Seamless Reconstruction of AMSR-E Land Surface Temperature Swath Gaps for Chinas Landmass
IEEE TRANSACTIONS ON GEOSCIENCE AND REMOTE SENSING(2023)
摘要
All-weather land surface temperature (LST) derived from passive microwave (PMW) sensors has significant implications for characterization on the physical processes of surface energy and water balance at local through global scales. However, the PMW sensors (e.g., the Advanced Microwave Scanning Radiometer-Earth Observing System (AMSR-E)] suffer from swath gaps cannot provide completely spatial-gapless observations. The existing multitemporal feature connection-convolutional neural network (MTFC-CNN) method caused obvious traces of "gaps" when the sample number is small or features are not rich. This article proposes a sample optimized-MTFC (SO-MTFC) seamless reconstruction method based on analyzing the periodicity and complementarity of AMSR-E swath gaps. Sample optimization includes two aspects: sample enhancement (SE) and single-cycle mask (SCM) strategy. Taking China's landmass as the study area, experimental results show that the original AMSR-E LSTs and the reconstructed AMSR-E LSTs are basically connected seamlessly. Validation against with the MODIS LSTs shows that the daytime (nighttime) root-mean-squared errors (RMSEs) of the original and the reconstructed AMSR-E LSTs are 3.87 (2.57 K) and 4.76 K (2.96 K), respectively, while the corresponding daytime (nighttime) $R<^>{2}$ are 0.88 (0.94) and 0.73 (0.90), respectively. Validation against with the six in situ LSTs shows that the RMSEs and $R<^>{2}$ of reconstructed AMSR-E LSTs against in situ LSTs are almost consistent with those of the original AMSR-E LST. The ablation study proves the effectiveness of the sample optimization. These findings indicated that the SO-MTFC achieved a good reconstruction effect. Compared with the MTFC-CNN, the SO-MTFC got higher scores visually and quantitatively. The SO-MTFC can potentially be implemented with other satellite PMW sensors to produce completely spatial-seamless PMW LST records on a global scale.
更多查看译文
关键词
Land surface temperature,Image reconstruction,Spatial resolution,Surface reconstruction,Land surface,Clouds,Training,Advanced Microwave Scanning Radiometer-Earth Observing System (AMSR-E),deep learning (DL),land surface temperature (LST),reconstruction,sample optimization
AI 理解论文
溯源树
样例
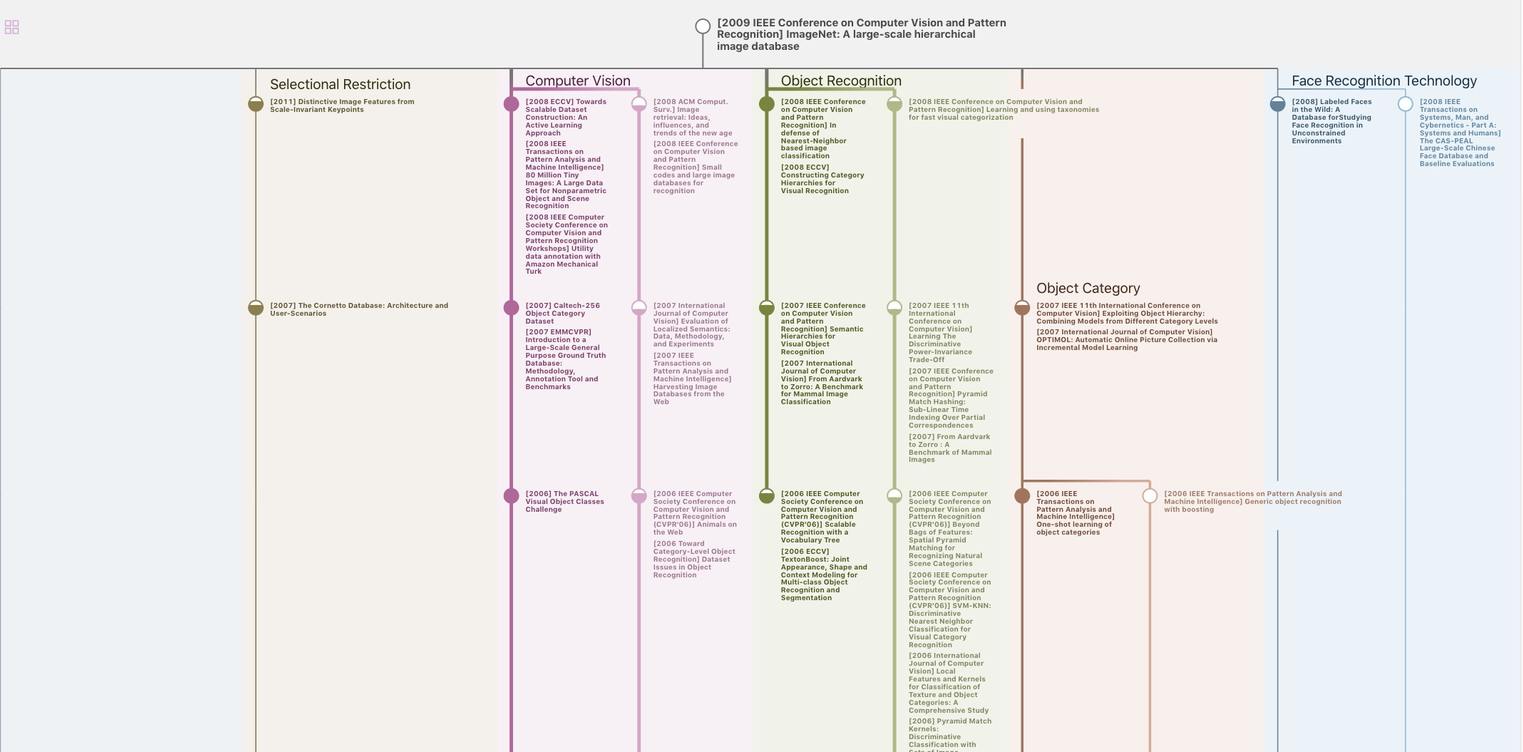
生成溯源树,研究论文发展脉络
Chat Paper
正在生成论文摘要