Interactive autoencoders with degradation constraint for hyperspectral super-resolution
IGARSS 2023 - 2023 IEEE INTERNATIONAL GEOSCIENCE AND REMOTE SENSING SYMPOSIUM(2023)
摘要
Owing to the strong ability of feature extraction and representation, deep learning has exhibited powerful potential in the field of multispectral-aided hyperspectral super-resolution (MS-aided HS-SR). Though great strides have been made by existing methods, their superior performance mainly drives from large training datasets, hence failing to handle the real cases with limited samples. In this article, we propose an unsupervised method inspired by the theory of spectral mixing, which is based solely on one pair of HS-MS correspondence. Specifically, two coupled autoencoders are employed as the backbone of our network, aiming at deriving the latent abundance representations and corresponding endmembers of input HS-MS data. To enrich the feature representations and guide the network learning, we embed an interactive module into the encoder part to enhance the information transmission and design a degradation loss to constrain the target image. Experiments in Chikusei dataset demonstrate the effectiveness of our proposed method.
更多查看译文
关键词
Deep learning,hyperspectral,multispectral,super-resolution
AI 理解论文
溯源树
样例
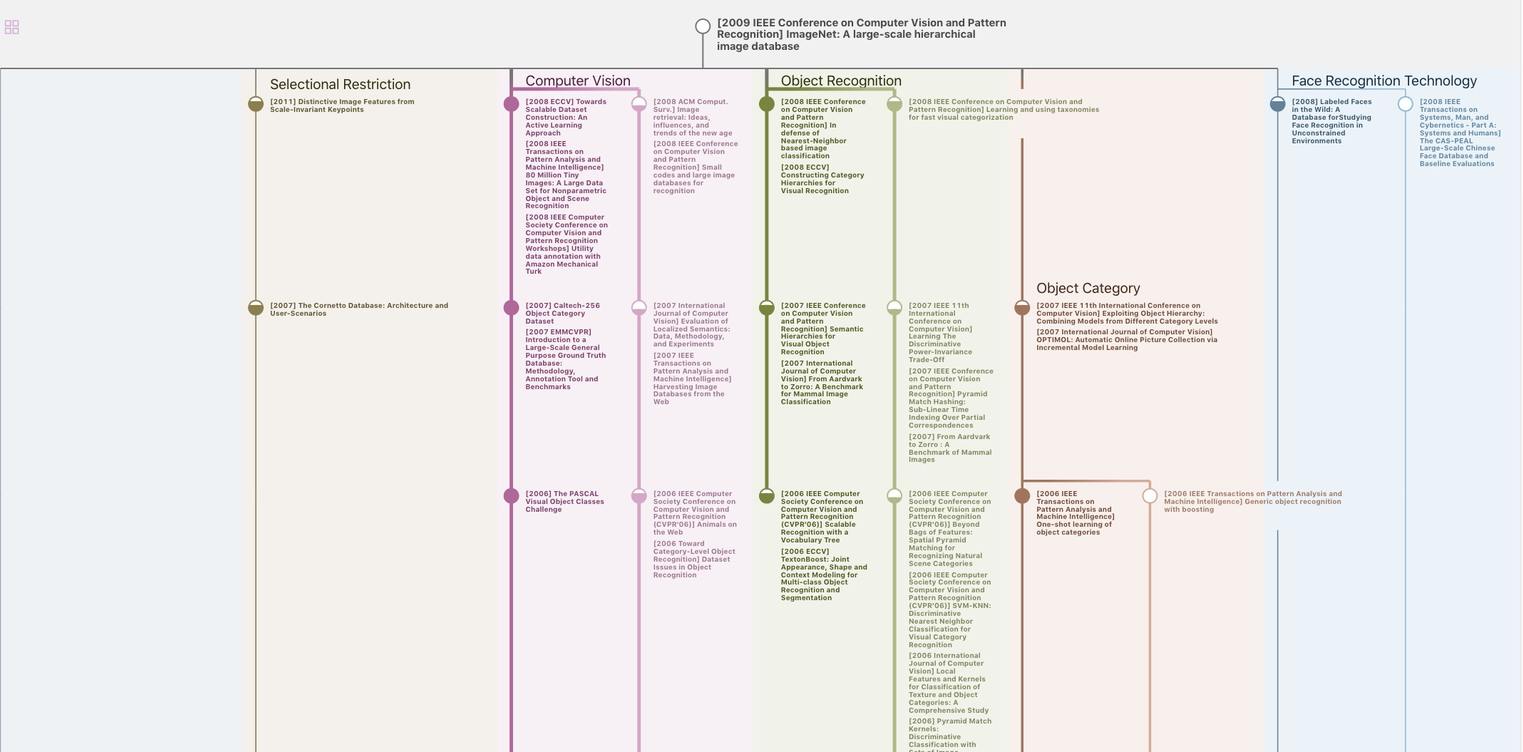
生成溯源树,研究论文发展脉络
Chat Paper
正在生成论文摘要