Conspiracy Spoofing Orders Detection with Transformer-Based Deep Graph Learning.
ADMA (2)(2023)
摘要
Spoofing behaviors profits from other participants via placing large amounts of deceptive orders to create misleading signals with the help of advanced electronic trading techniques, severely harming the healthy and sustainable development of modern financial markets. Existing works focus mostly on detecting individual spoofing issuers or orders, which ignore the capability of mining hidden conspiracy fraud patterns. Consequently, they face significant challenges in tracking organized spoofing behaviors. To this end, in this paper, we proposed a novel transformer-based graph neural network to learn the temporal inter-connected transaction representations for conspiracy spoofing behavior detection. In particular, we model user orders in the financial market by the temporal transaction graph layer first, which produces temporal and relational encodings of the user’s order behaviors. Then, we devise a transformer-based neural network to automatically learn the deeper sequential and inter-connected representations, which could perceive a boarder view of the user’s transaction patterns. Finally, the temporal and relational features are jointly optimized by the detection network in an end-to-end manner. We conduct extensive experiments on a real-world dataset from one of the largest financial exchange markets in East Asia. The result strongly proves the superior performance of our proposed method in fighting against conspiracy spoofing behaviors with higher accuracy without handcraft feature engineering, compared with ten state-of-the-art baselines. To the best of our knowledge, this is the first work that proposed a transformer-based deep graph learning approach for spoofing detection challenges and demonstrates its practical ability in real-world applications.
更多查看译文
关键词
graph,transformer-based
AI 理解论文
溯源树
样例
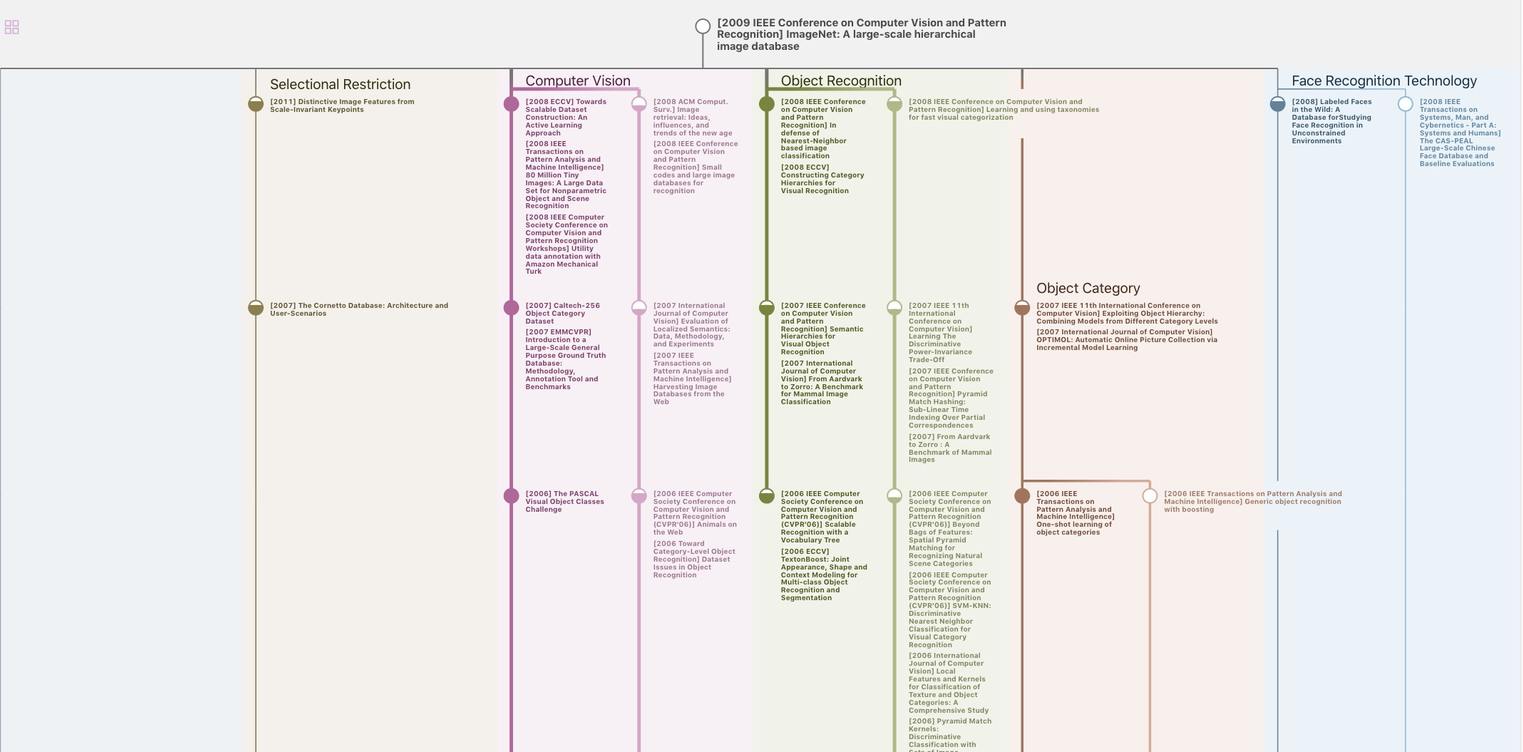
生成溯源树,研究论文发展脉络
Chat Paper
正在生成论文摘要