MVSSC: Meta-reinforcement learning based visual indoor navigation using multi-view semantic spatial context
PATTERN RECOGNITION LETTERS(2024)
摘要
In Visual Indoor Navigation (VIN), Deep Reinforcement Learning (DRL) is commonly used by agents to achieve end-to-end mapping from vision to action when navigating toward a target based on observation. However, current DRL-based work suffers from two challenges: partial observability resulting from using solely a single first-person view and poor generalization in the case of unknown scenes and unknown objects. In light of these issues, this paper introduces the integration of multi-view as an expansion of observability and meta -learning as a primary generalization technique into the DRL framework and presents the meta-reinforcement learning method that leverages Multi-View Semantic Spatial Context (MVSSC). Specifically, aiming to explore the informative multi-view context for better efficiency in searching for and navigating to the target, we model the objects' relationship from two aspects, multi-view semantic context (MVSEC) and multi-view spatial context (MVSPC). MVSEC enables agents to encode prior semantic relationships adaptively via a multi-view modulated graph. Meanwhile, MVSPC enhances the spatial representation of target-related objects' correlation through similarity grids of multi-view. After adaptively fusing the multi-view and context information under the meta -reinforcement learning framework, our method can encourage efficient target search and robust navigation with stronger generalization performance to unknown scenes and unknown objects. Extensive experimental results on the AI2-THOR simulator demonstrate that our method outperforms the current state-of-the-art approaches.
更多查看译文
关键词
Visual indoor navigation,Meta-reinforcement learning,Semantic spatial context
AI 理解论文
溯源树
样例
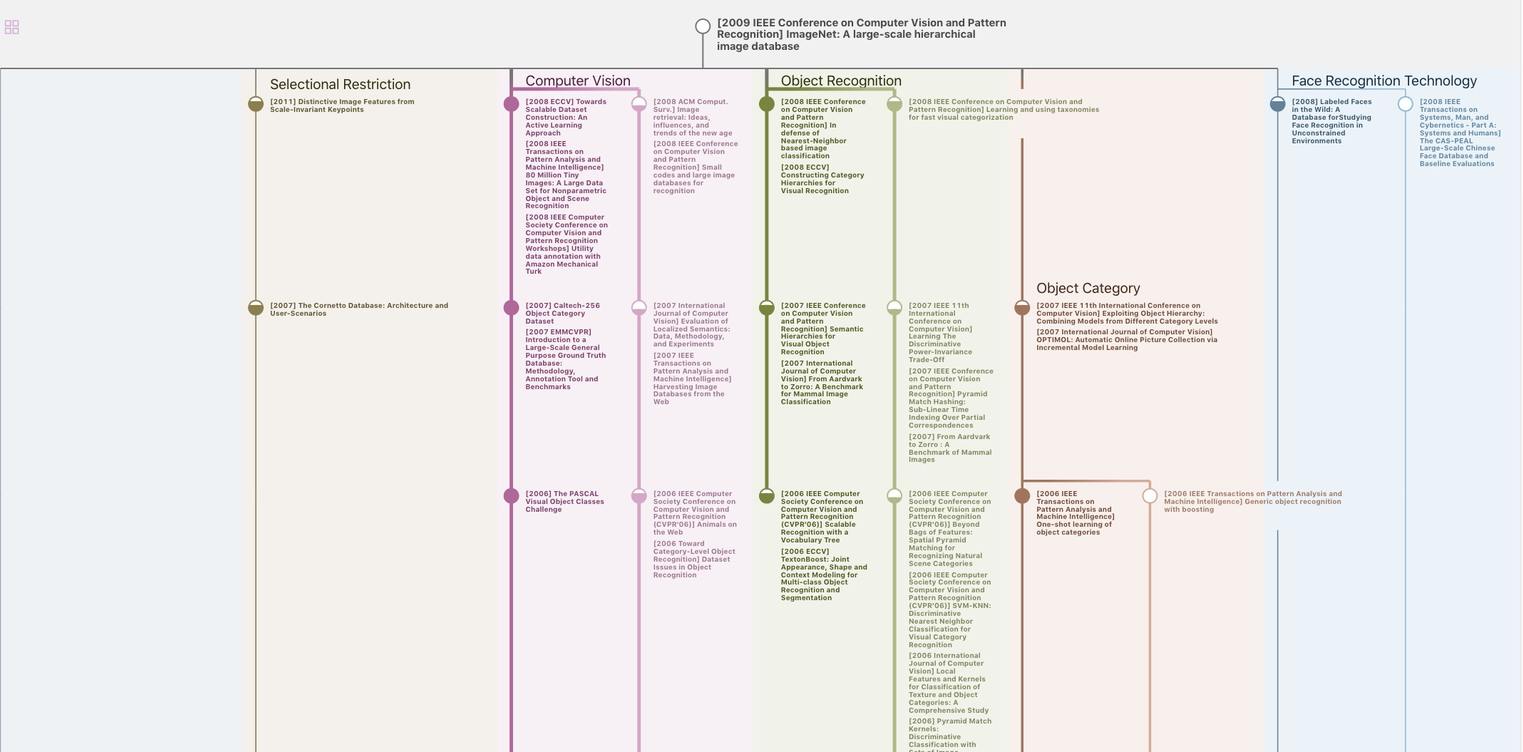
生成溯源树,研究论文发展脉络
Chat Paper
正在生成论文摘要