Bayesian gated-transformer model for risk-aware prediction of aero-engine remaining useful life
EXPERT SYSTEMS WITH APPLICATIONS(2024)
摘要
Remaining Useful Life (RUL) prediction plays a critical role in the prognostics and health management (PHM) for aero-engines. A variety of Deep Learning (DL) approaches have emerged for RUL prediction due to their flexibility of the architectures and superiority with nonlinear responses. The mainstream DL models usually focus on overall prediction accuracy, however, model reliability is actually the key impeding industrial applications. This paper proposes the Bayesian Gated-Transformer (BGT) model for reliable RUL prediction with quantified uncertainty. The BGT model is rooted in the transformer architecture and enhanced with the gated mechanism to balance between long-term trends and short-term patterns. Both the epistemic and aleatory uncertainties are quantified through the Bayesian setup of model weights and the introduced noise channel. The training of model weights is formulated with sampling-based variational inference which approximates the posterior of model uncertainty with Gaussian distributions. The BGT model has been applied to the NASA CMAPSS and N-CMAPSS datasets. Compared with alternative DL models, the BGT model demonstrates better or similar accuracy regarding overall prediction. The BGT model is capable of effective uncertainty quantification which enables risk-aware RUL prediction.
更多查看译文
关键词
Aero-engine prognosis,Remaining useful life prediction,Time-series forecasting,Bayesian deep learning,Uncertainty quantification
AI 理解论文
溯源树
样例
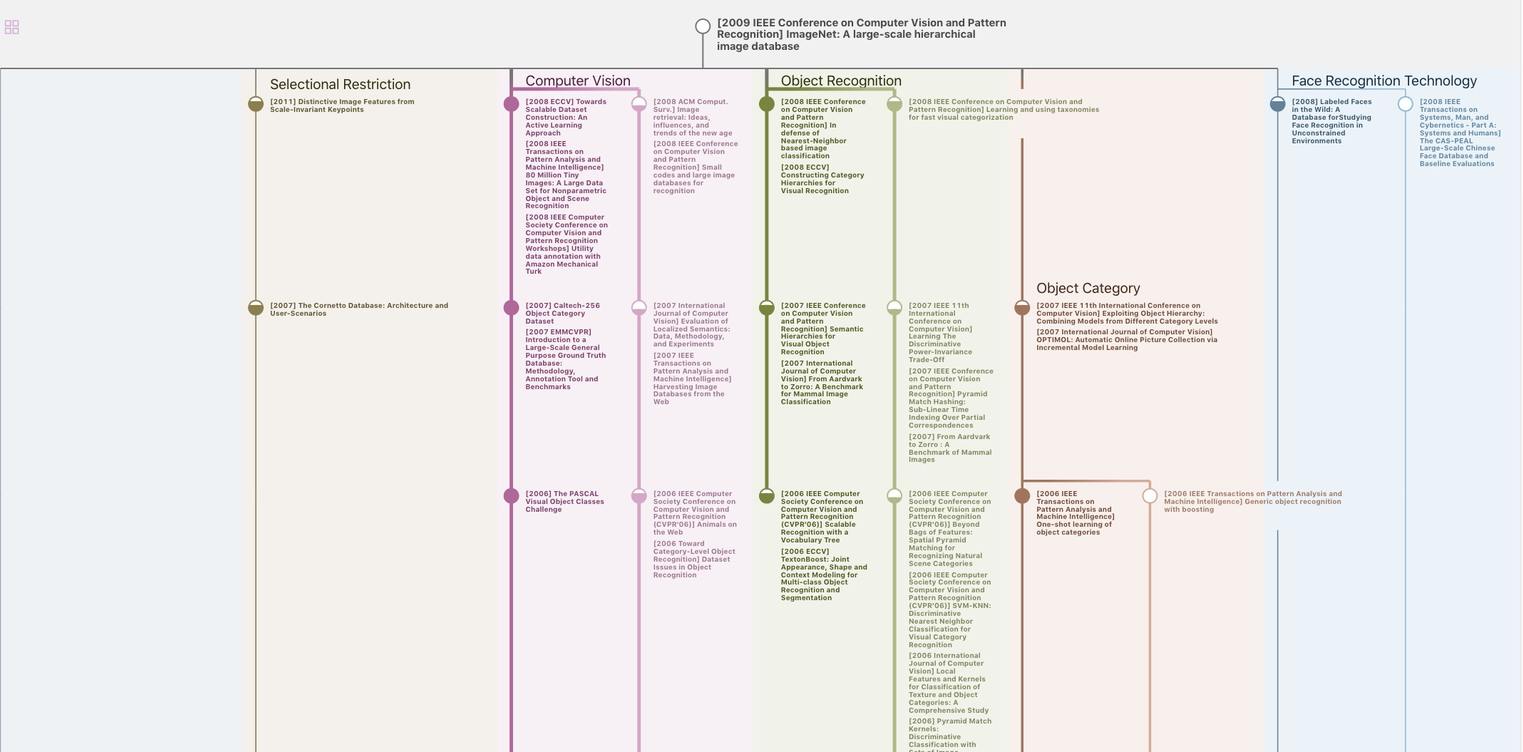
生成溯源树,研究论文发展脉络
Chat Paper
正在生成论文摘要