Time Series Anomaly Detection with a Transformer Residual Autoencoder-Decoder
NEURAL INFORMATION PROCESSING, ICONIP 2023, PT IV(2024)
摘要
Time series anomaly detection is of great importance in a variety of domains such as finance fraud, industrial production, and information systems. However, due to the complexity and multiple periodicity of time series, extracting global and local information from different perspectives remains a challenge. In this paper, we propose a novel Transformer Residual Autoencoder-Decoder Model called TRAD for time series anomaly detection, which is based on a multi-interval sampling strategy incorporating with residual learning and stacked autoencoder-decoder to promote the ability to learn global and local information. Prediction error is applied to calculate anomaly scores using the proposed model from different scales, and the aggregated anomaly scores are utilized to infer outliers of the time series. Extensive experiments are conducted on five datasets and the results demonstrate that the proposed model outperforms the previous state-of-the-art baselines.
更多查看译文
关键词
Time Series Analysis,Anomaly Detection,Transformer Autoencoder-Decoder
AI 理解论文
溯源树
样例
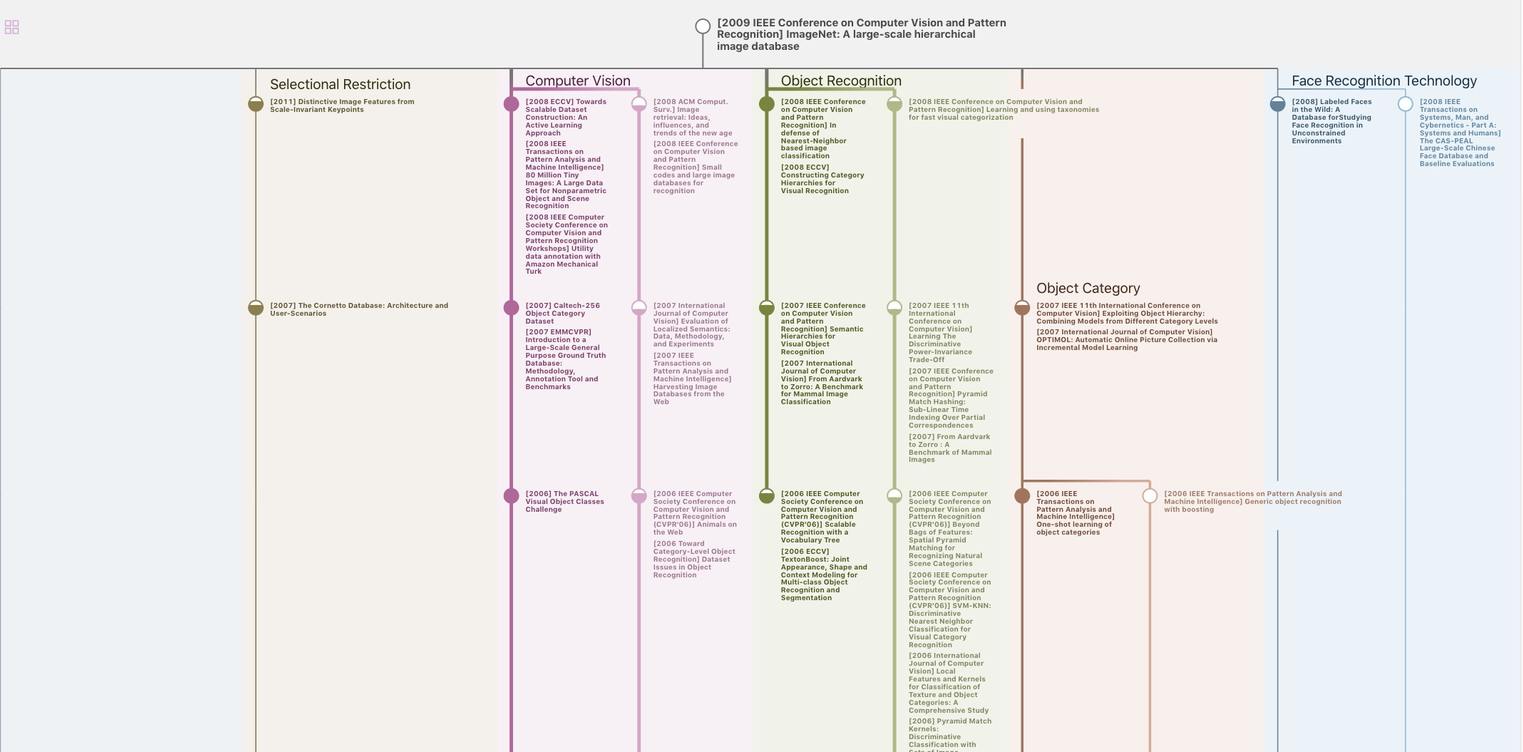
生成溯源树,研究论文发展脉络
Chat Paper
正在生成论文摘要