Transfer Learning-Based Feature Fusion Model for Classification of Maneuver Weapon Systems
J. Inf. Process. Syst.(2023)
摘要
Convolutional neural network-based deep learning technology is the most commonly used in image identification, but it requires large-scale data for training. Therefore, application in specific fields in which data acquisition is limited, such as in the military, may be challenging. In particular, the identification of ground weapon systems is a very important mission, and high identification accuracy is required. Accordingly, various studies have been conducted to achieve high performance using small-scale data. Among them, the ensemble method, which achieves excellent performance through the prediction average of the pre-trained models, is the most representative method; however, it requires considerable time and effort to find the optimal combination of ensemble models. In addition, there is a performance limitation in the prediction results obtained by using an ensemble method. Furthermore, it is difficult to obtain the ensemble effect using models with imbalanced classification accuracies. In this paper, we propose a transfer learning-based feature fusion technique for heterogeneous models that extracts and fuses features of pre-trained heterogeneous models and finally, fine-tunes hyperparameters of the fully connected layer to improve the classification accuracy. The experimental results of this study indicate that it is possible to overcome the limitations of the existing ensemble methods by improving the classification accuracy through feature fusion between heterogeneous models based on transfer learning.
更多查看译文
关键词
Artificial Intelligence,Deep Learning,Ensemble,Feature Fusion,Transfer Learning
AI 理解论文
溯源树
样例
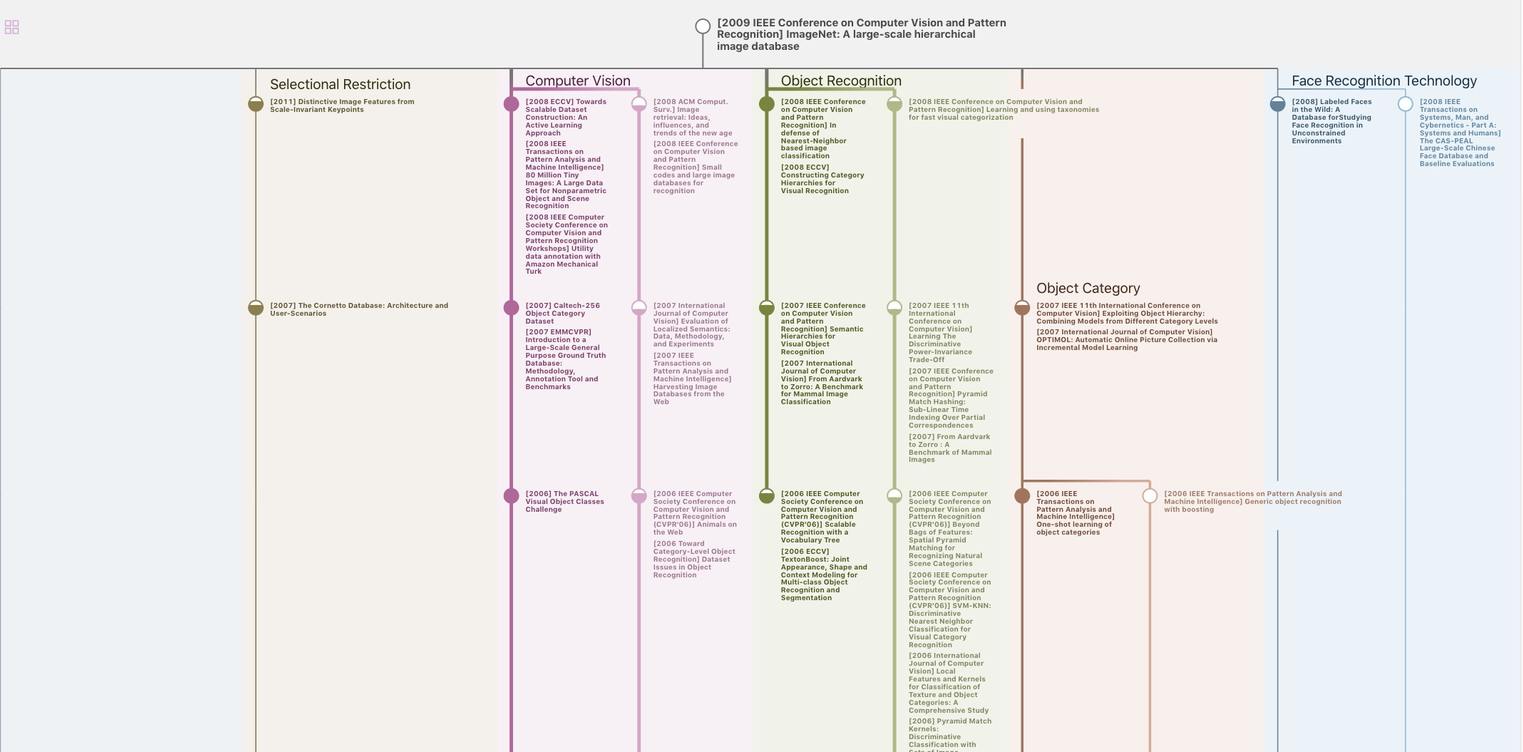
生成溯源树,研究论文发展脉络
Chat Paper
正在生成论文摘要