MMLF: Multi-Metric Latent Feature Analysis for High-Dimensional and Incomplete Data
IEEE TRANSACTIONS ON SERVICES COMPUTING(2024)
摘要
High-dimensional and incomplete (HDI) data are omnipresent in a variety of Big Data-related applications. Latent feature analysis (LFA) is a typical representation learning method that can extract useful yet latent knowledge from HDI data via low-rank embedding. Existing LFA-based models mostly adopt a single-metric-based modeling strategy, where the representation designed for the embedding Loss function is fixed and exclusive. However, real-world HDI data are commonly heterogeneous and have large diverse underlying patterns, making a single-metric-based model cannot represent such HDI data in a comprehensive and unbiased fashion. Motivated by this discovery, this article proposes a multi-metric latent feature (MMLF) model whose ideas are two-fold: 1) two vector spaces and three L-p-norms are simultaneously adopted to develop six LFA variants, each of which possesses a unique merit, and 2) all the variants are aggregated with a tailored, self-adaptive weighting strategy. As such, the proposed MMLF enjoys the merits originated from a set of disparate metric spaces all at once, achieving the comprehensive and unbiased representation of HDI data. Theoretical study guarantees that MMLF attains evident performance gain. Extensive experiments on ten real-world HDI matrices, spanning a wide range of industrial and scientific areas, verify that the proposed MMLF significantly outperforms nine state-of-the-art, shallow and deep counterparts.
更多查看译文
关键词
Online services,high-dimensional and incomplete data,latent feature analysis,representation learning,representation metric,multi-metric modeling
AI 理解论文
溯源树
样例
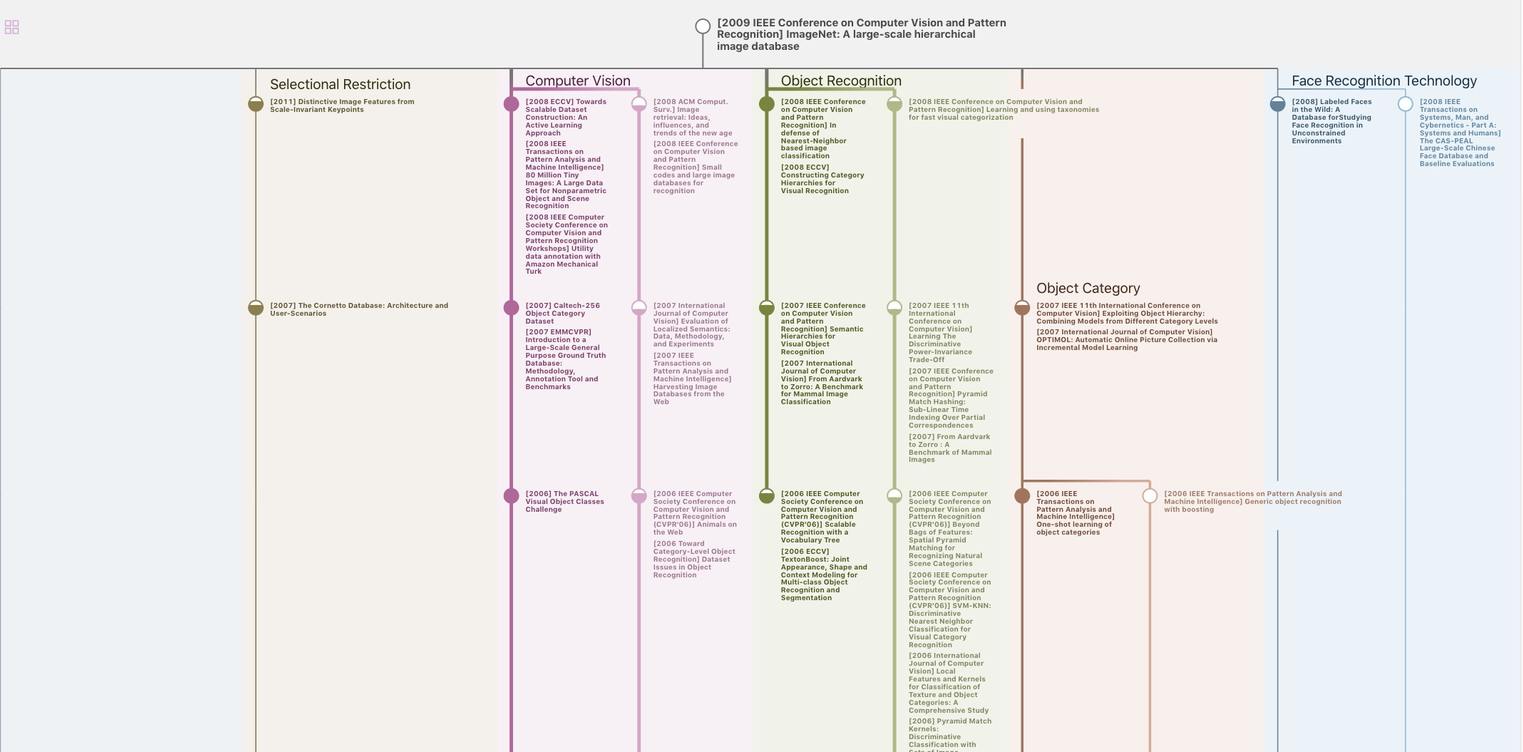
生成溯源树,研究论文发展脉络
Chat Paper
正在生成论文摘要