Aggregation Strategies of Wav2vec 2.0 Embeddings for Computational Paralinguistic Tasks
SPEECH AND COMPUTER, SPECOM 2023, PT I(2023)
摘要
Throughout the history of computational paralinguistics, numerous feature extraction, preprocessing and classification techniques have been used. One of the important challenges in this subfield of speech technology is handling utterances with different duration. Since standard speech processing features (such as filter banks or DNN embeddings) are typically frame-level ones and we would like to classify whole utterances, a set of frame-level features have to be converted into fixed-sized utterance-level features. The choice of this aggregation method is often overlooked, and simple functions like mean and/or standard deviation are used without solid experimental support. In this study we take wav2vec 2.0 deep embeddings, and aggregate them with 11 different functions. We sought to obtain a subset of potentially optimal aggregation functions, because there are no general rules yet that can be applied universally between subtopics. Besides testing both standard and non-traditional aggregation strategies individually, we also combined them to improve the classification performance. By using multiple aggregation functions, we were able to achieve significant improvements on three public paralinguistic corpora.
更多查看译文
关键词
Paralinguistics,Wav2vec 2.0,Embeddings,Aggregation
AI 理解论文
溯源树
样例
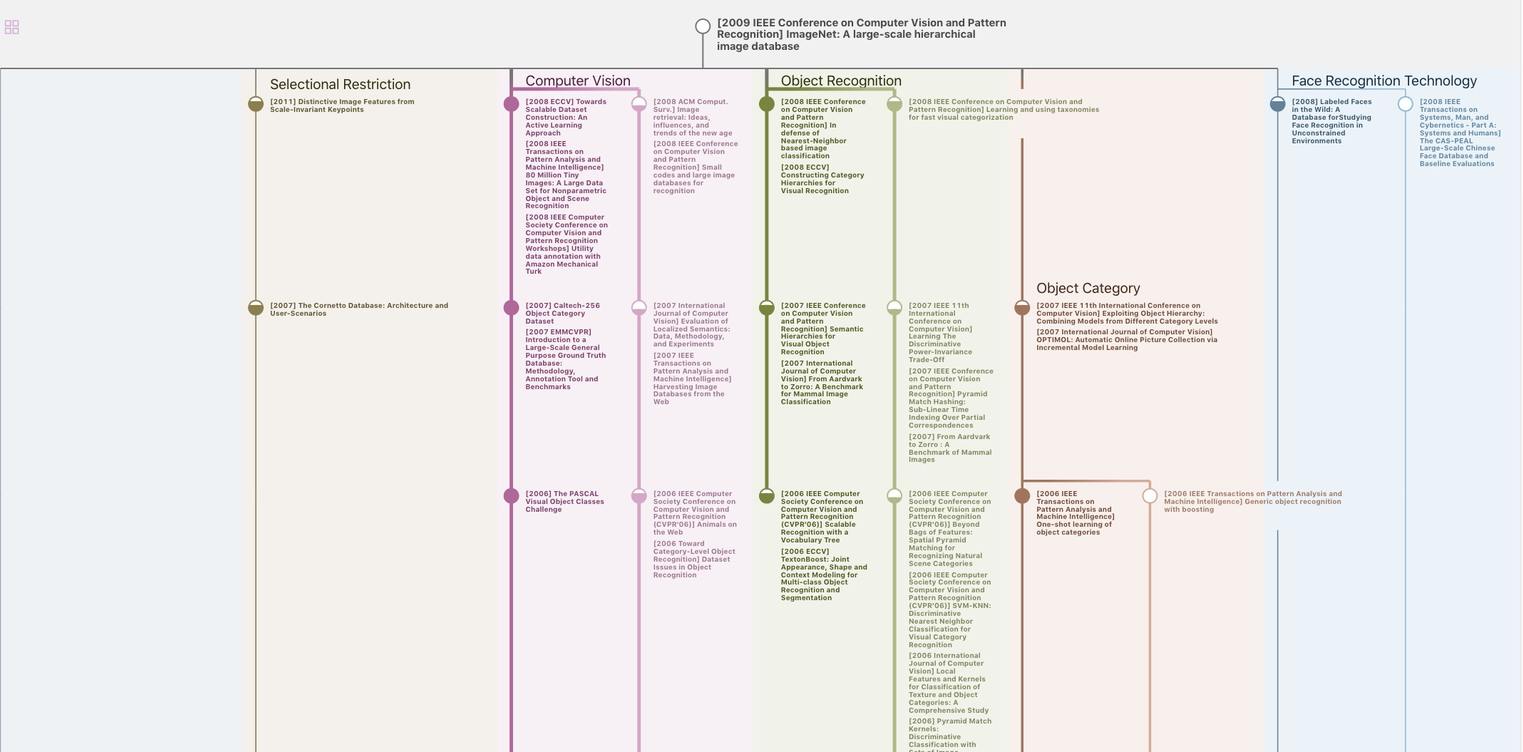
生成溯源树,研究论文发展脉络
Chat Paper
正在生成论文摘要