Contextual features online prediction for self-supervised graph representation
EXPERT SYSTEMS WITH APPLICATIONS(2024)
摘要
Self-supervised graph representation Learning (SSGRL) is an emerging technique for machine learning-based expert applications. SSGRL can effectively encode unlabeled data into machine-understandable knowledge embeddings that can be used in downstream expert tasks. Recently, Masked-Graph-Model (MGM) has achieved extraordinary performances in the field of SSGRL. However, MGM inevitably suffers from a so-called Negative Migration (NM) problem on unbalanced datasets for specific tasks such as graph classification. The NM problem forces the MGM to predict (reconstruct) the dominant nodes causing the ignoring of the minority but critical nodes. To this end, a novel online prediction module is proposed to dynamically predict the nodes with extended contexts that enable minority nodes can have more weights in the model. Based on the online prediction module, a graph representation learning model is proposed to alleviate the NM problem. Extensive experiments demonstrate that the model outperforms state-of-the-art methods on unbalanced datasets and achieves competitive performance on balanced datasets. Moreover, extra experiments demonstrate that the proposed method requires less computational resource overhead than existing methods. The code is available at: https://github.com/DuanhaoranCC/SimGOP.
更多查看译文
关键词
Self-supervised learning,Graph representation learning,Graph neural network
AI 理解论文
溯源树
样例
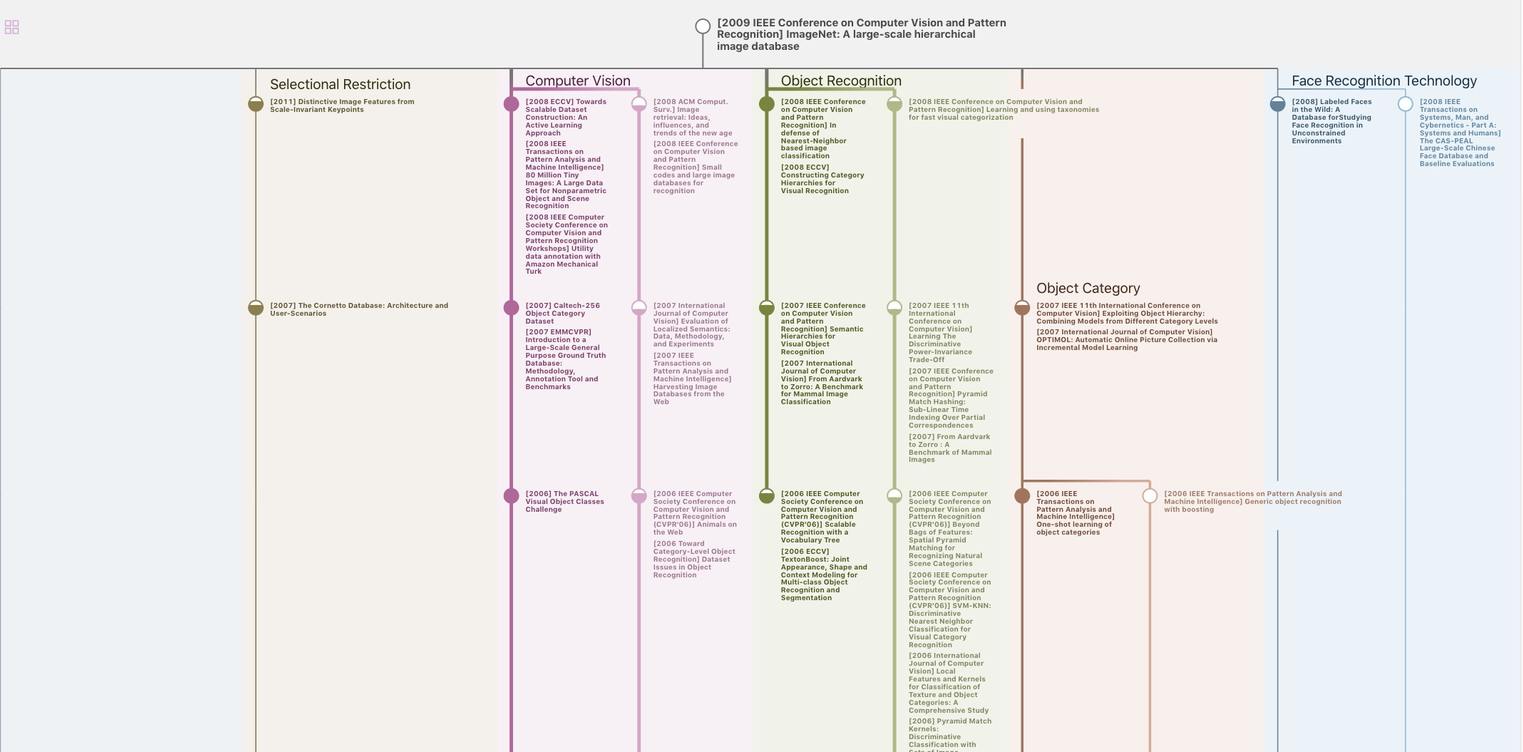
生成溯源树,研究论文发展脉络
Chat Paper
正在生成论文摘要