Machine learning for amniotic fluid volume prediction
ULTRASOUND IN OBSTETRICS & GYNECOLOGY(2023)
摘要
To present an objective and automated methodology of uncertainty quantification in medical segmentation tasks, with a focus on amniotic fluid (AF) segmentation and volume estimation. We used machine learning tools to obtain the segmentation, and then apply conformal prediction methods to quantify the uncertainty in the results of our algorithm. We retrospectively evaluate 652 fetal magnetic resonance imaging (MRI) with gestational age between 19 to 38 weeks. MRI was performed using a 1.5-Tesla magnet (Aera, Siemens Healthcare, Erlangen, Germany). We applied a 3D, T2-weighted true fast imaging sequence with steady-state precession (true FISP) in the sagittal plane (TR = 3.02 ms; TE = 1.43 ms; isotropic voxel = 1.0 × 1.0 × 1.0 mm3; matrix = 256 × 256 mm; 136 slices), acquisition time of 26 s. The uterine contents were segmented under supervision of the radiologist, and the AF was highlighted. We obtained an algorithm that can effectively segment AF from MRI, obtaining a Dice coefficient of 0.924 ± 0.06 in a test set with 112 exams, indicating widespread agreement between medical experts and the machine learning algorithm. The algorithm is based on a U-net neural network architecture with over 17 million parameters and trained with the binary cross-entropy loss over 420 exams, each with about 256 × 256 × 160 voxels. Of the few errors incurred by the network, most are concentrated on the border of the segmentation, where medical experts are also expected to disagree on the order of a few voxels. Neural networks can be applied effectively and help fetal medicine specialists to ascertain AF volume quickly and accurately. Please note: The publisher is not responsible for the content or functionality of any supporting information supplied by the authors. Any queries (other than missing content) should be directed to the corresponding author for the article.
更多查看译文
关键词
volume,machine learning,fluid,prediction
AI 理解论文
溯源树
样例
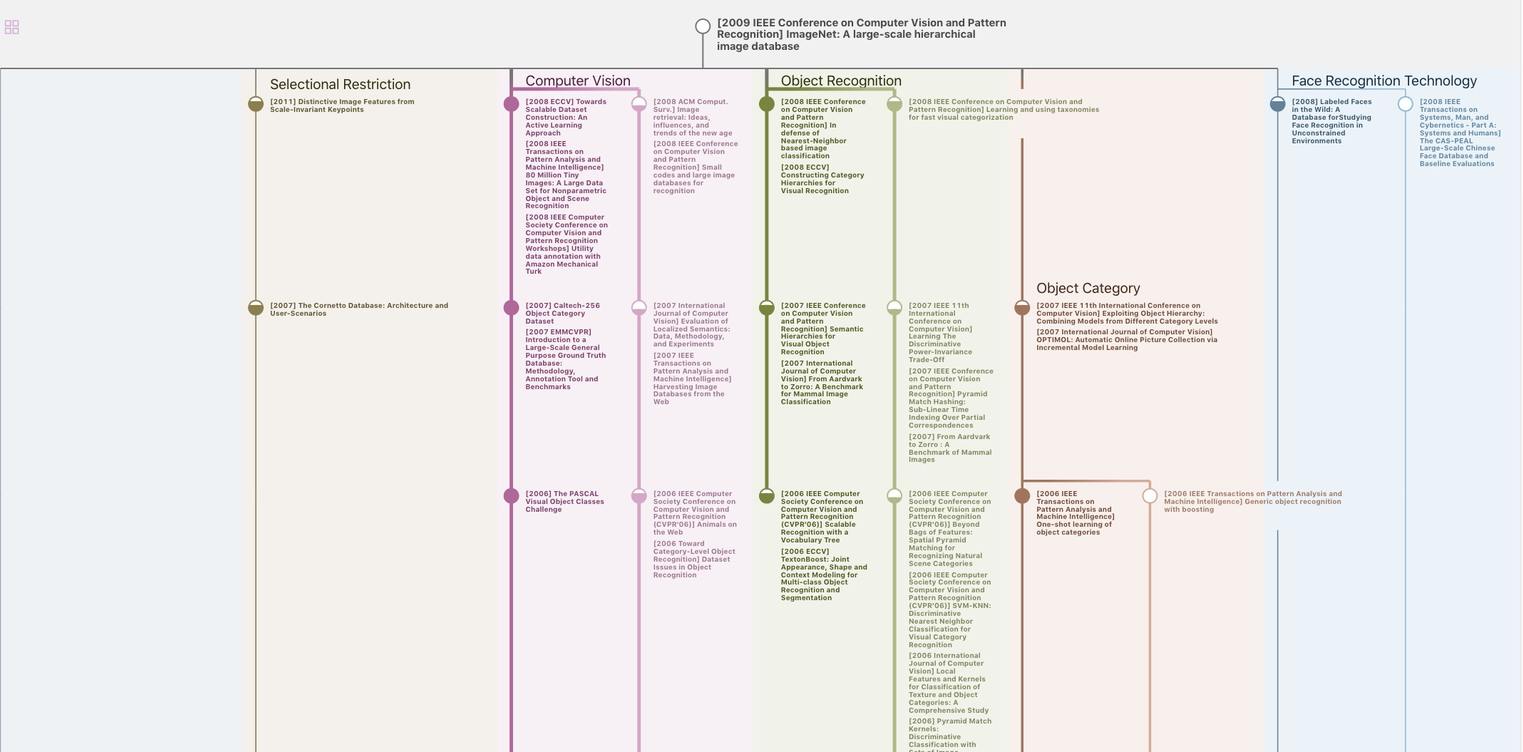
生成溯源树,研究论文发展脉络
Chat Paper
正在生成论文摘要