Universal Detection and Source Attribution of Diffusion Model Generated Images with High Generalization and Robustness
PATTERN RECOGNITION AND MACHINE INTELLIGENCE, PREMI 2023(2023)
摘要
The proliferation of synthetic media over the internet is posing a significant social threat. Recent advancements in Diffusion Models (DM) have made it easier to create astonishingly photo-realistic synthetic media with high stability and control. Moreover, applications like DALLE-2, powered by DM and Large Language Models (LLM), permit visual content generation from natural language description, enabling opportunities for everyone to generate visual media. Hence, there is an immediate need to identify synthetic images and attribute them to their source architectures. In this work, we propose a synthetic image detector as universal detector and a source model attributor based on a popular transfer-learning model ResNet-50 and compare the results with other popular models, including Visual Geometry Group (VGG) 16, XceptionNet and InceptionNet. The proposed universal detector attains over 96% accuracy, with a source attribution, accuracy over 93% for detection of Diffusion Model generated images. The model also succeeds in achieving significant generalization and robustness capabilities under different training-testing configurations, as proven by our experiments.
更多查看译文
关键词
Diffusion Model,Generative Adversarial Network,Source Attribution,Synthetic Media,Transfer Learning,Universal Detection
AI 理解论文
溯源树
样例
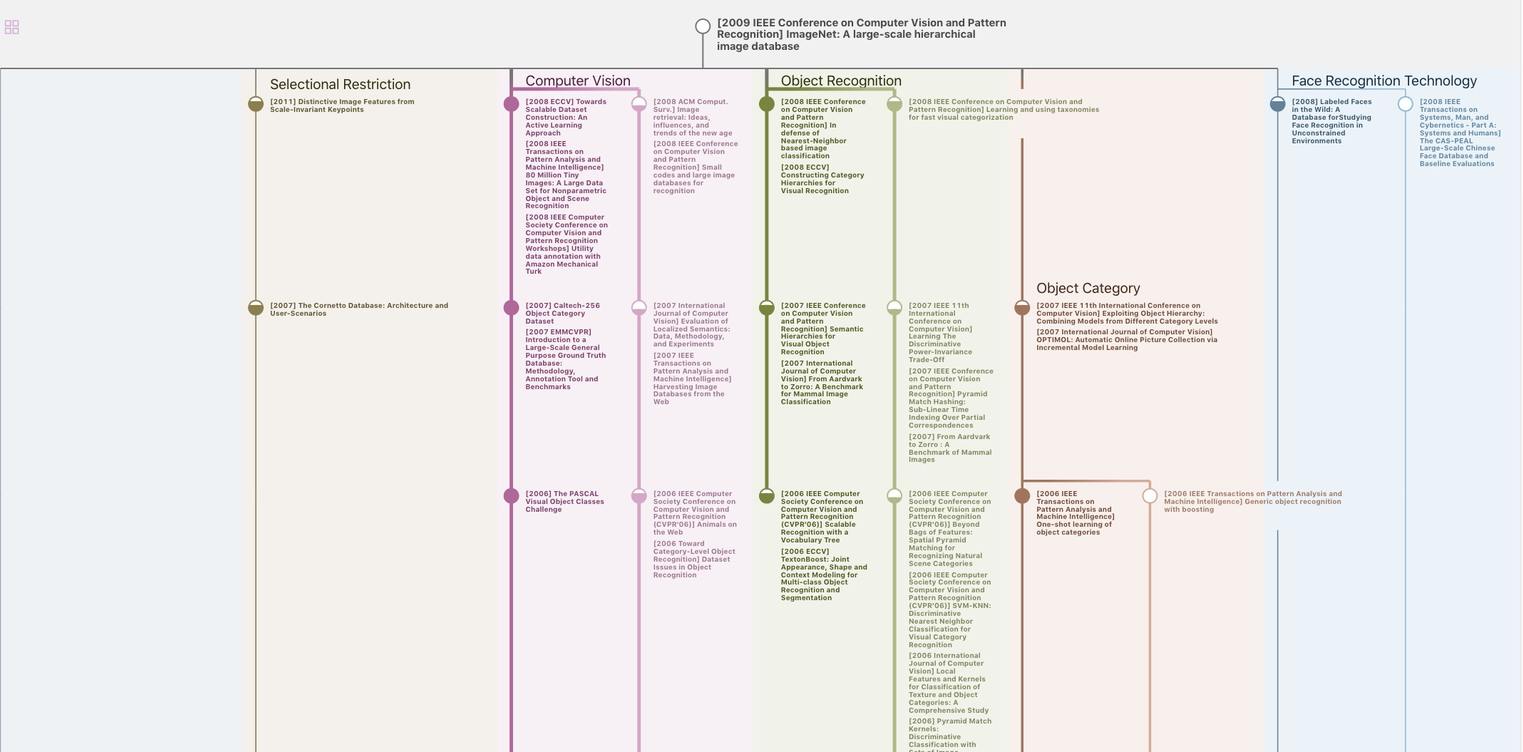
生成溯源树,研究论文发展脉络
Chat Paper
正在生成论文摘要