Using Less but Important Information for Feature Distillation
NEURAL INFORMATION PROCESSING, ICONIP 2023, PT I(2024)
摘要
The purpose of feature distillation is that using the teacher network to supervise student network so that the student network can mimic the intermediate layer representation of the teacher network. The most intuitive way of feature distillation is to use the Mean-Square Error (MSE) to optimize the distance of feature representation at the same level for both networks. However, one problem in feature distillation is that the dimension of the intermediate layer feature maps of the student network may be different from that of the teacher network. Previous work mostly elaborated a projector to transform feature maps to the same dimension. In this paper, we proposed a simple and straightforward feature distillation method without additional projector to adapt the feature dimension inconsistency between the teacher and the student networks. We consider the redundancy of the data and show that it is not necessary to use all the information when performing feature distillation. In detail, we propose a cut-off operation for channel alignment and use singular value decomposition (SVD) for knowledge alignment so that only important information is transferred to the student network to solve the dimension inconsistency problem. Extensive experiments on several different models show that our method can improve the performance of student networks.
更多查看译文
关键词
Neural network,Knowledge distillation,Model compression
AI 理解论文
溯源树
样例
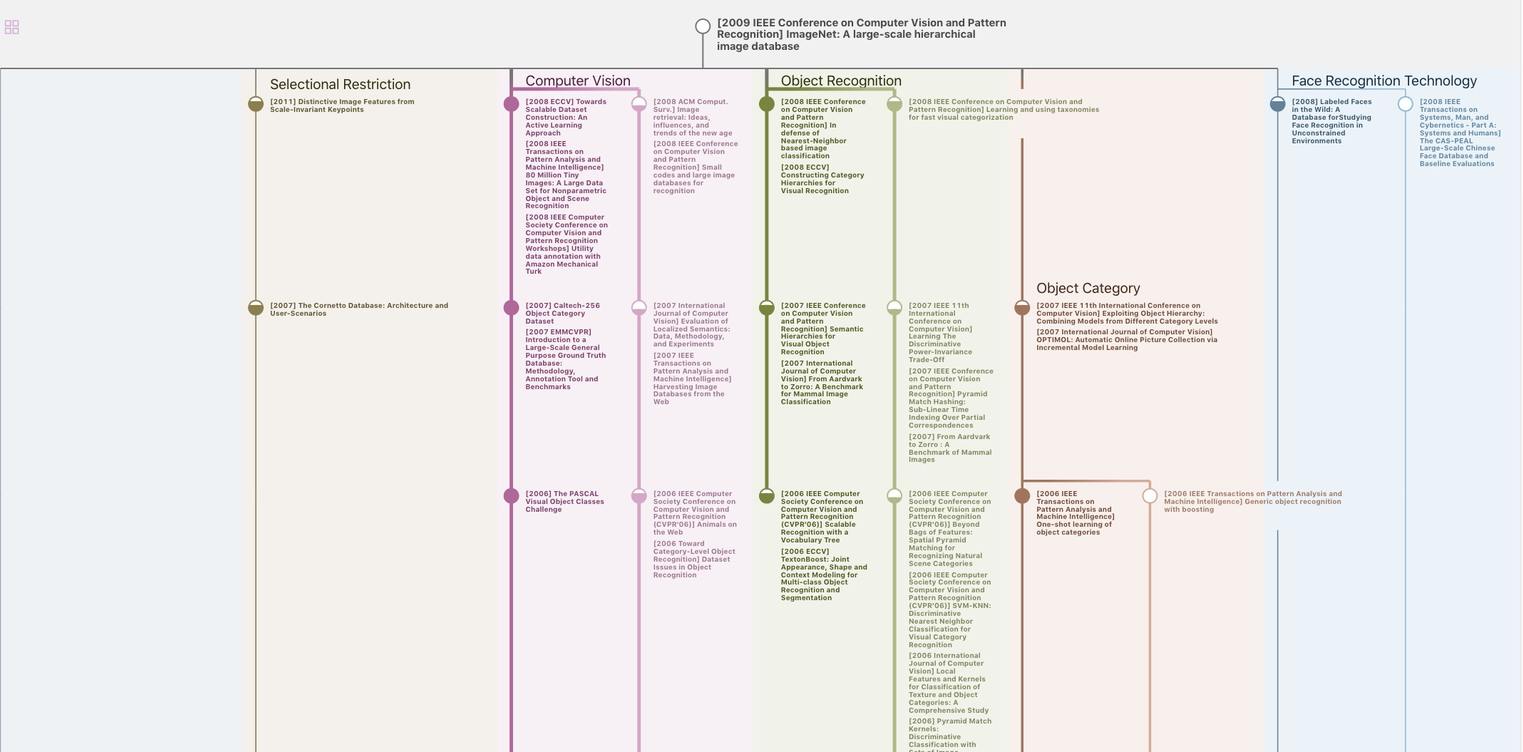
生成溯源树,研究论文发展脉络
Chat Paper
正在生成论文摘要