Observation impact metrics in NWP: A theoretical study. Part II: Systems with suboptimal observation errors
QUARTERLY JOURNAL OF THE ROYAL METEOROLOGICAL SOCIETY(2024)
摘要
Two methods are widely used to assess the impact of observations in global numerical weather prediction (NWP): data denial experiments (DDEs) and the forecast sensitivity-based observation impact (FSOI) method. A DDE measures the impact on forecast accuracy of removing an observation type from the system, whereas FSOI estimates the amount by which an observation type reduces the short-range forecast error within a system containing all observation types. This article describes the second part of a two-part study. In the first part, the theory behind DDE and FSOI metrics was presented and then applied to a simple model with two state variables, all in the context of optimal data assimilation (DA), for which the error covariances used in the DA system match reality. The article showed why and under what conditions the DDE and FSOI metrics give different results, even for an optimal DA system. In this second part, we extend the theory to suboptimal systems, and specifically to systems that are suboptimal in their specification of observation errors, and then apply it to a very simple model, in this case with one state variable. As expected, DDE impacts are reduced when the system is suboptimal. By contrast, relative FSOI impacts (i.e. relative to those of other observation types) increase for an observation type for which the errors are underestimated. This gives the erroneous impression that the change in assumed errors has led to an improvement, whereas the opposite is the case. These results provide some insights into the interpretation of FSOI results from a suboptimal DA system.
更多查看译文
关键词
data assimilation < 1,numerical weather prediction,NWP,observation impact
AI 理解论文
溯源树
样例
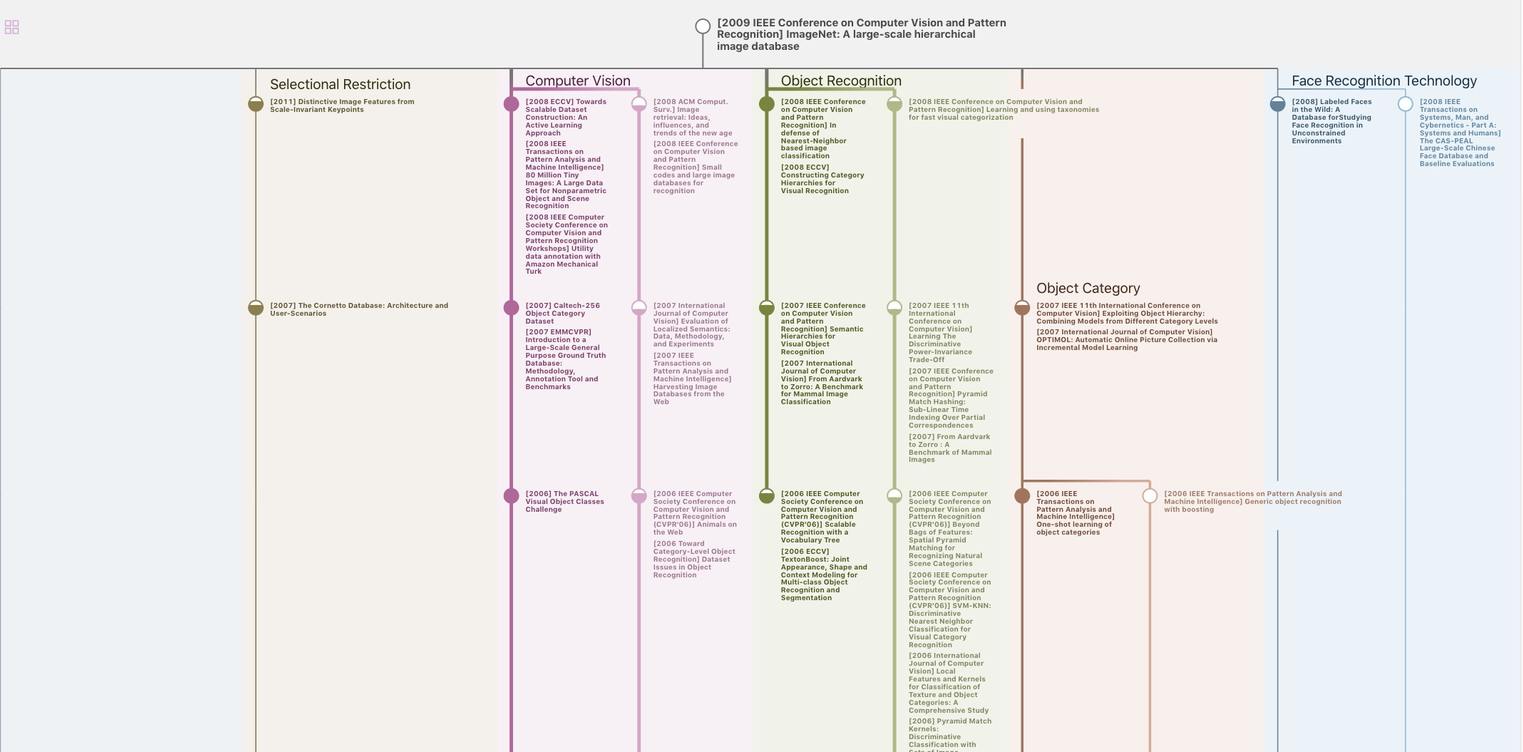
生成溯源树,研究论文发展脉络
Chat Paper
正在生成论文摘要