HyNCF: A hybrid normalization strategy via feature statistics for collaborative
EXPERT SYSTEMS WITH APPLICATIONS(2024)
摘要
Learning how to represent users based on historical interactions is a crucial problem for recommender systems. Unavoidable noise in interactions and long-tail items composed of a large number of unpopular items bring more challenges for learning better user representations and still limit the performance of existing models. Aiming to design a simple model that can alleviate both the noise problem and the long-tail item problem, we propose a Hybrid Normalization strategy via feature statistics for Collaborative Filtering (HyNCF). After each user is represented by his/her interacted items, the feature statistics of a target user are mixed with that of another randomly sampled user. In addition, the uncertainty estimation of the target user's feature statistics is calculated by a Gaussian sampling technique. Both kinds of improved feature statistics are separately used to normalize the target user's embedding, and then normalized embeddings are aggregated to generate two representations of the user. Based on the fusion of the two representations, the cosine contrastive loss is used to train HyNCF. The effectiveness of the proposed model is evaluated on five benchmark datasets.
更多查看译文
关键词
Recommender systems,Collaborative filtering,Normalization,Feature statistics
AI 理解论文
溯源树
样例
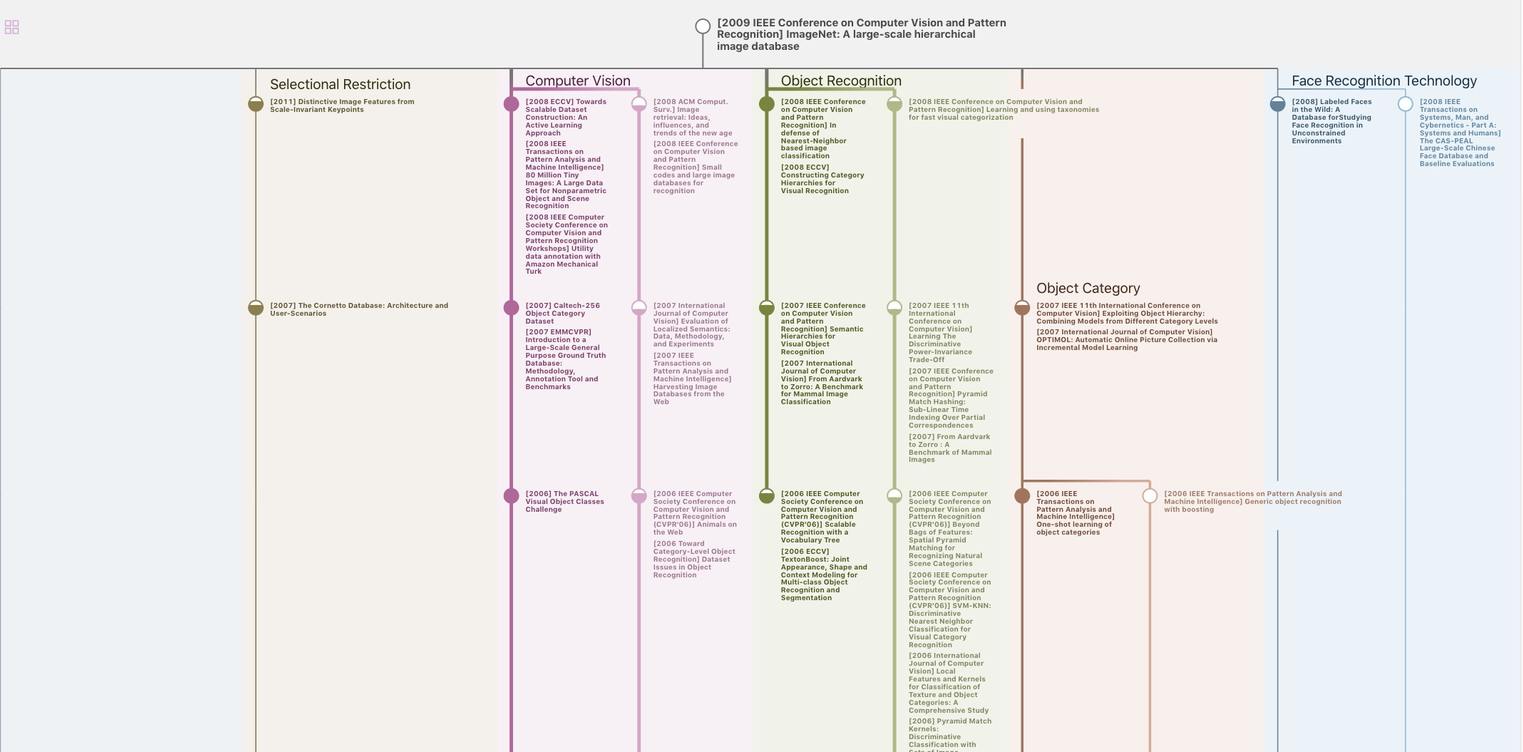
生成溯源树,研究论文发展脉络
Chat Paper
正在生成论文摘要