Inference using a composite-likelihood approximation for stochastic metapopulation model of disease spread
arxiv(2023)
摘要
Spatio-temporal pathogen spread is often partially observed at the
metapopulation scale. Available data correspond to proxies and are incomplete,
censored and heterogeneous. Moreover, representing such biological systems
often leads to complex stochastic models. Such complexity together with data
characteristics make the analysis of these systems a challenge. Our objective
was to develop a new inference procedure to estimate key parameters of
stochastic metapopulation models of animal disease spread from longitudinal and
spatial datasets, while accurately accounting for characteristics of census
data. We applied our procedure to provide new knowledge on the regional spread
of \emph{Mycobacterium avium} subsp. \emph{paratuberculosis} (\emph{Map}),
which causes bovine paratuberculosis, a worldwide endemic disease. \emph{Map}
spread between herds through trade movements was modeled with a stochastic
mechanistic model. Comprehensive data from 2005 to 2013 on cattle movements in
12,857 dairy herds in Brittany (western France) and partial data on animal
infection status in 2,278 herds sampled from 2007 to 2013 were used. Inference
was performed using a new criterion based on a Monte-Carlo approximation of a
composite likelihood, coupled to a numerical optimization algorithm
(Nelder-Mead Simplex-like). Our criterion showed a clear superiority to
alternative ones in identifying the right parameter values, as assessed by an
empirical identifiability on simulated data. Point estimates and profile
likelihoods allowed us to establish the initial state of the system, identify
the risk of pathogen introduction from outside the metapopulation, and confirm
the assumption of the low sensitivity of the diagnostic test. Our inference
procedure could easily be applied to other spatio-temporal infection dynamics,
especially when ABC-like methods face challenges in defining relevant summary
statistics.
更多查看译文
AI 理解论文
溯源树
样例
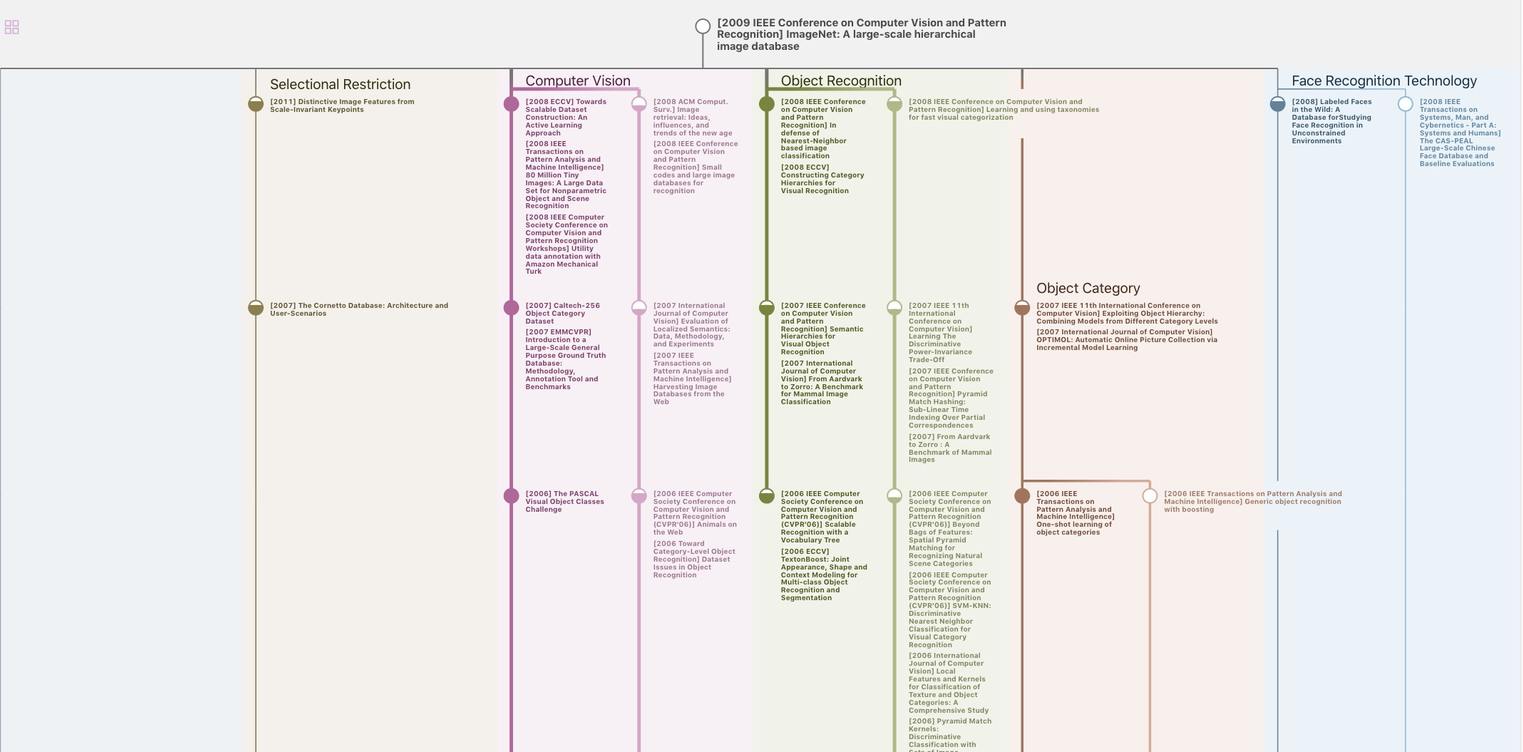
生成溯源树,研究论文发展脉络
Chat Paper
正在生成论文摘要