Developing a Novel Long Short-Term Memory Networks with Seasonal Wavelet Transform for Long-Term Wind Power Output Forecasting
International Journal of Computational Intelligence Systems(2023)
摘要
Long-term wind power forecasting is a challenging endeavor that requires predictions that span years into the future. Accurate forecasting is crucial for optimizing energy production, grid integration, maintenance scheduling, and financial planning. This study attempts to first develop the long short-term memory networks (LSTM) with a seasonal wavelet transform forecasting model for practical long-term wind power forecasting problems with seasonal and regional influences on wind power and the instability of data signals. This model encapsulates wavelet transformation and seasonal decomposition, and employs LSTM for forecasting. The new prediction model adopted seasonal decompositions and two LSTMs to approach low- and high-frequency series datasets, as well as the wavelet synthesis prediction values. Furthermore, the parameters of the LSTM models are selected using stochastic optimization. For a comprehensive evaluation, the proposed LSTM with seasonal wavelet transform is compared with seven methods, including seasonal LSTM (SLSTM), wavelet LSTM (WLSTM), and the seasonal auto-regressive integrated moving average (SARIMA), back propagation neural network (BPNN), generalized regression neural network (GRNN), least square support vector regression (LSSVR), and support vector regression (SVR) were employed for long-term wind power output forecasting of wind farms. The empirical results underscore that the performance of the proposed forecasting model is better than other methods in terms of forecasting accuracy, which could efficiently provide reliable long-term predictions for long-term wind power output forecasting.
更多查看译文
关键词
Long short-term memory networks,Wavelet transform,Seasonal composition,Long-term wind power output forecasting
AI 理解论文
溯源树
样例
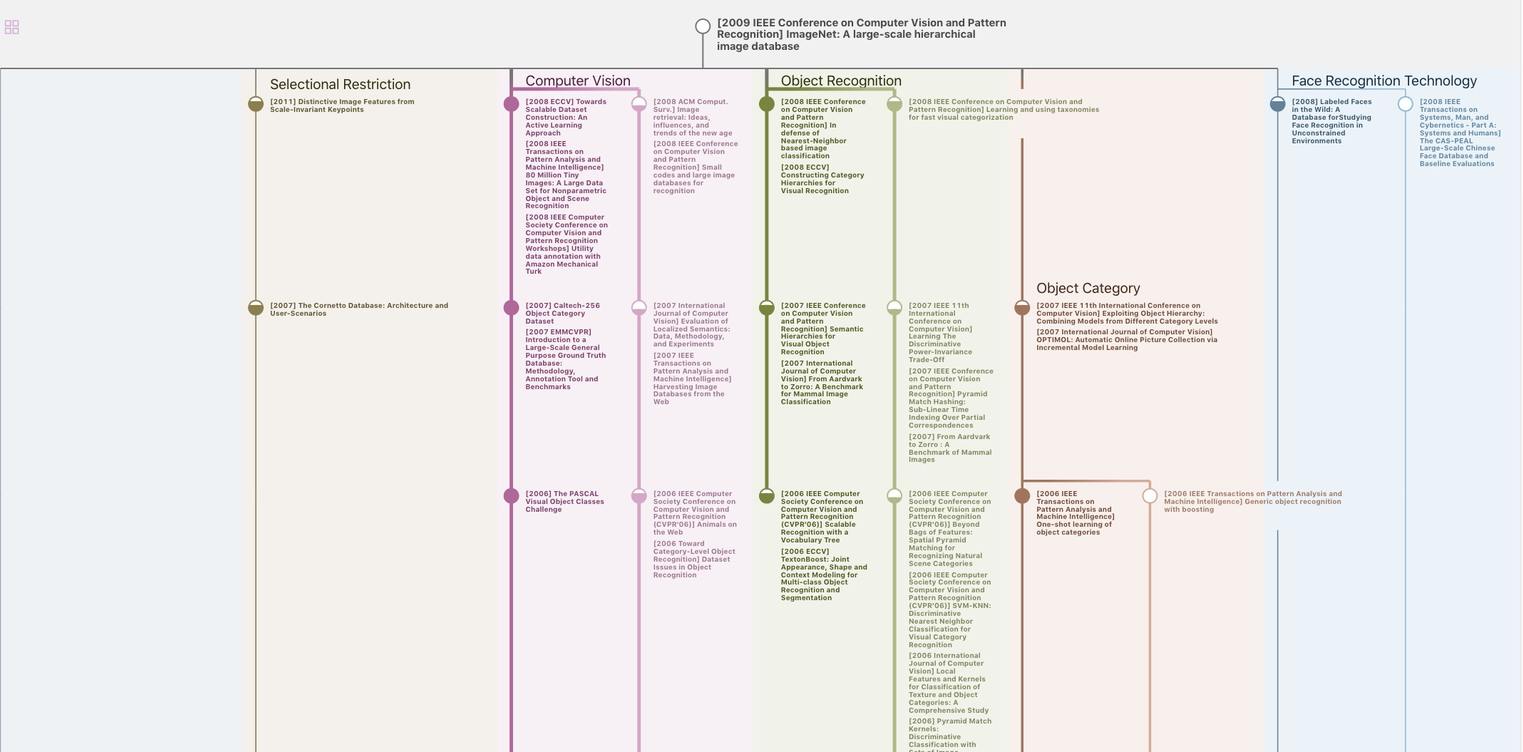
生成溯源树,研究论文发展脉络
Chat Paper
正在生成论文摘要