MOC: Multi-modal Sentiment Analysis via Optimal Transport and Contrastive Interactions
NEURAL INFORMATION PROCESSING, ICONIP 2023, PT II(2024)
Abstract
Multi-modal sentiment analysis (MSA) aims to utilize information from various modalities to improve the classification of emotions. Most existing studies employ attention mechanisms for modality fusion, overlooking the heterogeneity of different modalities. To address this issue, we propose an approach that leverages optimal transport for modality alignment and fusion, specifically focusing on distributional alignment. However, solely relying on the optimal transport module may result in a deficiency of intra-modal and inter-sample interactions. To tackle this deficiency, we introduce a double-modal contrastive learning module. Specifically, we propose a model MOC (Multi-modal sentiment analysis via Optimal transport and Contrastive interactions), which integrates optimal transport and contrastive learning. Through empirical comparisons on three established multi-modal sentiment analysis datasets, we demonstrate that our approach achieves state-of-the-art performance. Additionally, we conduct extended ablation studies to validate the effectiveness of each proposed module.
MoreTranslated text
Key words
Multi-modal sentiment analysis,Optimal transport,Contrastive learning
AI Read Science
Must-Reading Tree
Example
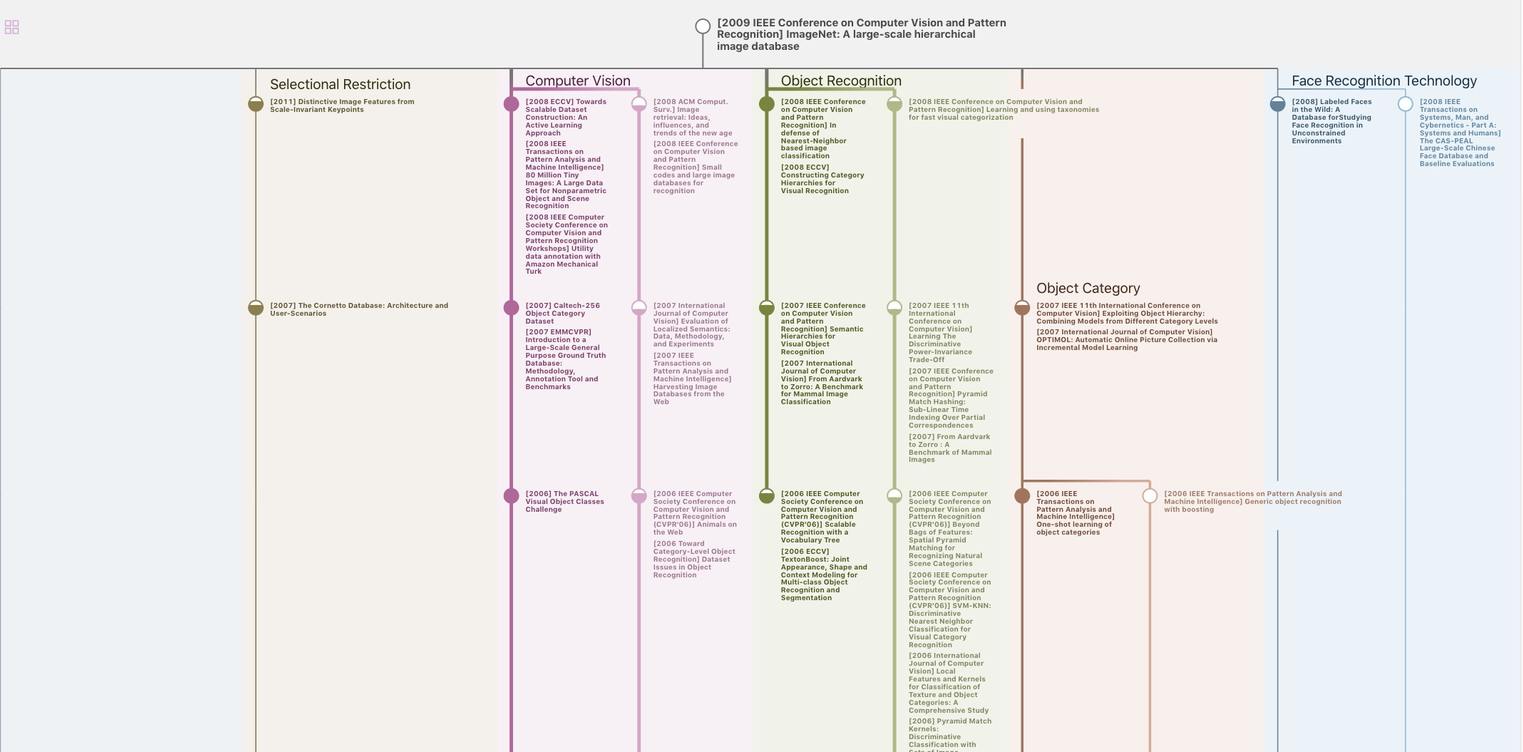
Generate MRT to find the research sequence of this paper
Chat Paper
Summary is being generated by the instructions you defined