Composition prediction of pore solution in hardened concrete materials based on machine learning
DEVELOPMENTS IN THE BUILT ENVIRONMENT(2023)
摘要
The pore solution composition (OH , Na+, K+, Ca2+ and SO42-, S2O32-, S2- concentrations) of hardened concrete materials, including binary systems of PC mixed with a single SCM and with two SCMs, was investigated. Based on database comprising more than 400 entries with more than 80 parameters, machine learning (ML) is applied to predict ion concentrations. Catboost model is the optimal model. The concentrations of OH , Na+, K+ were predicted with high accuracy (R2 of 0.92-0.95). The prediction accuracy of S is low, could also reaches a R2 of 0.79. But the prediction accuracy of linear regression model is very low, with R2 of 0.18-0.7. PC_MgO, PC_Na2O, PC_K2O and SCM_SiO2 rank high in the characteristic importance analysis for predicting the concentration of OH , Na+, K+. Compared with the classical pore solution prediction methods (Taylor's and NIST algorithm), the ML model is more accurate. Due to the use of more data and kinds of methods, the ML prediction results in this paper are also better than Cristhiana's ML model. These ML models can be used to predict pore solution of more than 28 d old normal PC concrete with silica fume, fly ash, slag, limestone or quartz powder, but PC or SCM with high phosphorus oxide is not suitable.
更多查看译文
关键词
Composition prediction,Pore solution,Machine learning,Catboost
AI 理解论文
溯源树
样例
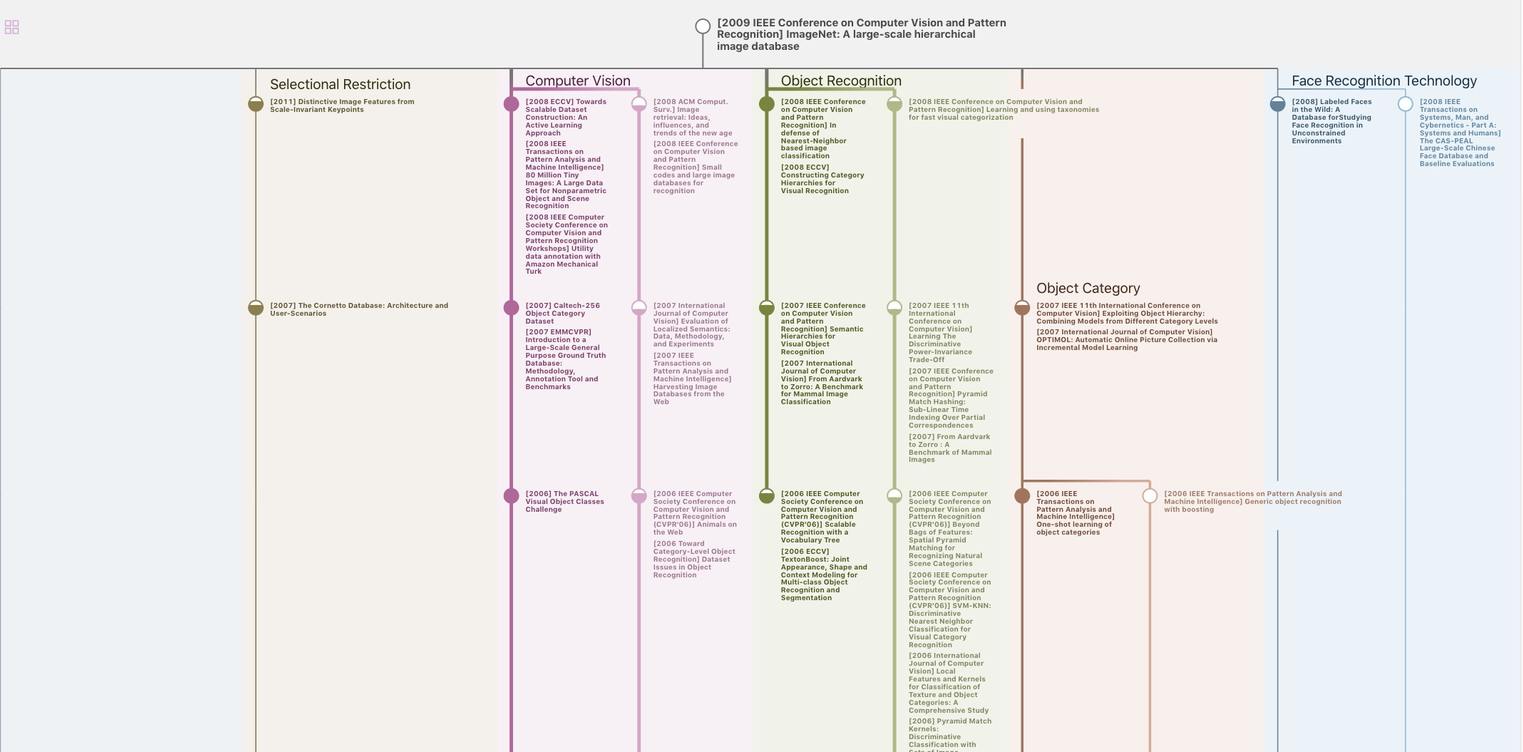
生成溯源树,研究论文发展脉络
Chat Paper
正在生成论文摘要