Towards Scalable Multi-View Clustering via Joint Learning of Many Bipartite Graphs
IEEE TRANSACTIONS ON BIG DATA(2024)
摘要
This paper focuses on two limitations to previous multi-view clustering approaches. First, they frequently suffer from quadratic or cubic computational complexity, which restricts their feasibility for large-scale datasets. Second, they often rely on a single graph on each view, yet lack the ability to jointly explore many versatile graph structures for enhanced multi-view information exploration. In light of this, this paper presents a new Scalable Multi-view Clustering via Many Bipartite graphs (SMCMB) approach, which is capable of jointly learning and fusing many bipartite graphs from multiple views while maintaining high efficiency for very large-scale datasets. Different from the one-anchor-set-per-view paradigm, we first produce multiple diversified anchor sets on each view and thus obtain many anchor sets on multiple views, based on which the anchor-based subspace representation learning is enforced and many bipartite graphs are simultaneously learned. Then these bipartite graphs are efficiently partitioned to produce the base clusterings, which are further re-formulated into a unified bipartite graph for the final clustering. Note that SMCMB has almost linear time and space complexity. Extensive experiments on twenty general-scale and large-scale multi-view datasets confirm its superiority in scalability and robustness over the state-of-the-art.
更多查看译文
关键词
Bipartite graph learning,ensemble clustering,large-scale clustering,large-scale data,multi-view clustering
AI 理解论文
溯源树
样例
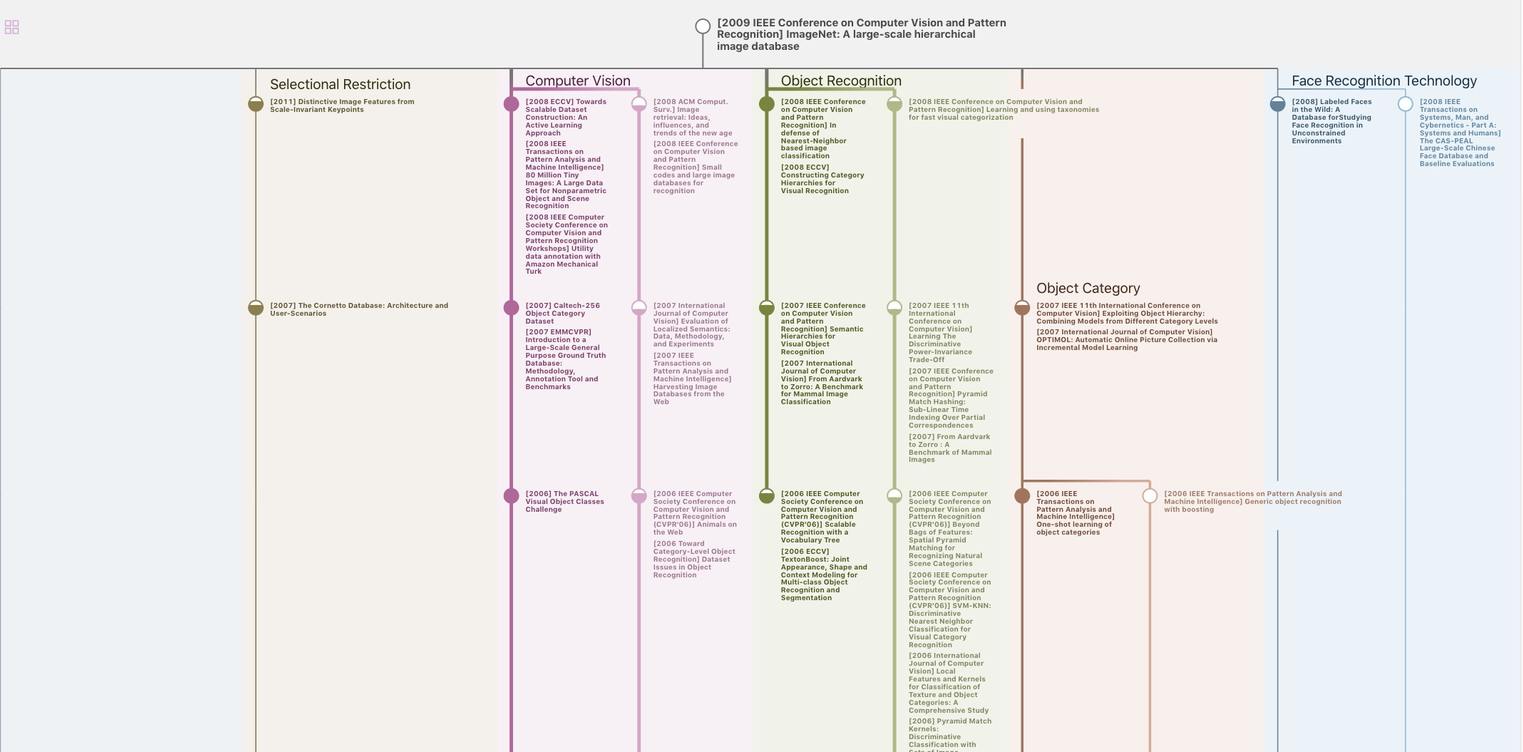
生成溯源树,研究论文发展脉络
Chat Paper
正在生成论文摘要