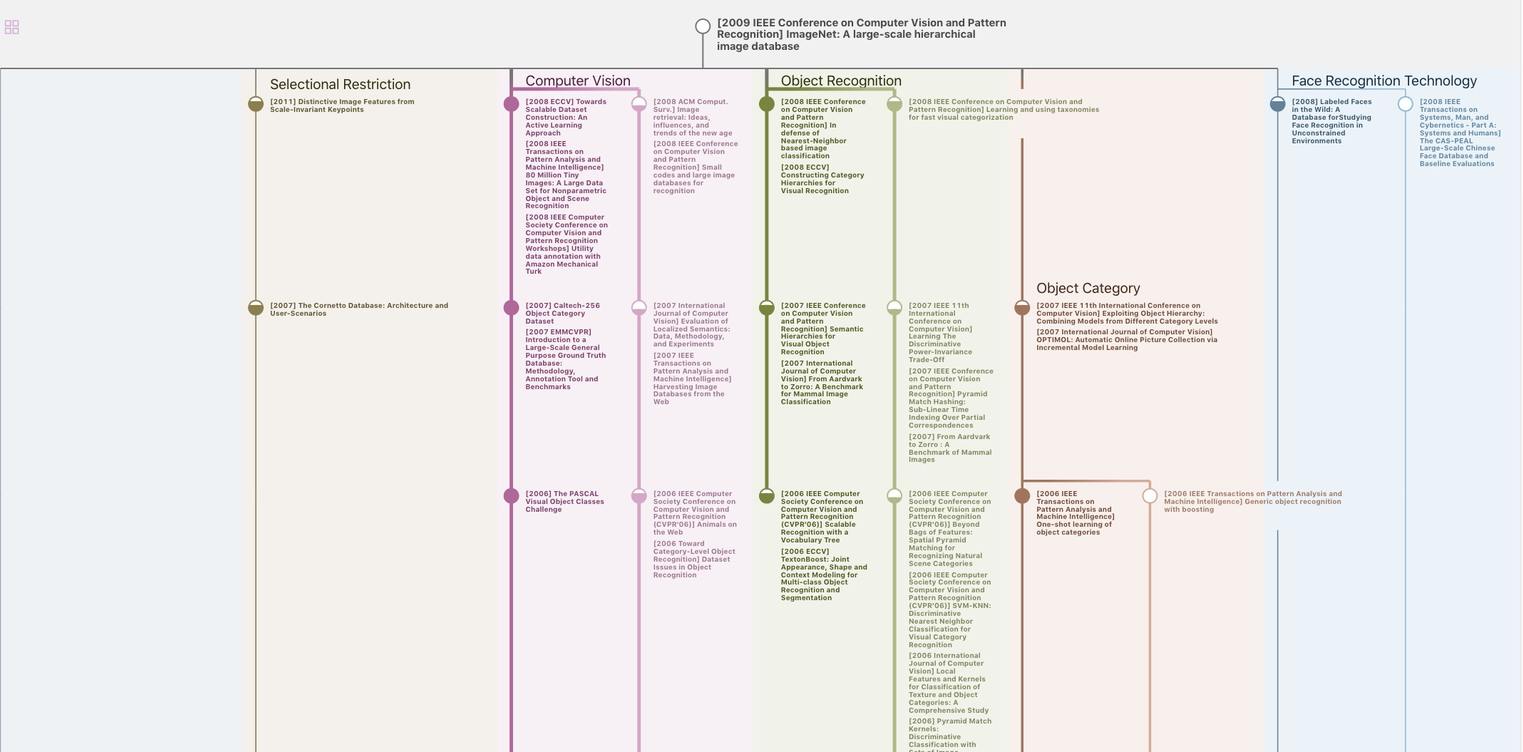
生成溯源树,研究论文发展脉络
Chat Paper
正在生成论文摘要