Supervised Representation Learning for Network Traffic With Cluster Compression
IEEE TRANSACTIONS ON SUSTAINABLE COMPUTING(2024)
摘要
In the face of increasing network traffic, network security issues have gained significant attention. Existing network intrusion detection models often improve the ability to distinguish network behaviors by optimizing the model structure, while ignoring the expressiveness of network traffic at the data level. Visual analysis of network behavior through representation learning can provide a new perspective for network intrusion detection. Unfortunately, representation learning based on machine learning and deep learning often suffer from scalability and interpretability limitations. In this article, we establish an interpretable multi-layer mapping model to enhance the expressiveness of network traffic data. Moreover, the unsupervised method is used to extract the internal distribution characteristics of the data before the model to enhance the data. What's more, we analyze the feasibility of the proposed flow spectrum theory on the UNSW-NB15 dataset. Experimental results demonstrate that the flow spectrum exhibits significant advantages in characterizing network behavior compared to the original network traffic features, underscoring its practical application value. Finally, we conduct an application analysis using multiple datasets (CICIDS2017 and CICIDS2018), revealing the model's strong universality and adaptability across different datasets.
更多查看译文
关键词
Cluster,data compression,network traffic,representation learning,spatial mapping
AI 理解论文
溯源树
样例
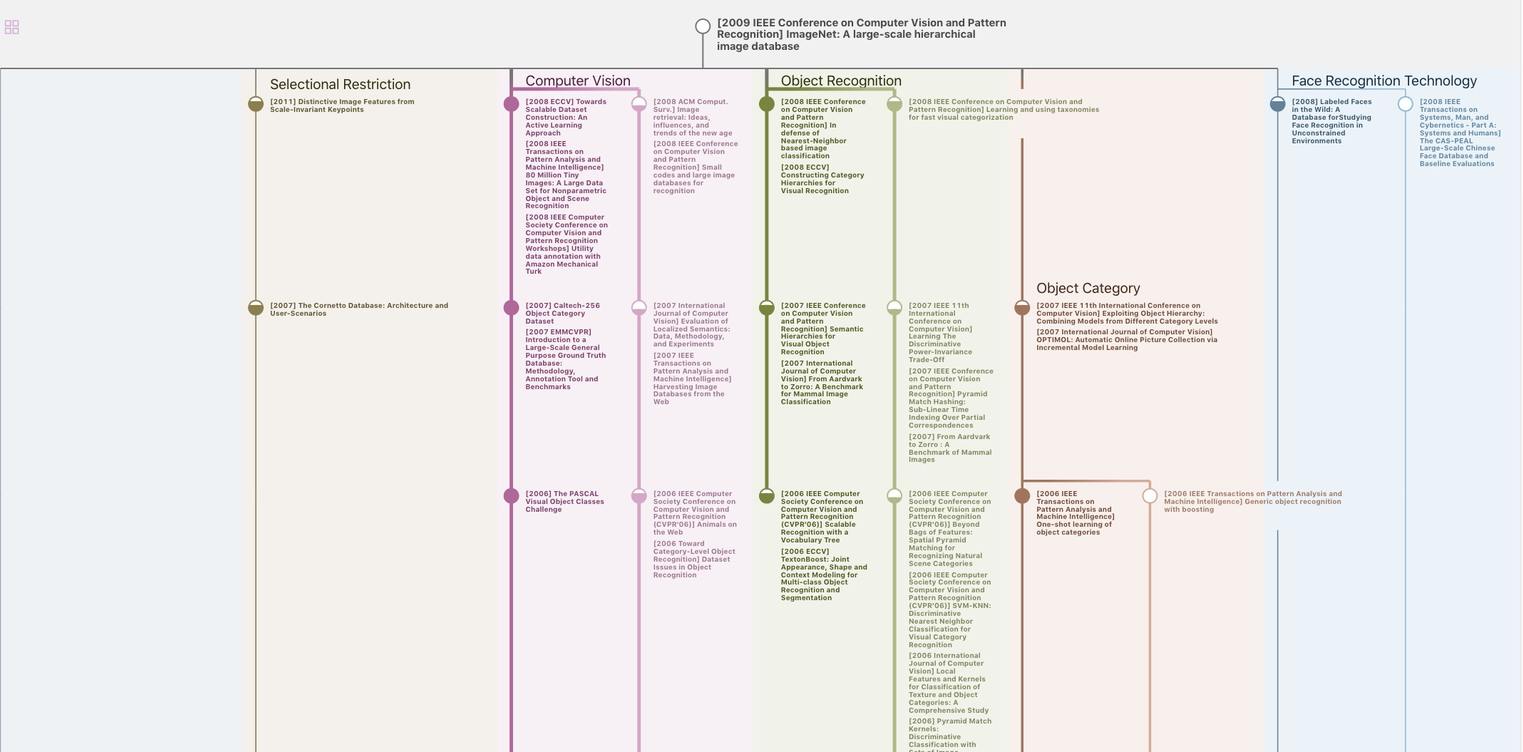
生成溯源树,研究论文发展脉络
Chat Paper
正在生成论文摘要