Deep feature representation with online convolutional adversarial autoencoder for nonlinear process monitoring
JOURNAL OF THE TAIWAN INSTITUTE OF CHEMICAL ENGINEERS(2024)
摘要
Background: The significant nonlinearity between the monitoring variables introduces challenges in the task of features extraction when implementing fault detection for an industrial process. Recently, neural network with complex hierarchical structure and layer-by-layer nonlinear transformation, especially autoencoder (AE), have attracted considerable attention from the process monitoring community. However, the latent features of AE cannot fully reflect process information, and there is redundancy between features. Methods: This study introduces an online convolutional adversarial autoencoder (AAE) model to learn nonlinear features with representative information of industrial processes. The structure of generative adversarial networks (GAN) in AAE aims to extract features that can reflect the manifold information and subject to the Gaussian distribution. Given the advantages of convolutional kernels in weight sharing and local perception, convolutional kernels are embedded in AAE to capture the spatial structure information of process data. On the basis of this model, the fault-relevant features selection strategy is designed to remove redundant information online and improve the accuracy of fault detection. Significant findings: The results show that the average fault detection rate of the penicillin fermentation process can be improved to 94% using the proposed algorithm comparing with the current fault detection methods.
更多查看译文
关键词
Nonlinear process monitoring,Adversarial autoencoder,Convolutional kernel,Penicillin fermentation process
AI 理解论文
溯源树
样例
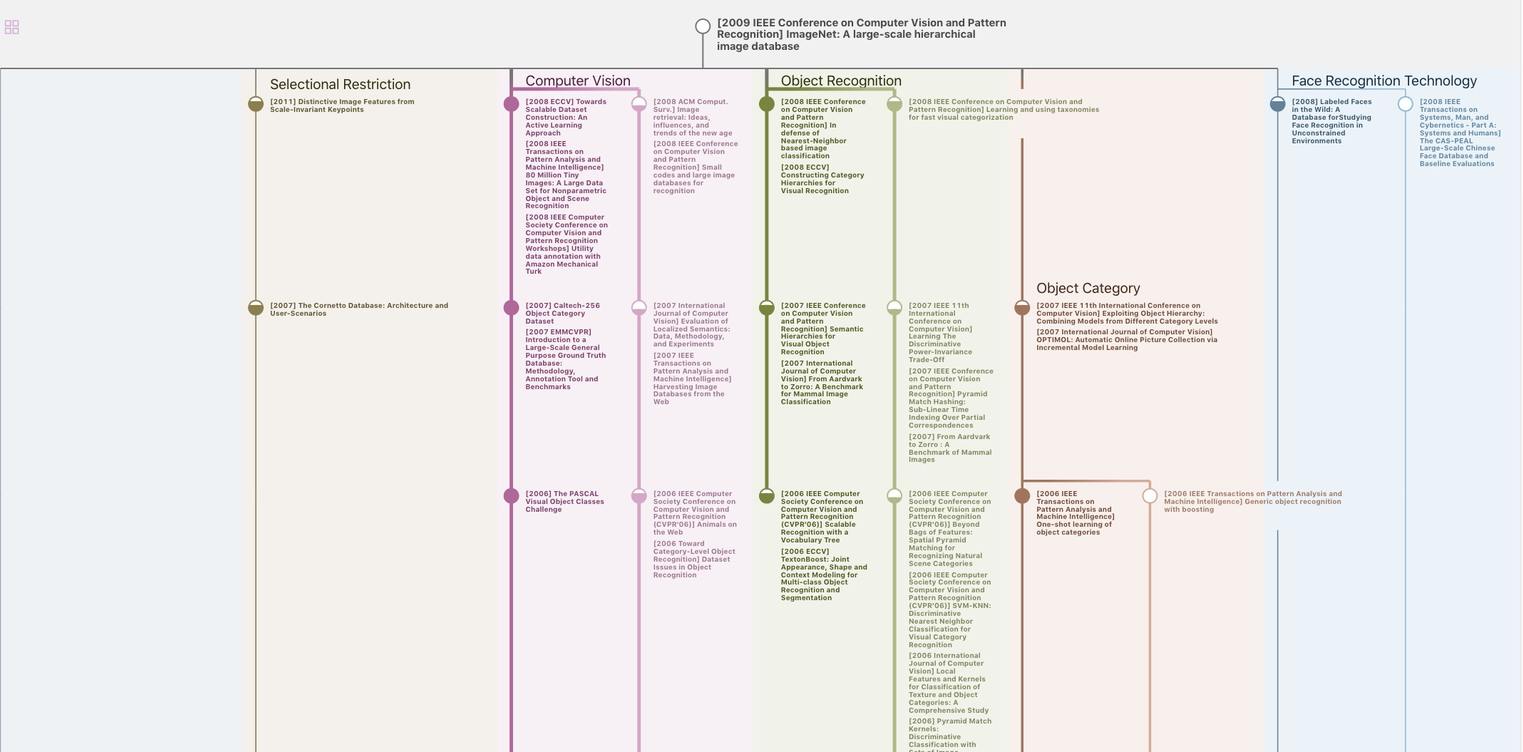
生成溯源树,研究论文发展脉络
Chat Paper
正在生成论文摘要