Visual Explainable Machine Learning for High-Stakes Decision-Making with Worst Case Estimates
Data Analysis and Optimization Springer Optimization and Its Applications(2023)
摘要
A major motivation for explaining and rigorous evaluating Machine Learning (ML) models is coming from high-stake decision-making tasks like cancer diagnostics, self-driving cars, and others with possible catastrophic consequences of wrong decisions. This chapter shows that visual knowledge discovery (VKD) methods, based on the General Line Coordinates (GLC) recently developed, can significantly contribute to solving this problem. The concept of hyperblocks (n-D rectangles) as interpretable dataset units and GLC are combined to create visual self-service machine learning models. Two variants of Dynamic Scaffold Coordinates (DSC) are proposed. It allows losslessly mapping high-dimensional datasets to a single two-dimensional Cartesian plane and building interactively an ML predictive model in this 2-D visualization space. Major benefits of DSC1 and DSC2 is their highly interpretable nature. They allow domain experts to control or establish new machine learning models through visual pattern discovery. It opens a visually appealing opportunity for domain experts, who are not ML experts, to build ML models as a self-service bringing the domain expertise to the model discovery, which increases model explainability and trust for the end user. DSC were used to find, visualize, and estimate the worst-case validation splits in several benchmark datasets, which is important for high-risk application. For large datasets DSC is combined with dimensionality reduction techniques such as principal component analysis, singular value decomposition, and t-distributed stochastic neighbor embedding. A software package referred to as Dynamic Scaffold Coordinates Visualization System (DSCViz) was created to showcase the DSC1 and DSC2 systems.
更多查看译文
关键词
visual explainable machine learning,decision-making decision-making,estimates,high-stakes
AI 理解论文
溯源树
样例
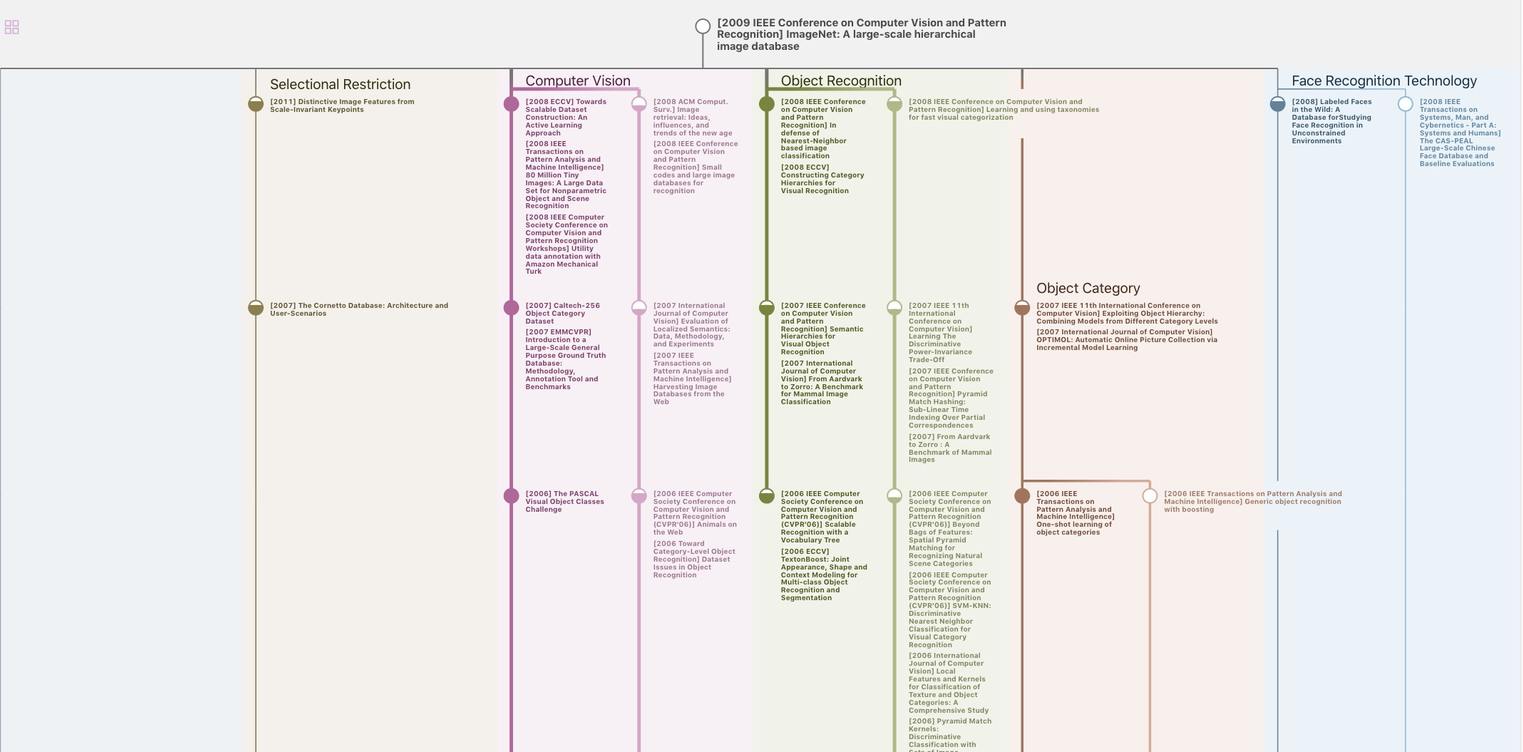
生成溯源树,研究论文发展脉络
Chat Paper
正在生成论文摘要