A multi-objective QoS-aware IoT service placement mechanism using Teaching Learning-Based Optimization in the fog computing environment
Neural Computing and Applications(2024)
摘要
The huge amount and diversity of data generated by Internet of Things (IoT) devices and the need to store and process this data led to the development of fog computing alongside cloud computing. Fog computing is a new paradigm for providing service at the edge of the network and close to end users, so that it can support real-time IoT applications. Because fog involves heterogeneous and distributed infrastructure with limited resources, so efficient resource allocation to satisfy Quality of Service (QoS) is challenging. IoT application placement mechanisms have been developed to address these issues, in which the subordinate services of these applications are mapped to fog nodes. Despite extensive research to solve the Service Placement Problem (SPP) in fog computing, efforts are still ongoing due to the importance of the issue. Hence, this paper proposes an efficient and autonomous mechanism for solving SPP using Teaching Learning-Based Optimization (TLBO) called SPP-TLBO. SPP-TLBO is a multi-objective QoS-aware algorithm that manages resources on distributed and localized fog domains. In addition to the above, we improve the performance of TLBO by configuring the evolution process with a shared parallel architecture. Besides, SPP-TLBO can save more resources to handle future requests by considering application deadlines and extracting the dynamic distribution of resources required over time. The proposed algorithm is evaluated by simulation on a synthetic fog environment. The simulation results show that SPP-TLBO improves system performance and is between 8 and 19% better efficiency compared to some advanced methods such as CSA-FSPP, FSP-ODMA and, WOA-FSP.
更多查看译文
关键词
Fog computing environment,IoT service placement,Autonomous mechanism,Teaching Learning-Based Optimization
AI 理解论文
溯源树
样例
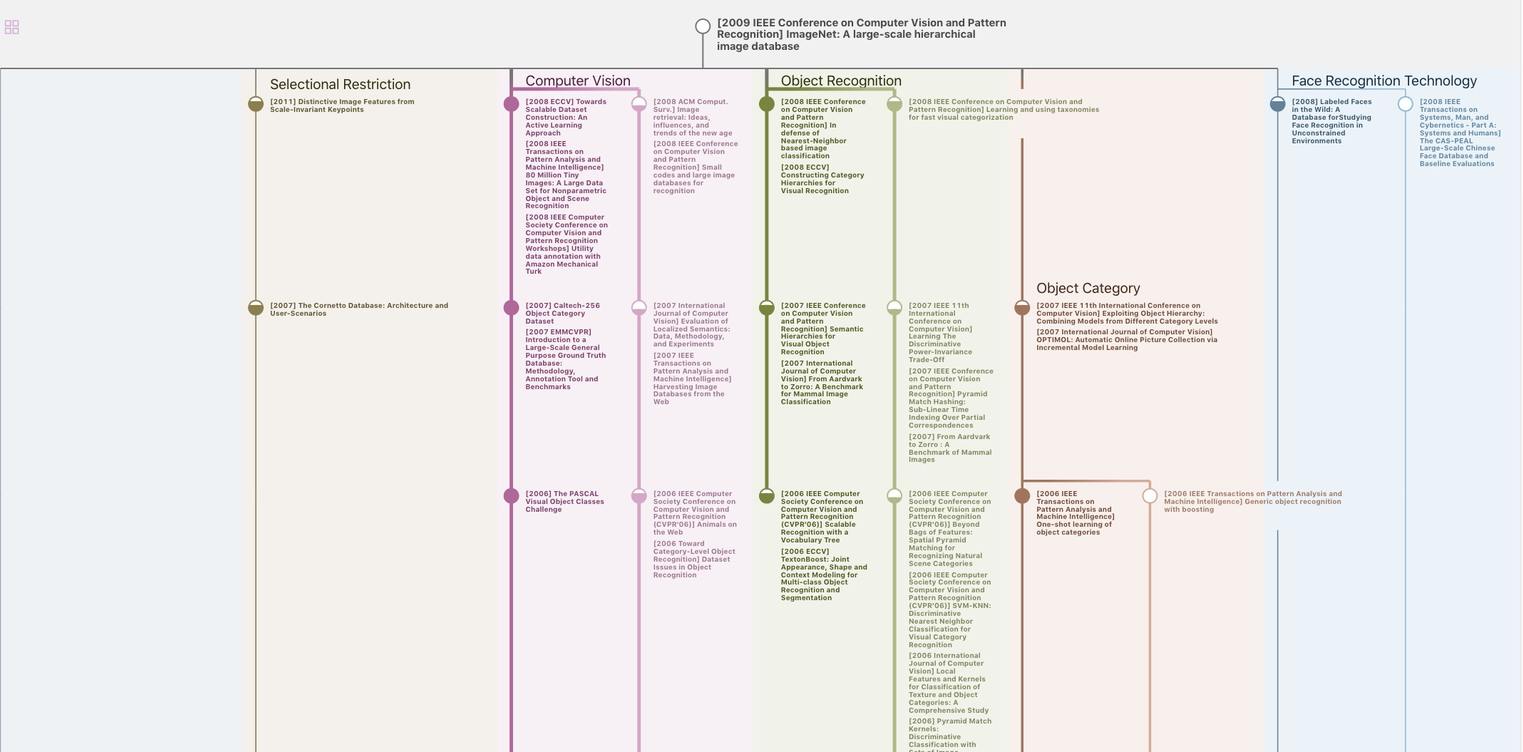
生成溯源树,研究论文发展脉络
Chat Paper
正在生成论文摘要