Personalized Stress Detection System Using Physiological Data from Wearable Sensors.
FDSE(2023)
摘要
Thanks to advancements in wearable technologies and biosensors, the accurate collection of physiological data is now more available and convenient than ever before. Thus, it has become more practical to use artificial intelligence technology to analyze physiological data collected from wearable elements. This could be beneficial to a large number of patients suffering from psychiatric disorders who experience high levels of pressure and stress in the modern era, as it allows for timely identification and intervention. Federated learning is a state-of-the-art machine learning paradigm that helps solve the impracticality of collecting personal physiological data, in a privacy respecting and distributed manner, for implementing artificial intelligence-powered healthcare applications. However, healthcare solutions based on federated learning still face accuracy problems as while physiological data varies from person to person, the averaging strategy of general federated learning algorithms cannot converge into an effective one-for-all solution model. To address this issue, we propose a stress detection system that integrates personalized federated learning algorithms. This system tailors the stress detection mechanism to each individual’s physiological data, thus improving the accuracy and overall performance of the entire system. To evaluate our proposed system, we conducted extensive simulations using a physiological dataset of drivers who were stressed while driving on crowded streets. The experiment results demonstrated that our proposed system improves stress detection accuracy by more than 5% compared to a typical federated learning system.
更多查看译文
AI 理解论文
溯源树
样例
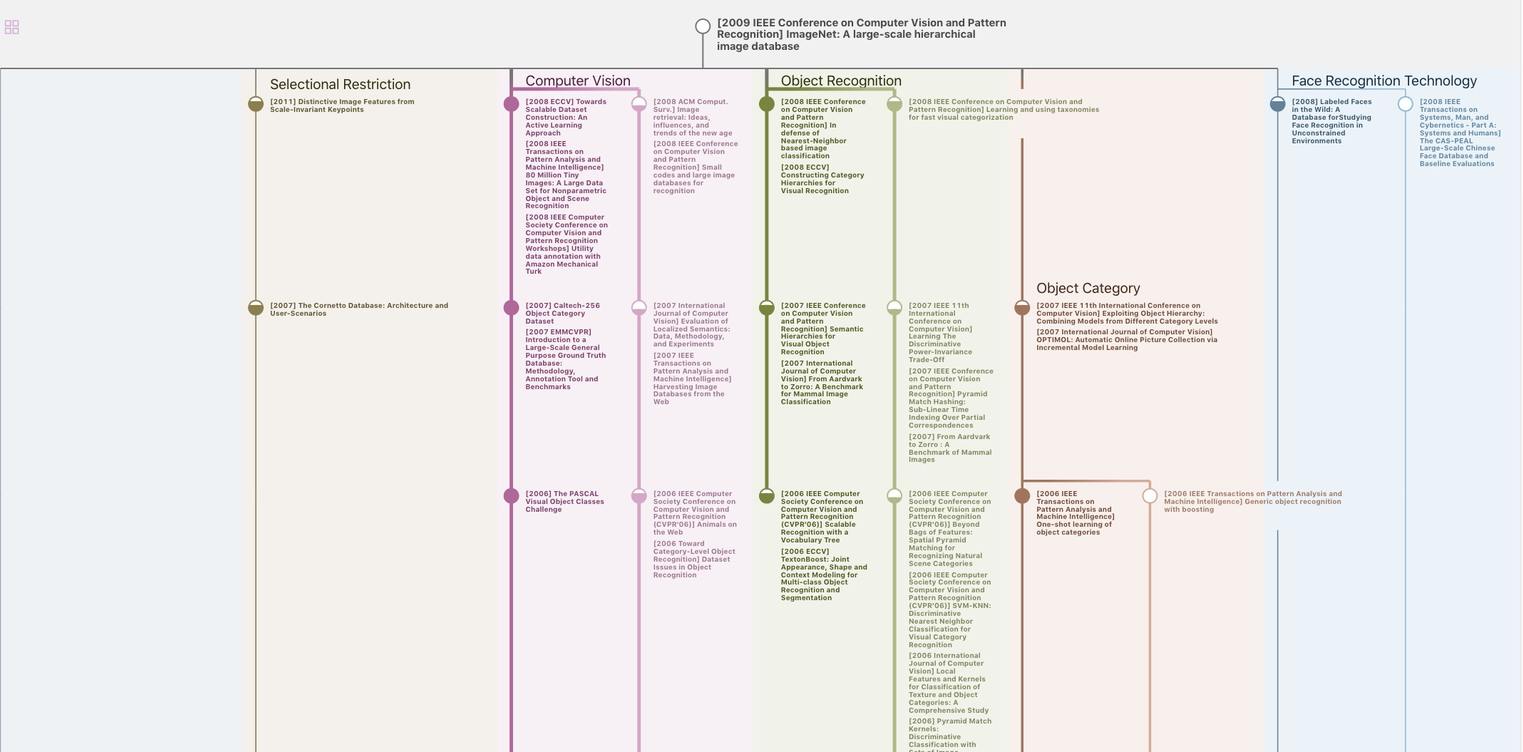
生成溯源树,研究论文发展脉络
Chat Paper
正在生成论文摘要