INNES: An intelligent network penetration testing model based on deep reinforcement learning
APPLIED INTELLIGENCE(2023)
摘要
Penetration testing (PT) is a crucial way to ensure the security of computer systems. However, it requires a high threshold and can only be implemented by trained experts. Automated tools can reduce the pressure of talent shortages, and reinforcement learning (RL) is a promising approach for achieving automated PT. Due to the unreasonable characterization of the PT process and the low efficiency of RL data, the applicability of the model is limited, and it is difficult to reuse, which hinders its practical application. In this paper, we propose an INNES ( IN telligent pe NE tration te S ting) model based on deep reinforcement learning (DRL). First, the model characterizes the key elements of PT more reasonably based on the Markov decision process (MDP), fully considering the commonality of the PT process in different scenarios to improve its applicability. Second, the DQN_valid algorithm is designed to constrain the agent’s action space, to improve the agent’s decision-making accuracy, and avoid invalid exploration, according to the feature that enables the effective action space to gradually increase during the PT process. The experimental results show that our model is not only effective for automated PT in the network environment but also has portability, which provides a possible future direction for practical application of intelligent PT based on RL.
更多查看译文
关键词
Network security assessment,Penetration testing,deep reinforcement learning,Markov decision process
AI 理解论文
溯源树
样例
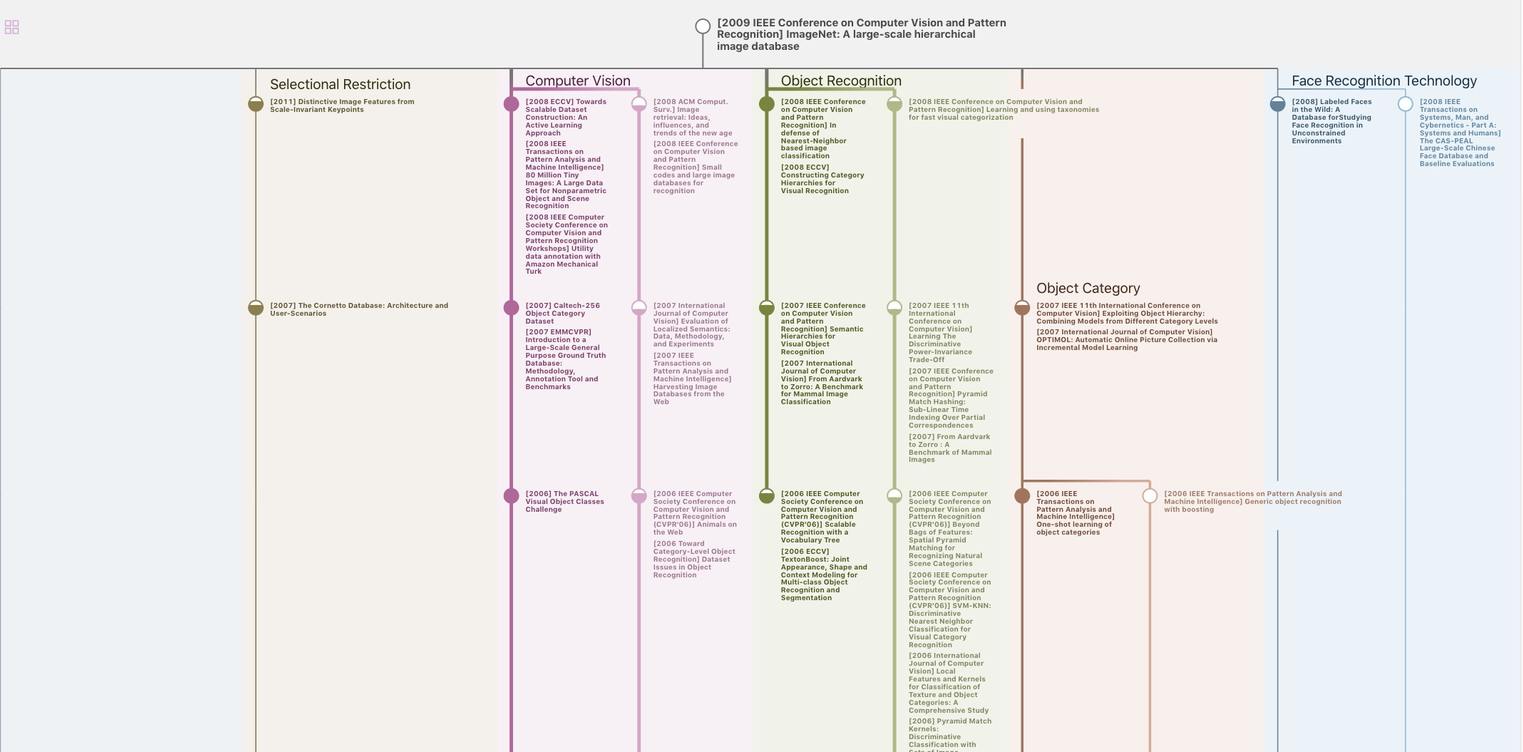
生成溯源树,研究论文发展脉络
Chat Paper
正在生成论文摘要