Elucidating genetic and environmental risk factors for antipsychotic-induced metabolic adverse effects using artificial intelligence and machine learning in the million veteran program
European Neuropsychopharmacology(2023)
摘要
Antipsychotic drugs are widely used to treat psychiatric disorders such as schizophrenia and bipolar disorder. However, they are known to have important adverse effects related to metabolic syndrome (MS), which significantly impacts their effectiveness. Quantifying this effect is complicated because the temporal patterns of both antipsychotic medication usage and MS-related outcomes vary across patients. To minimize deleterious effects on patients, it is of the utmost importance to characterize and predict MS effects using genetic and non-genetic information available in electronic medical record (EmR) datasets. For our initial analysis, we aggregate patient's EMR data into three-month intervals, and perform model selection and linear regression to identify important covariates such as age, sex, comorbid medical conditions, oral hypoglycemic, statin, and metformin usage, and hospitalization. We further develop a longitudinal deep learning model that uses high dimensional EMR data to predict MSvar trajectories and utilize SHAP (Shapely additive explanations) values to identify both temporal and polypharmacy-related patterns, predictive of adverse metabolic effects. In addition, we utilize highly predictive phenotypes developed on the full cohort of VA patients to perform GWAS for the rate of change in MS outcomes as a function of SNP genotype in the MVP population (currently 658,582 patients with genomic profiles) and antipsychotic type, while adjusting for 20 ancestry principal components and other biologically relevant covariates. Subsequently, we use summary statistics from GWAS to perform transcriptome/proteome/methylome wide analyses, at both gene and gene set levels, employing state-of-the-art mendelian randomization tools. Our cohort was selected on the basis of antipsychotic medication usage and completeness of the characterization of our MS outcomes, from all 25 million patients with records in the VA EMR system, in use between 2000 to 2023. This results in a combined 12,303,200 patient-quarters of observation among 135,200 unique patients who have had at least one hundred measurements of weight and ten antipsychotic prescription fills. We find in a multivariate logistic regression that Quetiapine, Risperidone, Olanzapine, and Aripiprazole were associated with weight gain and Haloperidol, Ziprasidone, and Fluphenazine with weight loss in quarters (three month periods) when each medication was prescribed at least once, compared to quarters without the medication. This was when controlling for Anxiety and Bipolar diagnoses (associated with weight gain), as well as diabetes medications (metformin associated with weight loss, while insulin with weight gain) and statins, which were associated with weight gain. We further observed a strong impact of age on these associations, with diabetes medications and statins relatively more important in older patients and the antipsychotic medications with the young. GWAS results will be presented. Several second-generation antipsychotics are associated with weight gain, while two first-generation antipsychotics, as well as ziprasidone, are associated with weight loss. These are age-dependent and impacted by both psychiatric diagnoses as well as insulin, statin, and metformin use. GWAS results will be discussed.
更多查看译文
关键词
million veteran program,elucidating genetic,adverse effects,antipsychotic-induced
AI 理解论文
溯源树
样例
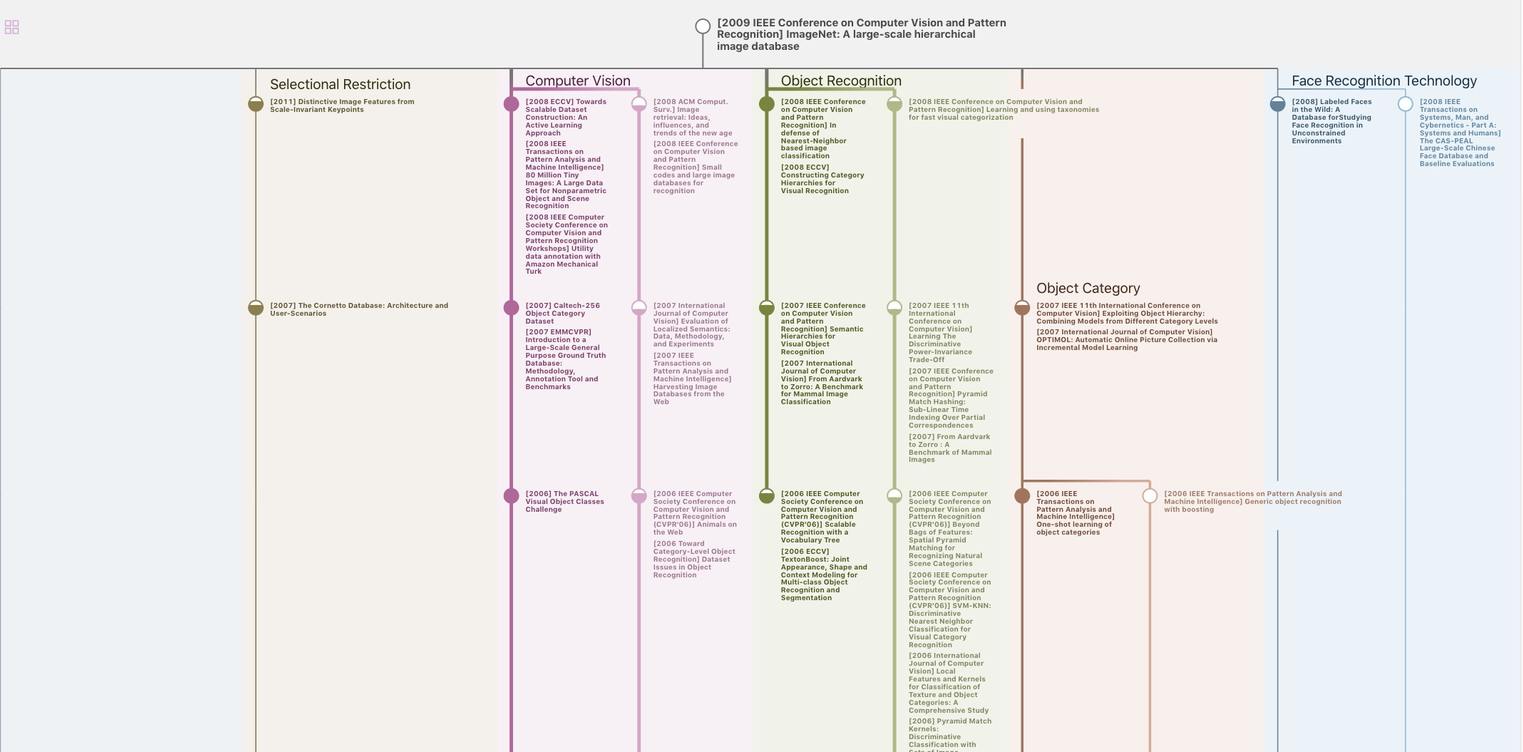
生成溯源树,研究论文发展脉络
Chat Paper
正在生成论文摘要