Interactive Selection Recommendation Based on the Multi-head Attention Graph Neural Network
NEURAL INFORMATION PROCESSING, ICONIP 2023, PT III(2024)
摘要
The click-through rate prediction of users is a critical task in the recommendation system. As a powerful machine learning method, graph neural networks have been favored by scholars to solve the task recently. However, most graph neural network-based click-through rate prediction models ignore the effectiveness of feature interaction and generally model all feature combinations, even if some are meaningless. Therefore, this paper proposes a Multi-head attention Graph Neural Network with Interactive Selection, named MGNN IS in short, to capture the complex feature interactions via graph structures. In particular, there are three sub-graphs to be constructed to capture internal information of users and items respectively, and interactive information between users and items, namely the user internal graph, item internal graph, and user-item interaction graph correspondingly. Moreover, the proposed model designs a multi-head attention propagation module for the aggregation with an interactive selection strategy. This module can select the constructed graph and increase diversity with multiple heads to achieve the high-order interaction from the multiple layers. Finally, the proposed model fuses the features, and predicts. Experiments on three public datasets demonstrate that the proposed model outperformed other advanced models.
更多查看译文
关键词
Click-through Rate,Feature Interaction,Internal Graph,Interaction Graph,Multi-head Attention
AI 理解论文
溯源树
样例
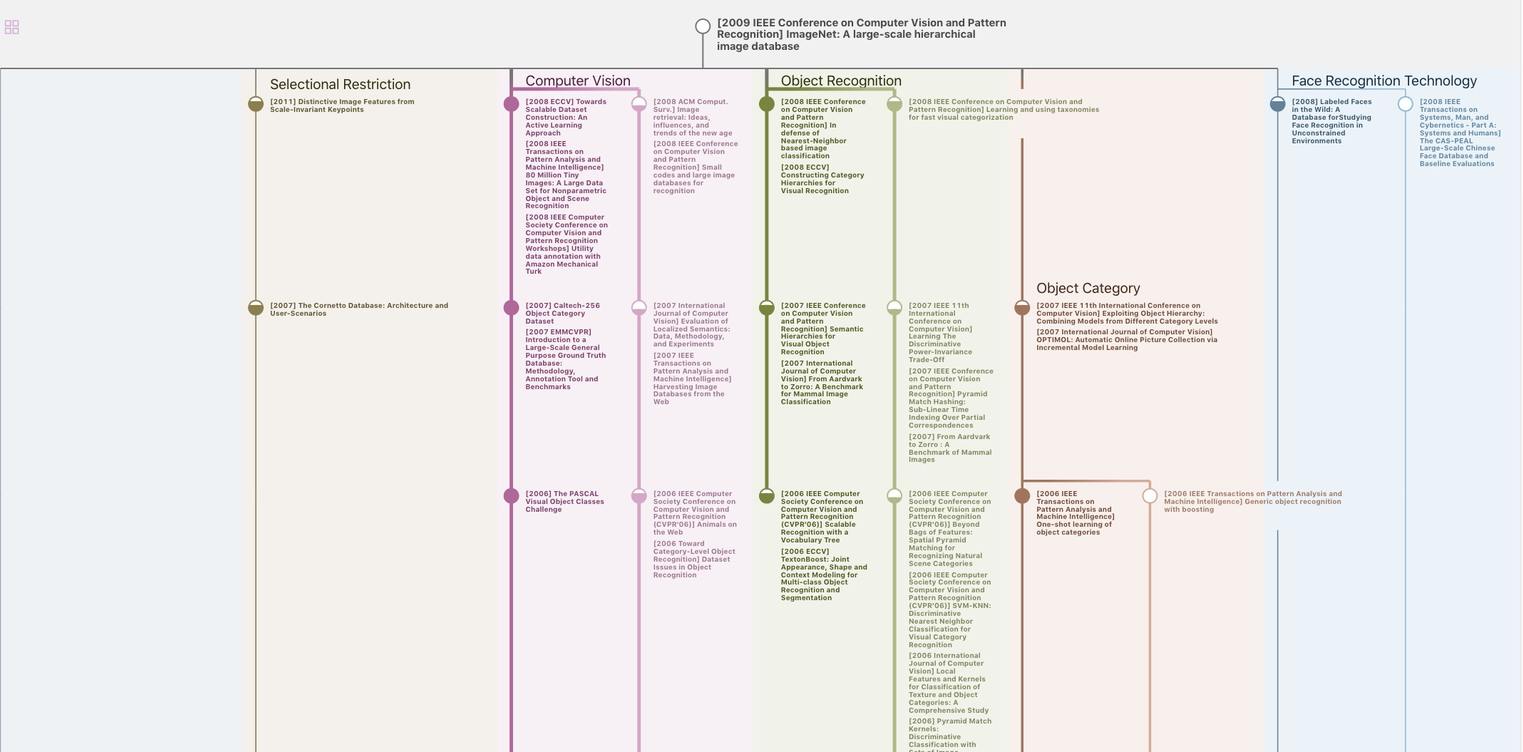
生成溯源树,研究论文发展脉络
Chat Paper
正在生成论文摘要