Introducing Semantic-Based Receptive Field into Semantic Segmentation via Graph Neural Networks
NEURAL INFORMATION PROCESSING, ICONIP 2023, PT VI(2024)
摘要
Current semantic segmentation models typically use deep learning models as encoders. However, these models have a fixed receptive field, which can cause mixed information within the receptive field and lead to confounding effects during neural network training. To address these limitations, we propose the "semantic-based receptive field" based on our analysis in current models. This approach seeks to improve the segmentation performance by aggregate image patches with similar representation rather than their physical location, aiming to enhance the interpretability and accuracy of semantic segmentation models. For implementation, we utilize Graph representation learning (GRL) approaches into current semantic segmentation models. Specifically, we divide the input image into patches and construct them into graph-structured data that expresses semantic similarity. Our Graph Convolution Receptor block uses graph-structured data purpose-built from image data and adopt a node-classification-like perspective to address the problem of semantic segmentation. Our GCR module models the relationship between semantic relative patches, allowing us to mitigate the adverse effects of confounding information and improve the quality of feature representation. By adopting this approach, we aim to enhance the accuracy and robustness of the semantic segmentation task. Finally, we evaluated our proposed module on multiple semantic segmentation models and compared its performance to baseline models on multiple semantic segmentation datasets. Our empirical evaluations demonstrate the effectiveness and robustness of our proposed module, as it consistently outperformed baseline models on these datasets.
更多查看译文
关键词
Semantic Segmentation,Computer Vision,Graph representation learning
AI 理解论文
溯源树
样例
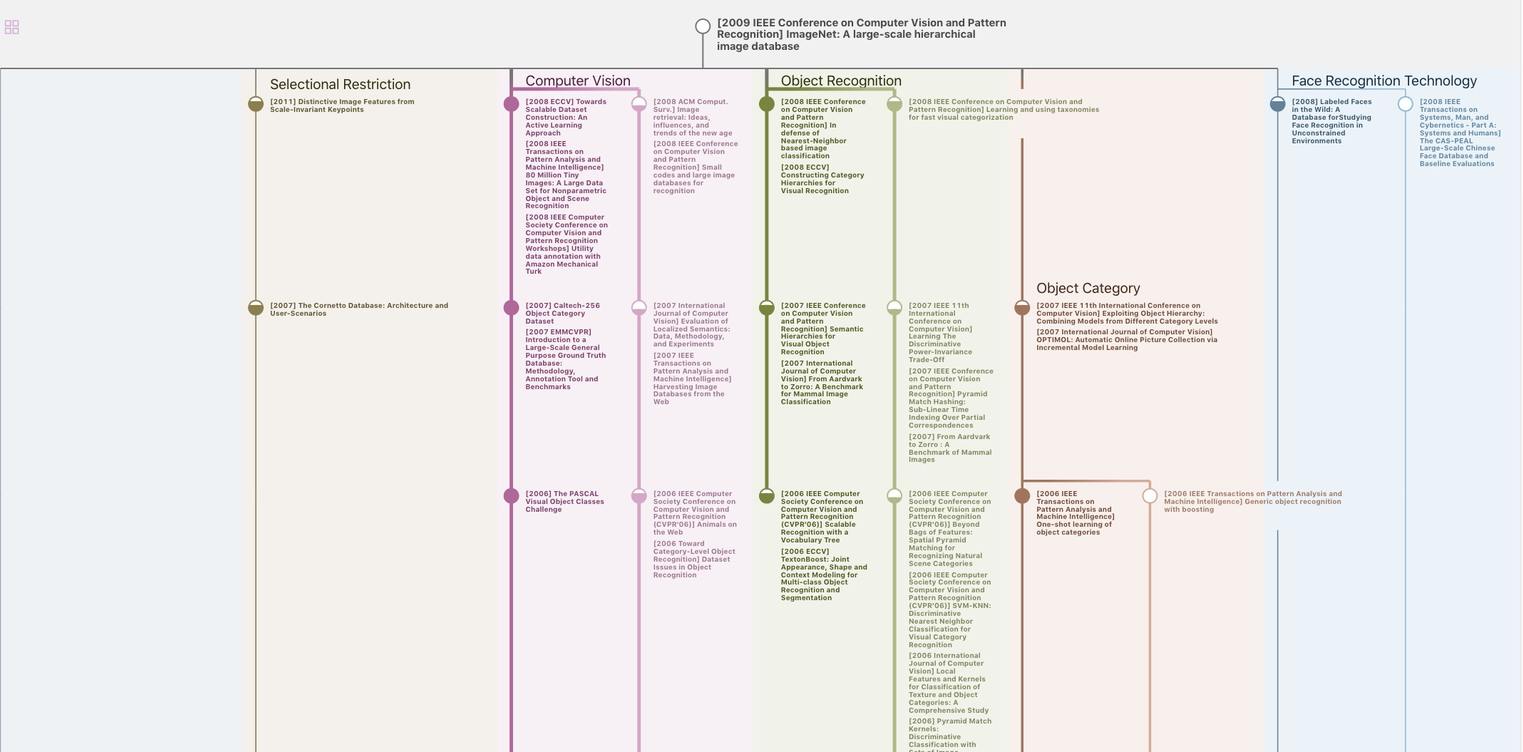
生成溯源树,研究论文发展脉络
Chat Paper
正在生成论文摘要