Refined Defect Detector With Deformable Transformer and Pyramid Feature Fusion for PCB Detection
IEEE TRANSACTIONS ON INSTRUMENTATION AND MEASUREMENT(2024)
摘要
Printed circuit board (PCB) defect detection is a part of the quality control process, which detects and identifies predamage in finished products. However, it is difficult to detect them due to small defects. To this end, this article designs a refined defect detector (RDTor) with deformable transformer and pyramid feature fusion to precisely capture small defects for achieving the defect detection of PCB, where RDTor is comprised of three parts: multihead nonlocal transformer (MNT) module, multiscale pyramid feature fusion (MPFF) module, and adaptive defect detection (ADD) ones. Specifically, an MNT module is first developed to adaptively focus on the defect areas for highlighting defect features and suppressing nondefect background ones, an MPFF module is proposed to preserve the features of small defects much more as network deepening, and an ADD module is presented to adaptively perform the defect inspection for obtaining defect categories and defect prediction box. The experimental results on a large-scale PCB image dataset acquired from real-world industrial products show that the proposed method achieves the state-of-the-art accuracy in industrial applications, where it can achieve 99.6% (accuracy), 97.2% (precision), 98.8% (recall), 98.0% (F1-score), and 99.3% mean Average Precision (mAP) in terms of multiscale defects classification and detection results.
更多查看译文
关键词
Deep learning,deformable transformer,printed circuit board (PCB) defect detection,pyramid feature fusion
AI 理解论文
溯源树
样例
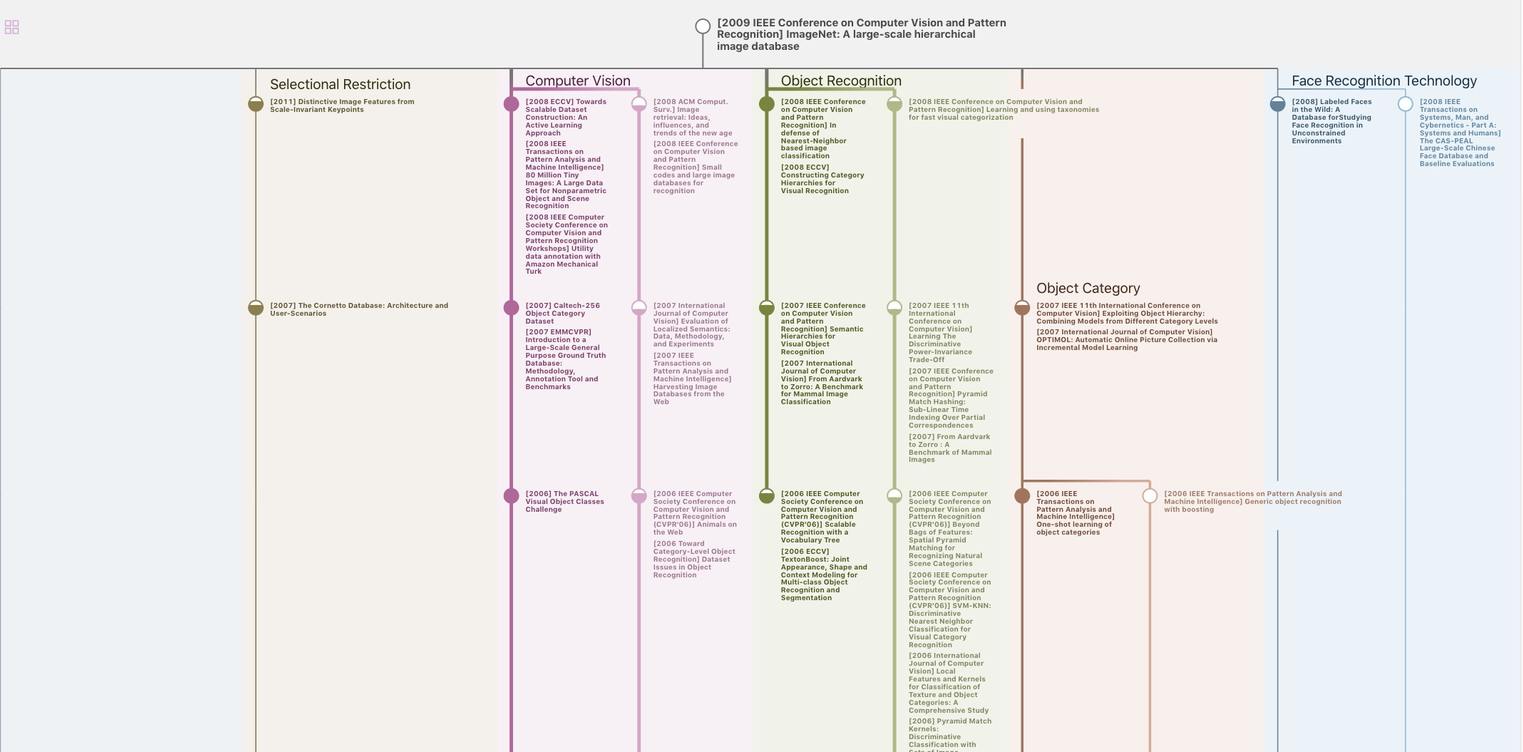
生成溯源树,研究论文发展脉络
Chat Paper
正在生成论文摘要