Ensemble Gene Selection Method Based on Multiple Tree Models
J. Inf. Process. Syst.(2023)
摘要
Identifying highly discriminating genes is a critical step in tumor recognition tasks based on microarray gene expression profile data and machine learning. Gene selection based on tree models has been the subject of several studies. However, these methods are based on a single-tree model, often not robust to ultra-highdimensional microarray datasets, resulting in the loss of useful information and unsatisfactory classification accuracy. Motivated by the limitations of single-tree-based gene selection, in this study, ensemble gene selection methods based on multiple-tree models were studied to improve the classification performance of tumor identification. Specifically, we selected the three most representative tree models: ID3, random forest, and gradient boosting decision tree. Each tree model selects top-n genes from the microarray dataset based on its intrinsic mechanism. Subsequently, three ensemble gene selection methods were investigated, namely multipletree model intersection, multiple-tree module union, and multiple-tree module cross-union, were investigated. Experimental results on five benchmark public microarray gene expression datasets proved that the multiple tree module union is significantly superior to gene selection based on a single tree model and other competitive gene selection methods in classification accuracy.
更多查看译文
关键词
Ensemble Tree Model,Gradient Boosting Decision Tree,Gene Selection,ID3,Random Forest
AI 理解论文
溯源树
样例
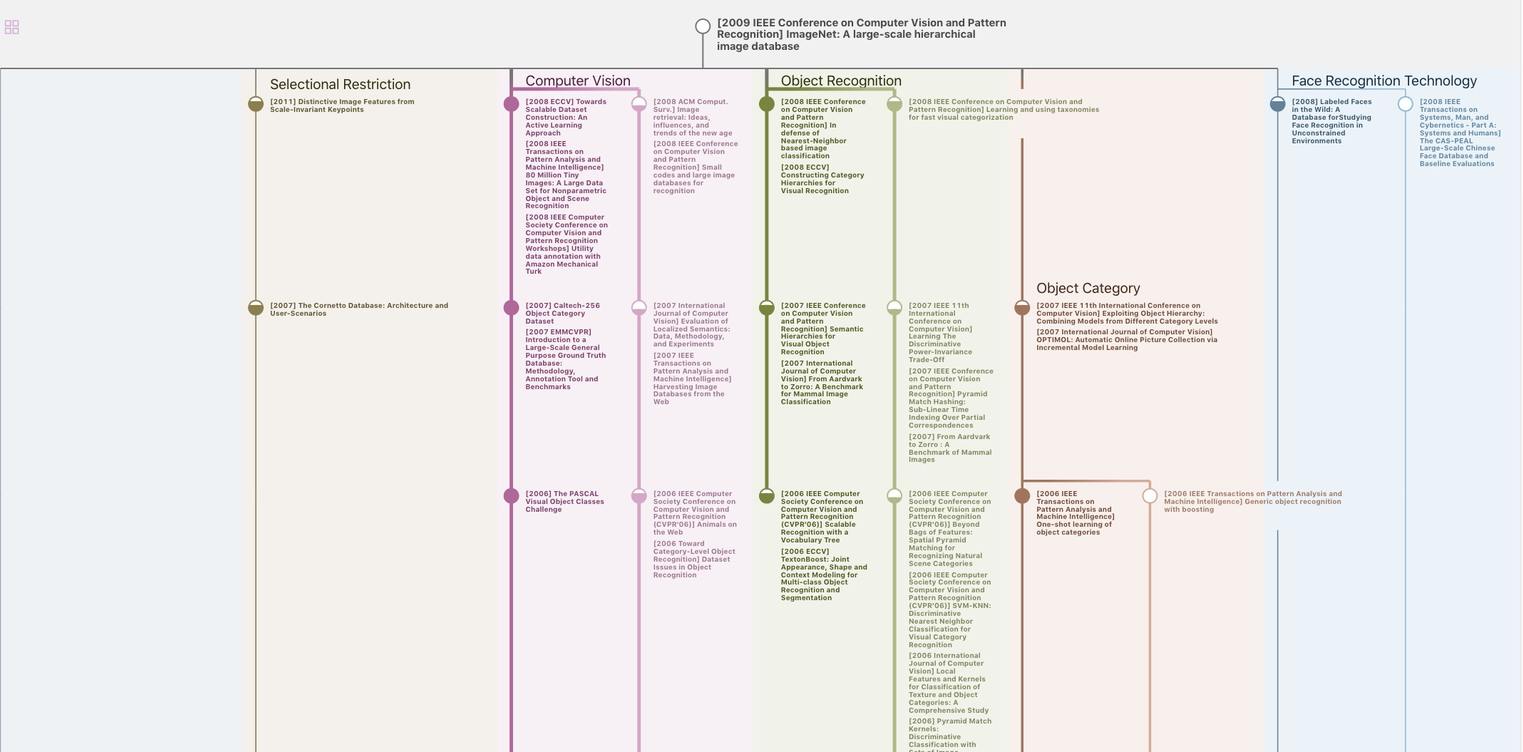
生成溯源树,研究论文发展脉络
Chat Paper
正在生成论文摘要