Deep Neural Networks for Direct Hydrocarbon Detection in Prestack Seismic Data Based on AVO Analysis
IEEE Transactions on Geoscience and Remote Sensing(2023)
摘要
Hydrocarbon detection remains a significant focus in geophysical exploration as it directly reflects production potential. The amplitude variation with offset (AVO) theory supports hydrocarbon detection using prestack seismic data, but its applicability is currently low in deep hydrocarbon exploration. In this study, AVO characteristics from sidetrack data are harnessed as inputs, accompanied by hydrocarbon traits as labels. A deep neural network (DNN) is a direct application that establishes an all-encompassing correlation between the data and hydrocarbon content. By meticulously training thoughtfully chosen network parameters, a predictive network is formulated to enable a comprehensive approach to hydrocarbon detection. This methodology diminishes the impact of human variables, embraces data-derived results, augments feasibility and adaptability, and enables a direct form of hydrocarbon detection. The effectiveness of the proposed methodology is substantiated by means of analyzing both the Marmousi2 model data and authentic data obtained from the Leikoupo Formation in Western Sichuan, exhibiting an accuracy rate exceeding 90%. By comparing evaluations with conventional AVO theory methods, the DNN method indicates a significant improvement in accuracy, thus providing an exemplary approach for direct hydrocarbon detection.
更多查看译文
关键词
Hydrocarbons,Artificial neural networks,Reservoirs,Neurons,Windows,Training,Deep learning,Carbonate reservoirs,deep neural network (DNN),direct hydrocarbon indication (DHI)
AI 理解论文
溯源树
样例
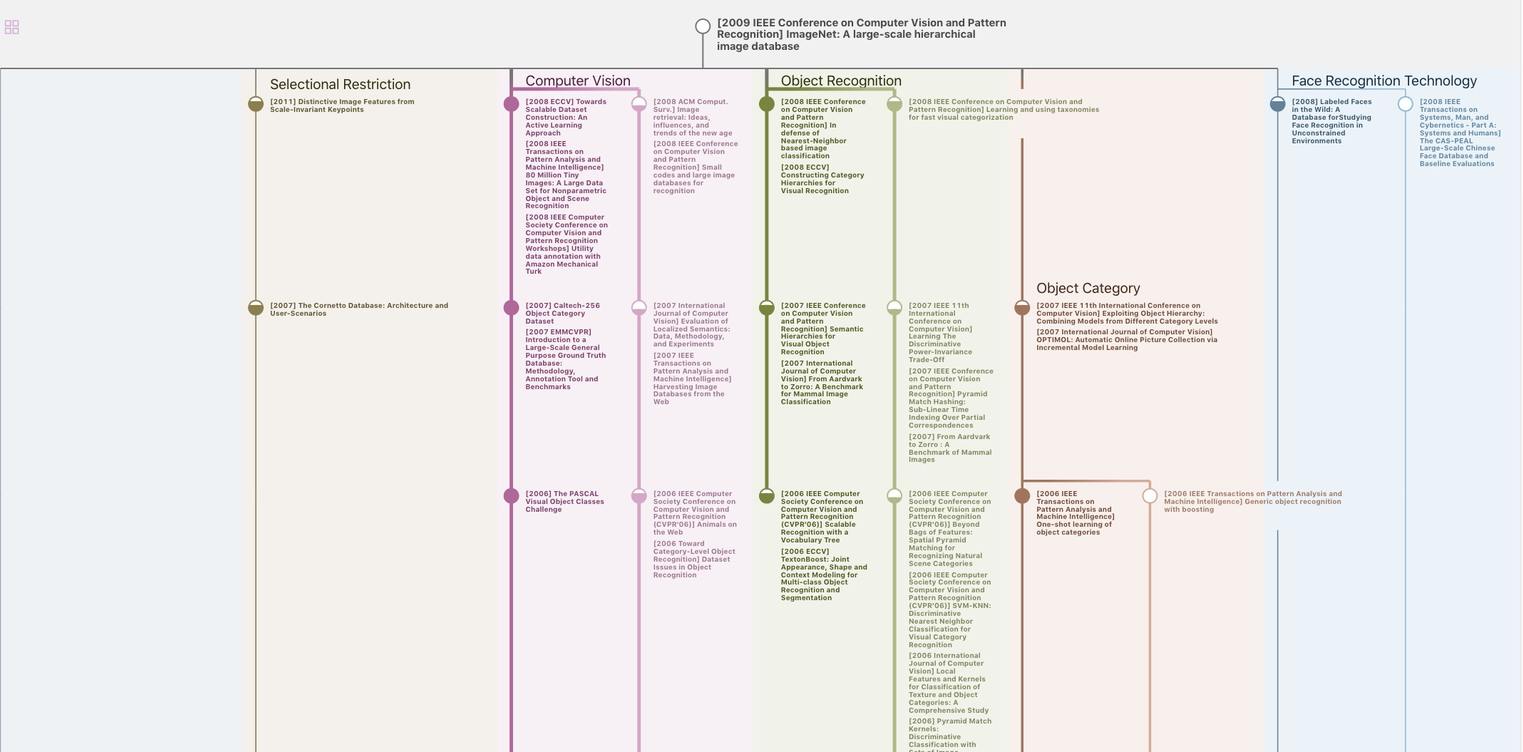
生成溯源树,研究论文发展脉络
Chat Paper
正在生成论文摘要