Identifying Critical Congested Roads Based on Traffic Flow-Aware Road Network Embedding.
ADMA (1)(2023)
摘要
Traffic congestion occurs frequently and concurrently on urban road networks, and may cause widespread traffic paralysis if not controlled promptly. To relieve traffic congestion and avoid traffic paralysis, it is significant to identify critical congested roads with great propagation influence on others. Existing studies mainly focus on topological measures and statistical approaches to evaluate the criticality of road segments. However, critical congested roads are generated by dynamic changes in traffic flow, so that identifying them involves both the network structure and dynamic traffic flows is required. In this paper, we propose a novel road network embedding model, called Seg2Vec, to learn comprehensive features of road segments considering both the road structural information and traffic flow distribution. The Seg2Vec model combines a Markov Chain-based random walk with the Skip-gram model. The random walk is conducted on the road network based on the transition probabilities computed from historical trajectory data. Moreover, we define the propagation influence of a congested road by a score function based on the learned road representation. The goal is to find the critical congested roads with top-K propagation influences. Evaluation experiments are conducted to verify the effectiveness and efficiency of the proposed method. A case study of identifying critical congested roads from a congestion cluster is also demonstrated. The identified critical congested roads can facilitate decision-making for traffic management.
更多查看译文
关键词
critical congested roads,traffic,flow-aware
AI 理解论文
溯源树
样例
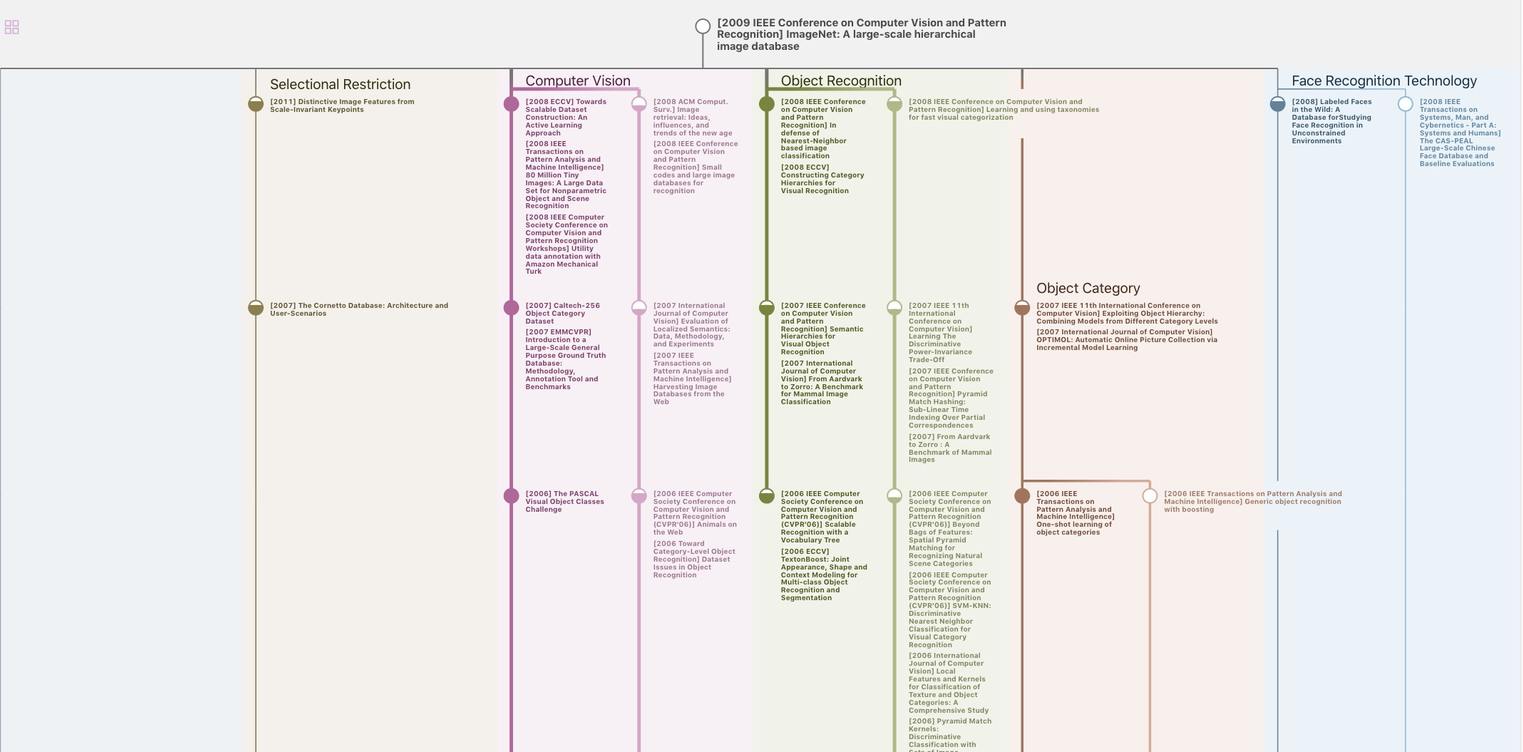
生成溯源树,研究论文发展脉络
Chat Paper
正在生成论文摘要