How Legal Knowledge Graph Can Help Predict Charges for Legal Text
NEURAL INFORMATION PROCESSING, ICONIP 2023, PT VI(2024)
摘要
The existing methods for predicting Easily Confused Charges (ECC) primarily rely on factual descriptions from legal cases. However, these approaches overlook some key information hidden in these descriptions, resulting in an inability to accurately differentiate between ECC. Legal domain knowledge graphs can showcase personal information and criminal processes in cases, but they primarily focus on entities in cases of insolation while ignoring the logical relationships between these entities. Different relationships often lead to distinct charges. To address these problems, this paper proposes a charge prediction model that integrates a Criminal Behavior Knowledge Graph (CBKG), called Charge Prediction Knowledge Graph (CP-KG). Firstly, we defined a diverse range of legal entities and relationships based on the characteristics of ECC. We conducted fine-grained annotation on key elements and logical relationships in the factual descriptions. Subsequently, wematched the descriptions with theCBKGto extract the key elements, which were then encoded by Text Convolutional Neural Network (TextCNN). Additionally, we extracted case subgraphs containing sequential behaviors from the CBKG based on the factual descriptions and encoded them using a Graph Attention Network (GAT). Finally, we concatenated these representations of key elements, case subgraphs, and factual descriptions, collectively used for predicting the charges of the defendant. To evaluate the CP-KG, we conducted experiments on two charge prediction datasets consisting of real legal cases. The experimental results demonstrate that the CP-KG achieves scores of 99.10% and 90.23% in the Macro-F1 respectively. Compared to the baseline methods, the CP-KG shows significant improvements with 25.79% and 13.82% respectively.
更多查看译文
关键词
Charge prediction,Easily confused charges,Knowledge graph,Graph attention network
AI 理解论文
溯源树
样例
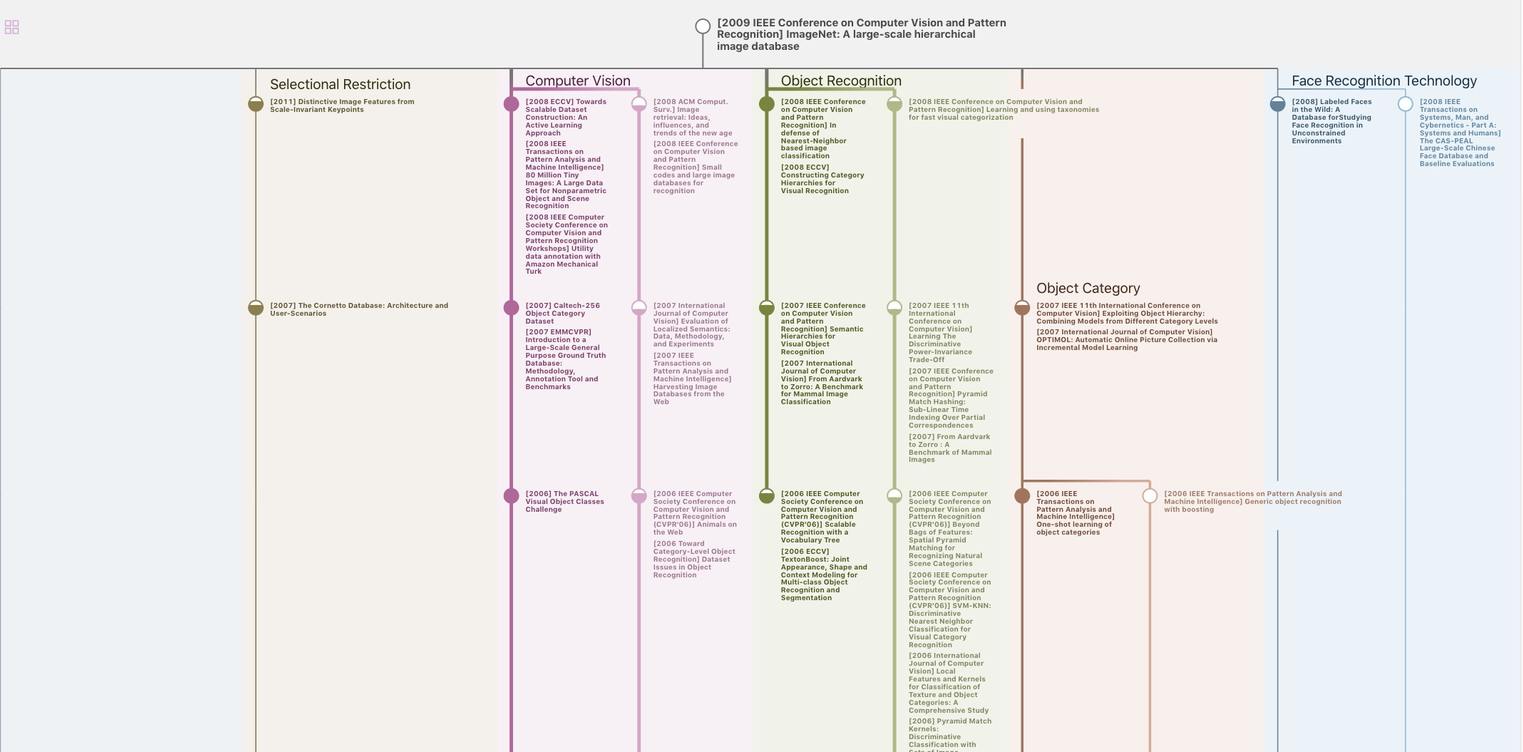
生成溯源树,研究论文发展脉络
Chat Paper
正在生成论文摘要