Machine Learning Approach for Pitch Type Classification Based on Pelvis and Trunk Kinematics Captured with Wearable Sensors
SENSORS(2023)
摘要
The large stream of data from wearable devices integrated with sports routines has changed the traditional approach to athletes' training and performance monitoring. However, one of the challenges of data-driven training is to provide actionable insights tailored to individual training optimization. In baseball, the pitching mechanics and pitch type play an essential role in pitchers' performance and injury risk management. The optimal manipulation of kinematic and temporal parameters within the kinetic chain can improve the pitcher's chances of success and discourage the batter's anticipation of a particular pitch type. Therefore, the aim of this study was to provide a machine learning approach to pitch type classification based on pelvis and trunk peak angular velocity and their separation time recorded using wearable sensors (PITCHPERFECT). The Naive Bayes algorithm showed the best performance in the binary classification task and so did Random Forest in the multiclass classification task. The accuracy of Fastball classification was 71%, whilst the accuracy of the classification of three different pitch types was 61.3%. The outcomes of this study demonstrated the potential for the utilization of wearables in baseball pitching. The automatic detection of pitch types based on pelvis and trunk kinematics may provide actionable insight into pitching performance during training for pitchers of various levels of play.
更多查看译文
关键词
baseball,pitching,wearables,classification,pitch types
AI 理解论文
溯源树
样例
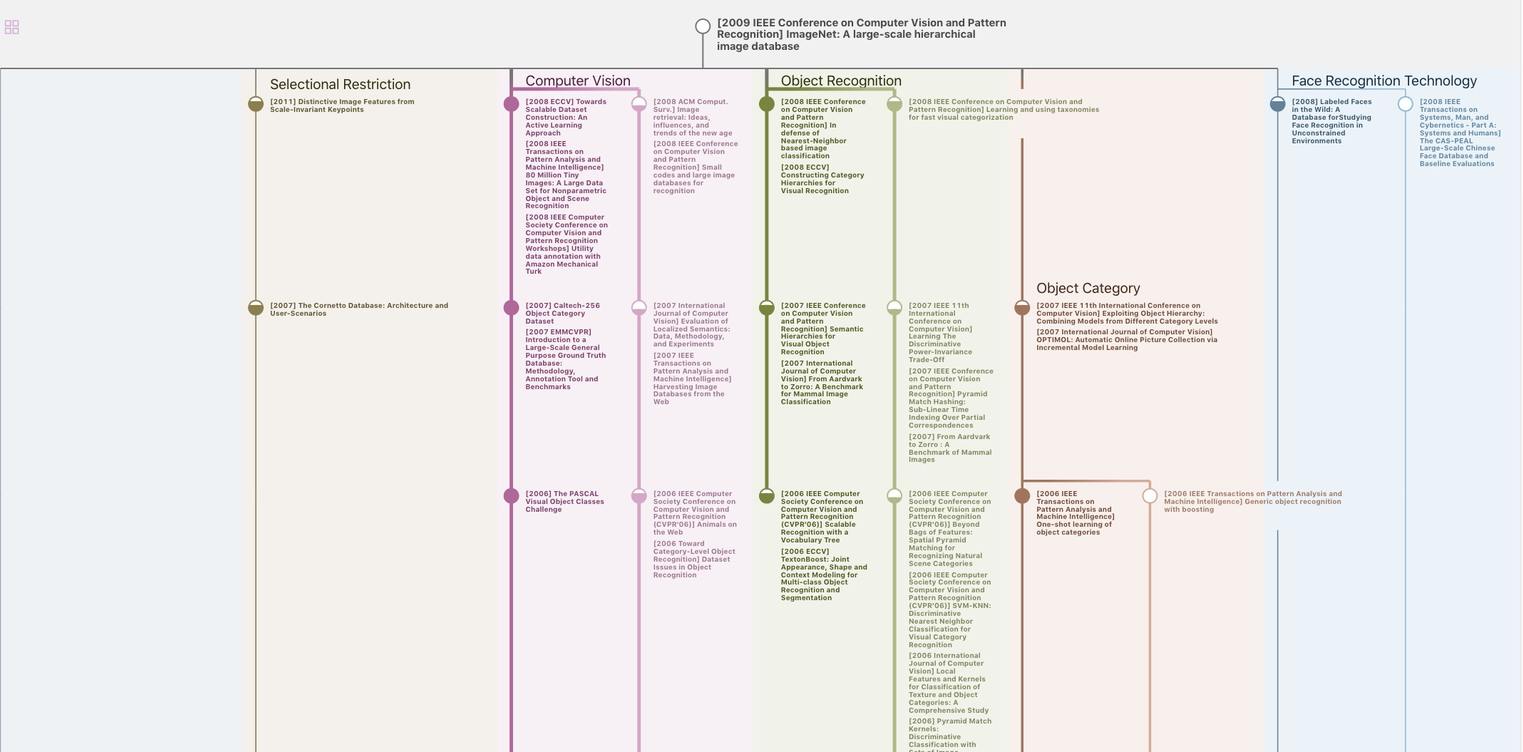
生成溯源树,研究论文发展脉络
Chat Paper
正在生成论文摘要